- These Sony headphones deliver premium sound and comfort - without the premium price
- The LG soundbar I prefer for my home theater slaps with immersive audio - and it's not the newest model
- Samsung's new flagship laptop rivals the MacBook Pro, and it's not just because of the display
- Email marketing is back and big social is panicking - everything you need to know
- Revisiting Docker Hub Policies: Prioritizing Developer Experience | Docker
4 essential lessons in AI governance

Austin-based software company Planview began using generative AI to boost productivity around 18 months ago. During that same period, they started integrating gen AI into their products, building a copilot that users interact with to do strategic portfolio management and value stream management. The copilot creates plan scenarios to help managers hit product release goals, and suggests ways to move deliverables around on roadmaps, share work between teams, and reallocate investment.
As early adopters, Planview realized early on that if they really wanted to lean into AI, they’d need to set up policies and governance to cover both what they do in house, and what they do to enhance their product offering. Based on the company’s experience, and that of other CIOs elsewhere, four lessons can be distilled to help organizations develop their own approach to AI governance.
Piggyback on an existing framework
AI governance is not much different from any other governance. In fact, according to Planview CTO Mik Kersten, because most AI policy is about data, it should be easy to leverage existing frameworks. Planview took the guidelines they were already using for open-source and cloud, and adapted them to what they needed for AI governance.
Mik Kersten, CTO, Planview
Planview
A very different organization, Florida State University (FSU), grew AI governance out of the existing IT governance council, which meets on a regular basis to prioritize investments and risk. “We rank investments, both financially and in terms of value and impact across the campus,” says Jonathan Fozard, the university’s CIO. “AI projects became a part of that discussion and that’s how we built our AI governance.”
FSU’s use cases range from scientific research to office productivity — and they teach AI as part of curricula from engineering and law to all other majors where students are likely to use AI when they join the workforce. Fozard says the act of balancing cost and risk with the potential value add is like a teeter-totter approach. During the first round of discussions, certain projects rise to the top. Then the council starts looking at those high priority projects to make sure they can protect everything the university needs to protect, including intellectual property, research aspirations, user privacy, and sensitive data.
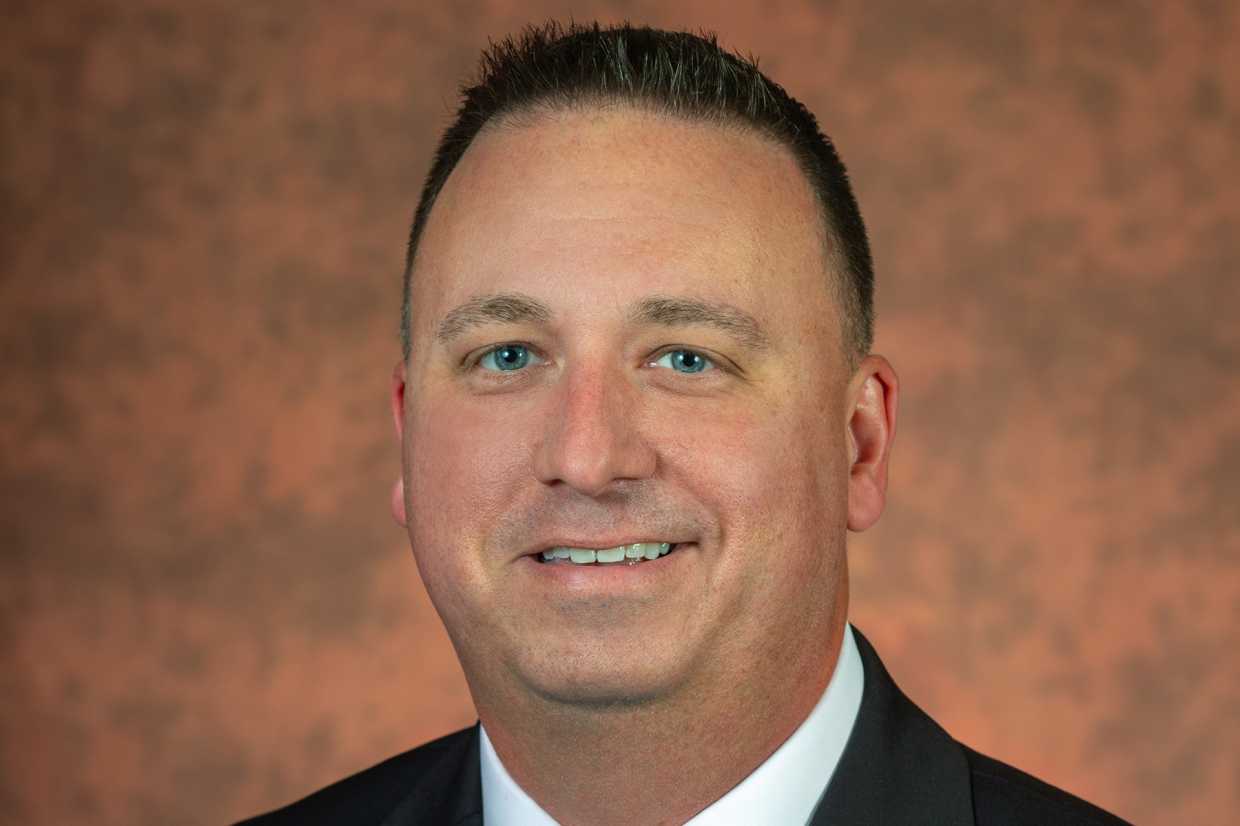
Jonathan Fozard, CIO, FSU
Florida State University
“Whether you’re in higher education or a corporate environment, focus on production first,” says Fozard. “Get beyond the flashiness and think about what you’re trying to achieve. Find out how you can use the technology to enable innovation at all levels of the organization. Then make sure you protect your data and your people.”
Be clear on what’s done in-house and where you partner
“We have to make a very clear policy on what we build versus what we buy,” says Kersten. “I have fairly large AI and data science teams, and our customer success organization wanted those teams to build customer support capabilities. But we need those experts to develop product features. To keep them on the core work, our policy makes it clear what we build and what we buy.”
Kersten thinks it’s also important to make clear policy on how open-source is used. Planview chose to integrate open-source models only for research and internal use cases. As for features they sell, the company builds on LLMs that have clear terms of use. “Part of our policy is to make sure the terms of use of the large language model provider meet our privacy and compliance needs,” he says.
In a completely different industry, Wall Street English, the Hong Kong-based international English language academy, developed its own AI stack to achieve mastery of a technology they consider core to their business. “We strive for faster innovation, better results and a range of customized solutions that perfectly match student and teacher needs,” says Roberto Hortal, the company’s chief product and technology officer. “We maintain a proactive approach. Part of our policy is to be on top of the latest developments, best practices, and potential risks.”
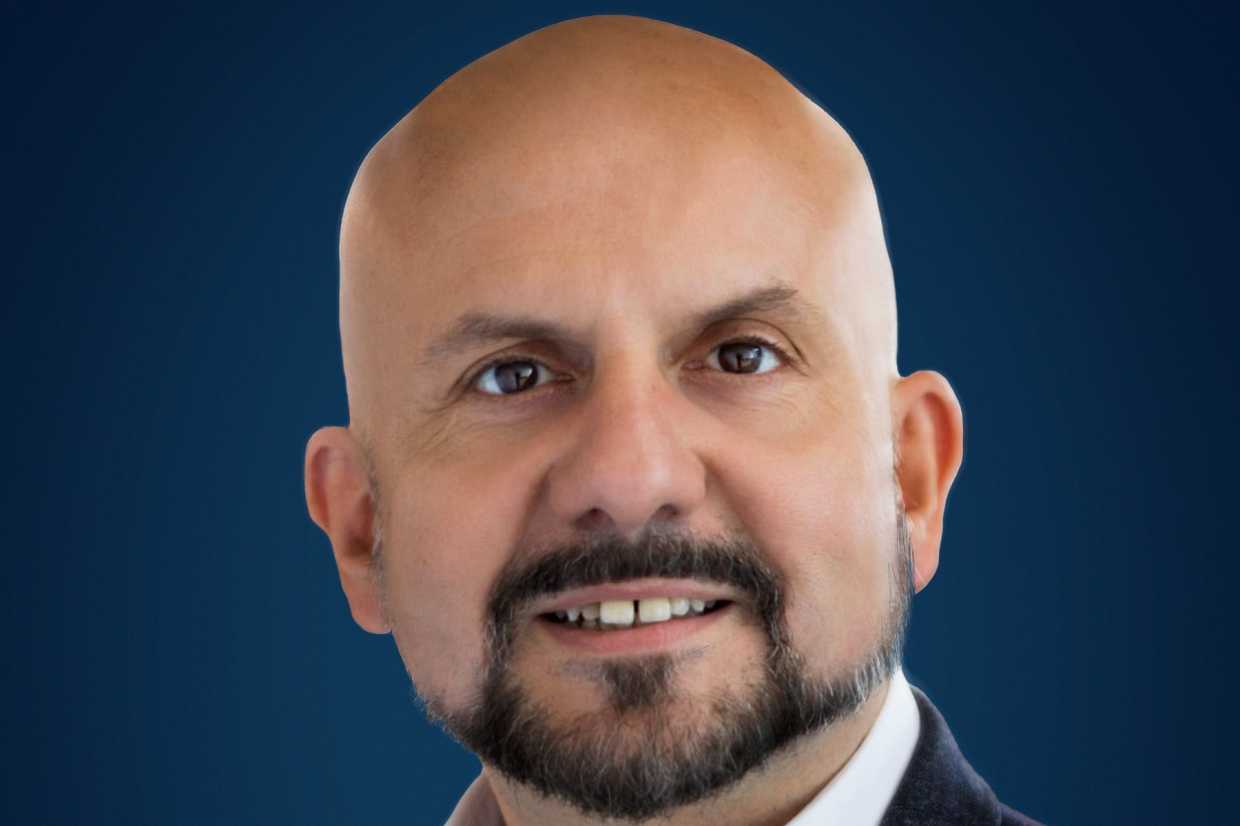
Roberto Hortal, chief product and technology officer, Wall Street English
Wall Street English
As an educational organization, Wall Street English integrates AI into their self-study programs. They use it for speech recognition to provide feedback on pronunciation, as well as a basis for conversation agents who let students practice conversational skills by mimicking real-life scenarios. The company established a governance framework that includes not only technology, finance, and legal considerations, but also ethics in a multicultural environment.
Protect the right things across the value chain
Because it uses code generation tools, Planview’s AI governance includes rules and guidelines that ensure they don’t infringe on copyrights. It also protect its own software so none of the code generation tools pick it up and reuse it elsewhere. Kersten says the company’s AI governance not only makes these points clear, but also tells users how to configure tools.
“GitHub Copilot has a setting that checks to make sure it doesn’t give you code that’s protected by copyright,” says Kersten. “Then another setting causes it to check your final code to make sure it isn’t too close to something in its repositories. There’s also a setting to tell GitHub Copilot not to keep your code.”
Naturally, what needs to be protected depends on the line of business. Whereas Planview is concerned with protecting IP, Wall Street English is mindful of cultural sensitivities. They adjust their course content to avoid offending students, and their AI tools need to do the same. “Just as we ringfence our online classes with trained teachers to guarantee nothing inappropriate is said, we must ensure that AI avoids expressing unintended opinions or inappropriate content,” says Hortal. “We employ techniques, such as input sanitization, contextual tracking, and content filtering, to mitigate risks and vulnerabilities. All of these things are part of our AI governance.”
Whatever you’re protecting, the rules shouldn’t stop within your own organization. Efforts should be made to ensure the same protections can be guaranteed when work is outsourced. “Some of the most sophisticated companies in the world have an amazing AI governance structure internally,” says Matt Kunkel, CEO of LogicGate, a software company that provides a holistic governance, risk, and compliance (GRC) platform. “But then they ship all their data over to third parties who use that data with their large language models. If your third parties aren’t in agreement with your AI usage policies, then at that point, you lose control of AI governance.”
Start now
The most common advice coming from IT leaders who have already implemented AI governance is to start now. From the time IT leadership starts working on AI governance to when they communicate the rules across their organization could be months. A case in point, it took Planview about six months from when they began thinking through their policy to when they made it available to the whole company in their learning management system.
As one of the early adopters of AI, Kersten frequently talks publicly about Planview’s experience. “The organizations who wait are the ones that will fall behind,” he says. “Get your policies up there now. It’s not as hard as you think. And once they’re there, it’ll really help both how you build things internally and also what you offer the market externally.”
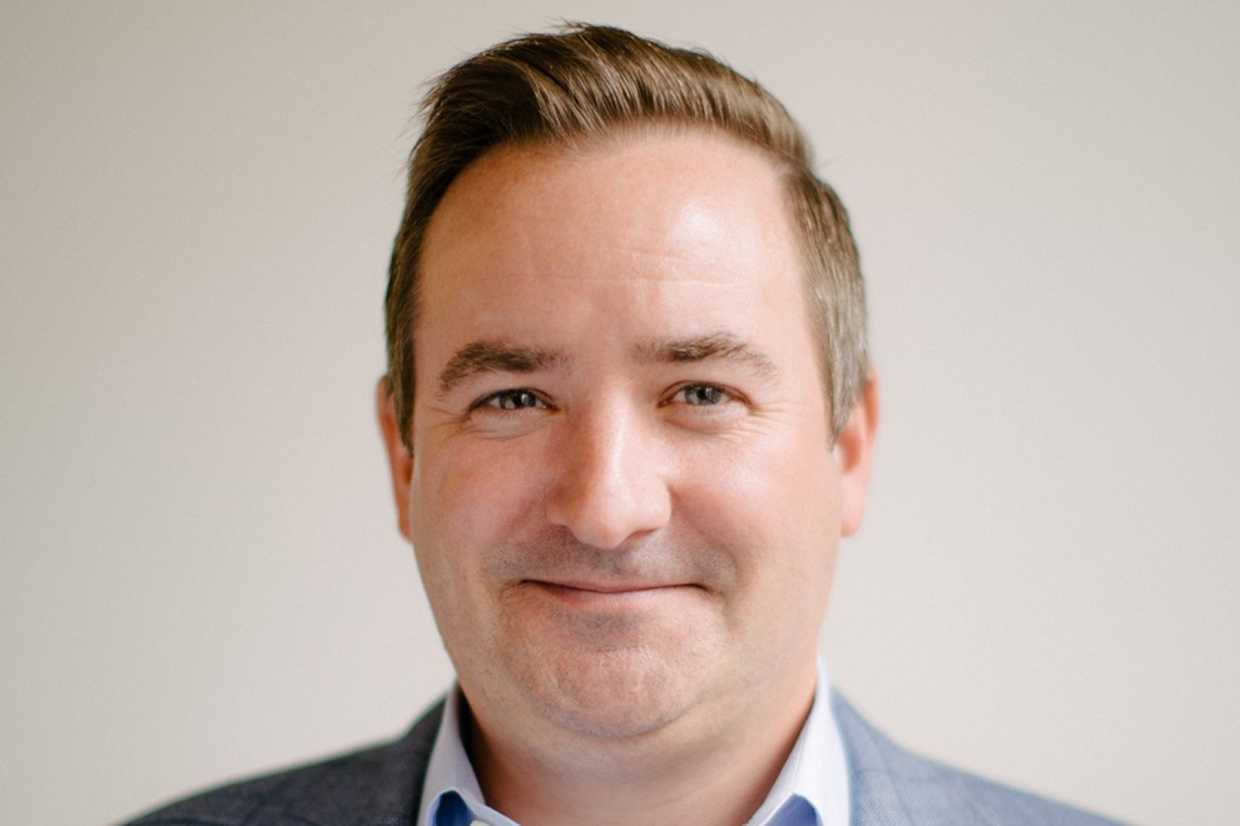
Matt Kunkel, CEO, LogicGate
LogicGate
Kunkel agrees. “Shadow use cases are already forming, so it’s important for CIOs to get a handle on AI policy as soon as possible,” he says. “One place to start is to build a consensus around the organization’s risk appetite concerning AI. People need to have a frank conversation about the balance they want to strike between moving fast and protecting customer data.”
Once you develop your governance and communicate it to the whole organization, people are free to focus on adding value.