- I ditched my daily driver Bose headphones for the XM6 - and I'm hesitant to go back
- This Lenovo ThinkPad is my top pick for remote work - and it's nearly 50% off now
- I invested in this 3-in-1 robot vacuum, and it's paying off for my home
- I've tested the Meta Ray-Bans for months, and these 5 features still amaze me
- My new favorite iPhone portable charger has a magnetic superpower - and it's cheap
70% of Businesses Prioritize Innovation Over Security in Generative AI
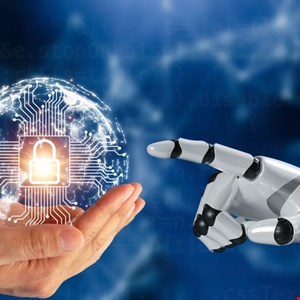
Businesses are being left vulnerable to a range of cybersecurity and privacy risks as 70% of business executives prioritize innovation over security when it comes to generative AI projects, according to a new report by IBM.
The survey also found that less than a quarter (24%) of generative AI projects are being secured.
This is despite 82% of respondents admitting that secure and trustworthy AI is essential to the success of their business.
Early Controls Key to Mitigating Breaches
Speaking to Infosecurity about the findings, Akiba Saeedi, VP of Data Security, IBM, said it is vital to avoid mistakes made in the past during the deployment of cloud technologies, which were often implemented without adequate security controls being built in.
For example, she noted that cloud misconfigurations are now one of the most common ways of threat actors infiltrate cloud environments. AI misconfigurations are also likely to be a major driver of breaches in the future if proper security controls are not established early on by organizations.
“We’re in that phase of education to really help organizations get more mature,” noted Saeedi.
The executives surveyed in the report highlighted a range of concerns relating to the deployment of generative AI tools in their organization. Over half (51%) cited unpredictable risks and new security vulnerabilities arising as a result of generative AI, while 47% highlighted new attacks targeting existing AI models, data and services.
The main forms of emergent threats to AI operations highlighted in the report were:
- Model extraction: Stealing a model’s behavior by observing the relationships between inputs and outputs
- Prompt injection: Manipulating AI models into performing unintended actions by dropping guardrails and limitations put in place by the developers
- Inversion exploits: Information on the data used to train a model being revealed
- Data poisoning: Changing the behavior of AI models by altering the data used to train them
- Backdoor exploits: Altering a model subtly during training to cause unintended behaviors under certain triggers
- Model evasion: Circumventing the intended behavior of an AI model by crafting inputs that trick it
- Supply chain exploits: Generating harmful models that hide malicious behavior, or target vulnerabilities in systems connected to the AI models
- Data exfiltration: Accessing and stealing sensitive data used in training and tuning models through vulnerabilities, phishing or misused privilege credentials
Saeedi warned: “The generative AI model itself presents a new threat landscape that didn’t exist before.”
Securing Generative AI in the Workplace
Most (81%) respondents acknowledged that generative AI requires a fundamentally new security governance model to mitigate the types of risks posed to these technologies.
IBM noted that governments around the world are bringing in a range of AI regulations. These include the EU’s AI Act and President Joe Biden’s Executive Order ’Promoting the Use of Trustworthy AI in the Federal Government’ in the US.
This further necessitates a comprehensive governance strategy specifically for generative AI, the researchers said.
The following security considerations should be incorporated within this governance framework, according to the report:
- Conduct threat modelling to understand and manage emerging threat vectors
- Identify open-source and widely used models that have been thoroughly scanned for vulnerabilities, tested and vetted
- Manage training data workflows, such as using encryption in transit and at rest
- Protect training data from poisoning and exploits that could introduce inaccuracies or bias, compromising the model’s behavior
- Harden security for API and plug-in integrations to third-party models
- Monitor models over time for unexpected behaviors, malicious outputs and security vulnerabilities that may appear
- Use identity and access management practices to manage access to training data and models
- Manage compliance with laws and regulations for data privacy, security and responsible AI use
Saeedi emphasized that most mature organizations will already have a governance, risk and compliance framework that can be built upon to achieve such a model, working out the new measures required for generative AI.
She said the most important aspect of this remains having the correct basic security infrastructure in place.
“Data moves, and you need the data security and identity of who gets to access those systems to know the lifecycle of where that data is going,” Saeedi advised.
The security components must fit inside a wider AI governance program, encompassing aspects like bias and trustworthiness.
“You want to enthuse security context into that, not have it be two separate silos,” added Saeedi.
Shadow AI Emerging as a Threat
The report also emphasized the growing threat of ‘shadow AI’ to enterprises.
This issue arises from employee sharing private organizational data into third-party applications embedded with generative AI, such as OpenAI’s ChatGPT and Google Gemini. This can lead to:
- Sensitive or privileged data being exposed
- Proprietary data being incorporated into third-party models
- Exposing data artifacts that could be vulnerable should the vendor experience a data breach
Such risks are particularly difficult for security teams to assess and mitigate because they are unaware of the usage, the report stated.
IBM set out the following actions organizations should consider taking to mitigate the risk of shadow AI:
- Establish and communicate policies that address use of certain organizational data within public models and third-party applications
- Understand how third parties will use data from prompts and whether they will claim ownership of that data
- Assess the risks of third-party services and applications and understand which risks they are responsible for managing
- Implement controls to secure the application interface and monitor user activity, such as the content and context of prompt inputs/outputs