- Buy Microsoft Visio Professional or Microsoft Project Professional 2024 for just $80
- Get Microsoft Office Pro and Windows 11 Pro for 87% off with this bundle
- Buy or gift a Babbel subscription for 78% off to learn a new language - new low price
- Join BJ's Wholesale Club for just $20 right now to save on holiday shopping
- This $28 'magic arm' makes taking pictures so much easier (and it's only $20 for Black Friday)
5 perspectives on modern data analytics
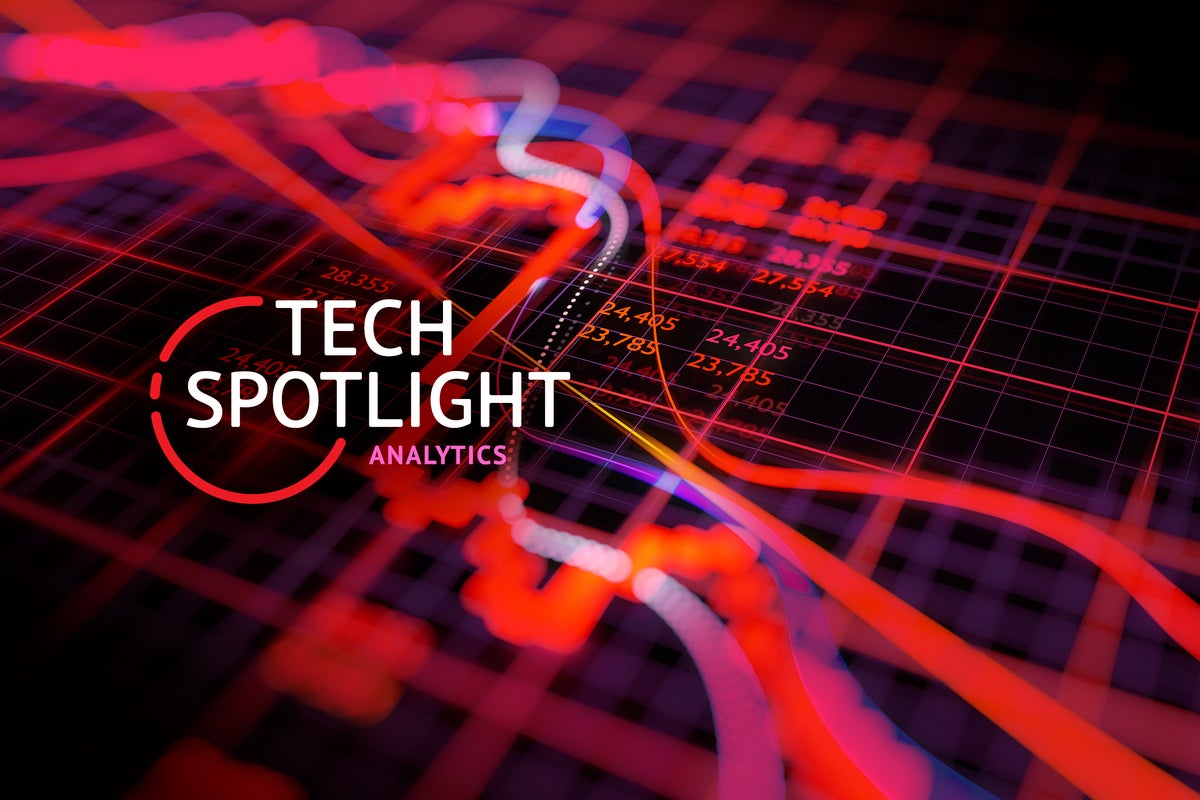
Some things don’t change, even during a pandemic. Consistent with previous years, in CIO’s 2021 State of the CIO survey, a plurality of the 1,062 IT leaders surveyed chose “data/business analytics” as the No.1 tech initiative expected to drive IT investment.
Unfortunately, analytics initiatives seldom do nearly as well when it comes to stakeholder satisfaction.
Last year, CIO contributor Mary K. Pratt offered an excellent analysis of why data analytics initiatives still fail, including poor-quality or siloed data, vague rather than targeted business objectives, and clunky one-size-fits-all feature sets. But a number of fresh approaches and technologies are making these pratfalls less likely.
In this bundle of articles from CIO, Computerworld, CSO, InfoWorld, and Network World, you’ll find advice and examples that can help ensure your own analytics efforts deliver the goods. These initiatives tend to resemble dev projects – even when commercial products are involved – and feature the same well-defined goals and iterative cycles that distinguish successful software development outcomes.
To get the big picture, start with the InfoWorld primer “How to excel with data analytics” by contributor Bob Violino. In this crisply written piece, Violino covers all the bases: establishing analytics centers of excellence; the benefits of self-service solutions (such as Tableau or Power BI); the exciting possibilities for machine learning; and the swing toward cloud analytics solutions. Violino expands on that last point in a second article, this one for CIO: “Analytics in the cloud: Key challenges and how to overcome them.” As he observes, the cloud’s scalability and abundant analytics tools may be irresistible, but migrating masses of company data to the cloud and securing it can be a heart-pounding adventure.
New technology invariably incurs new risks. No advancement has had more momentous impact on analytics than machine learning – from automating data prep to detecting meaningful patterns in data – but it also adds an unforeseen hazard. As CSO Senior Writer Lucian Constantin explains in “How data poisoning attacks corrupt machine learning models,” deliberately skewed data injected by malicious hackers can tilt models toward some nefarious goal. The result could be, say, manipulated product recommendations, or even the ability for hackers to infer confidential underlying data.
Without question, analytics has a dark side, as Matthew Finnegan corroborates in the Computerworld article “Collaboration analytics: Yes, you can track employees. Should you?” Collecting and analyzing metadata about user interactions on collaboration platforms has its legitimate benefits, such the ability to identify communication bottlenecks or to optimize the employee experience. But the same platforms can be used as employee monitoring systems that invade privacy and degrade trust between management and everyone else.
On a lighter note, consider this fine case study about analytics boosting user satisfaction: “Major League Baseball makes a run at network visibility.” Writing for Network World, Senior Editor Ann Bednarz examines how MLB employs network flow analysis software across its infrastructure to ensure players and fans enjoy consistent network performance – end-to-end, from Wi-Fi in the seats to cloud services.
That effort to deploy unified network analytics to optimize the user experience began just two years ago, mainly because MLB’s new principal network automation software engineer saw the necessity. His realization broke through perhaps the most important barrier to successful analytics initiatives: cultural inertia.
In the end, the secret to successful analytics is not in choosing and implementing the perfect technology, but in cultivating a broad understanding that pervasive analytics yields better decisions and superior outcomes. Usually, you can iron out technology kinks or requirements misunderstandings. But if you can’t change the mindset, few will use the beautiful analytics machine you just built.
Copyright © 2021 IDG Communications, Inc.