- This 3-in-1 MagSafe dock will charge your Apple devices while keeping them cool (and for Black Friday it's only $48)
- Why Cisco Leads with Wi-Fi 7: Transforming Future Connectivity
- What is AI networking? How it automates your infrastructure (but faces challenges)
- I traveled with a solar panel that's lighter than a MacBook, and it's my new backpack essential (and now get 23% off for Black Friday)
- Windows 11 24H2 hit by a brand new bug, but there's a workaround
Deloitte: How sensitive AI data may become more private and secure in 2022
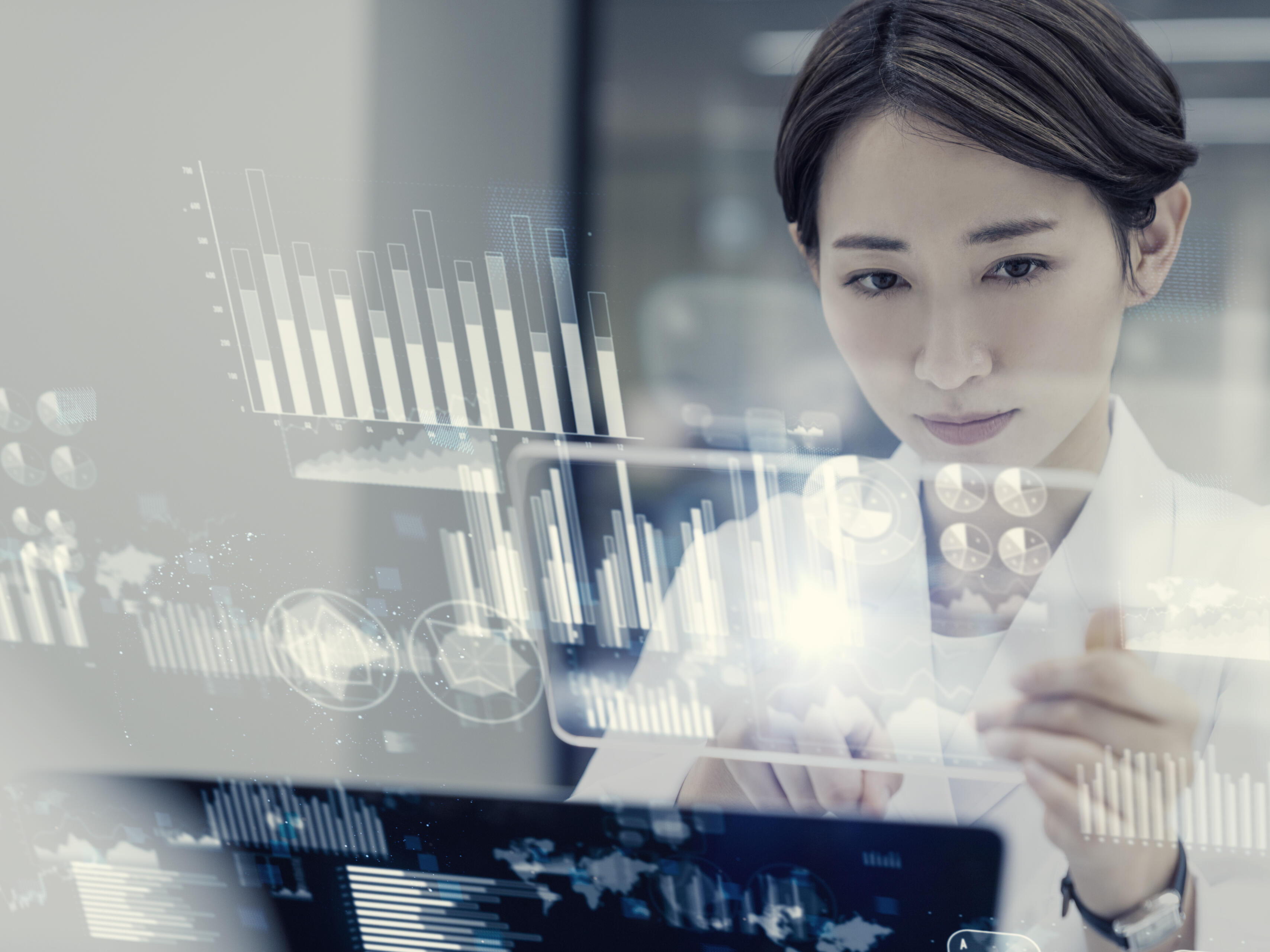
Technologies are available to better protect the data used in artificial intelligence, but they’re not quite ready for prime time, says Deloitte.
Image: iStock/metamorworks
With consumers concerned about their privacy and security, ensuring that user data is protected should be a top priority for any organization. That’s enough of a challenge with conventional processes. But throw artificial intelligence into the mix, and the obstacles become even greater. New tools that can better safeguard AI-based data are already here. Though they’re not yet practical, organizations should be aware of how they may play out in 2022 and beyond.
SEE: Artificial intelligence ethics policy (TechRepublic Premium)
In a report released on Wednesday, consulting firm Deloitte describes two tools that can make AI tasks such as machine learning more private and secure. Known as homomorphic encryption and federated learning, these are part of a group called privacy-enhancing technologies.
HE allows machine learning systems to use data while it’s encrypted. Normally, such data needs to be decrypted before the system can process it, which makes it vulnerable to compromise. FL deploys machine learning to local or edge devices so that the data is not all in one place where it could more easily be breached or hacked. Both HE and FL can be used at the same time, according to Deloitte.
Organizations that use artificial intelligence have already been eyeing HE and FL as a way to better secure their data. One advantage is that the use of these tools could satisfy regulators that are looking to impose new security and privacy requirements on such data. Cloud companies are interested in HE and FL because their data needs to be sent to and from the cloud and processed off premises. Other sectors, such as health care and public safety, are also starting to examine these tools in response to privacy concerns.
SEE: Metaverse cheat sheet: Everything you need to know (free PDF) (TechRepublic)
There are some technological obstacles to using HE and FL. Processing encrypted data with HE is slower than processing unencrypted data. And for FL to play a role, you need fast and powerful machines and devices on the edge where the actual machine learning occurs. In this case, an edge device could be something as simple as a smartphone or a more complex item such as factory equipment, according to Deloitte.
Progress is being made to surmount the obstacles. Wi-Fi 6 and 5G have brought faster and more reliable connectivity to edge devices. Thanks to new and speedier hardware, processing data with HE is now only 20% slower than processing unencrypted data, whereas in the past, it was a trillion times slower, Deloitte said. Even the processors that power FL are getting more robust and less expensive, leading to a wider deployment.
Another bonus is that 19 major tech players have already publicly announced initial tests and products for HE and FL. Though that sounds like a small number, the companies involved in these efforts include Apple, Google, Microsoft, Nvidia, IBM, while users and investors encompass DARPA, Intel, Oracle and Mastercard.
Though HE and FL still aren’t yet pragmatic in terms of cost and performance, organizations that need to focus on the security and privacy of AI-based data should be aware of their potential. These tools may be of particular interest to cloud providers and cloud users, businesses in sensitive industries such as health care and finance, public sector companies that deal with crime and justice, companies that want to exchange data with competitors but still retain their intellectual property and chief information security officers and their teams.
For organizations that want to investigate HE and FL, Deloitte offers the following suggestions:
- Understand the impact on your industry. What implications could HE and FL have on your industry as well as similar industries? How would a more secure and private AI affect your company strategically and competitively? To try to answer these questions, monitor the progress of these tools to see how other companies are working with them.
- Create a strategy. Until HE and FL gain more maturity, your existing strategy may be to do nothing about them. But you need to plan for the future by monitoring for trigger events that will tell you when it’s time to begin your investment and analysis. And for that, you’ll want skilled and knowledgeable people to help you develop the right strategy.
- Monitor technology developments. As HE and FL mature, your strategy surrounding these tools should change. Be sure to adjust your strategy so that you catch new developments before they pass you by.
- Bring in cybersecurity earlier rather than later. When evaluating HE and FL, make sure you bake cybersecurity into your strategy early on during the deployment stage.
“Privacy and security technologies, including HE and FL, are tools, not panaceas,” Deloitte said in its report. “But while no tools are perfect, HE and FL are valuable additions to the mix. By helping to protect the data that lies at the heart of AI, they can expand AI to more and more powerful uses, with the promise of benefiting individuals, businesses and societies alike.”