- Potential Nvidia chip shortage looms as Chinese customers rush to beat US sales ban
- These tech markets are taking the brunt of the new US tariffs - what that means for you
- JALを救ったSAKURAプロジェクト:50年ぶりの改革と復活の全貌
- IBM Cloud speeds AI workloads with Intel Gaudi 3 accelerators
- Change these 5 settings on your TV for a quick and easy way to improve its picture quality
Why the Future of Threat Detection and Prevention is Unified Security and Risk Analytics
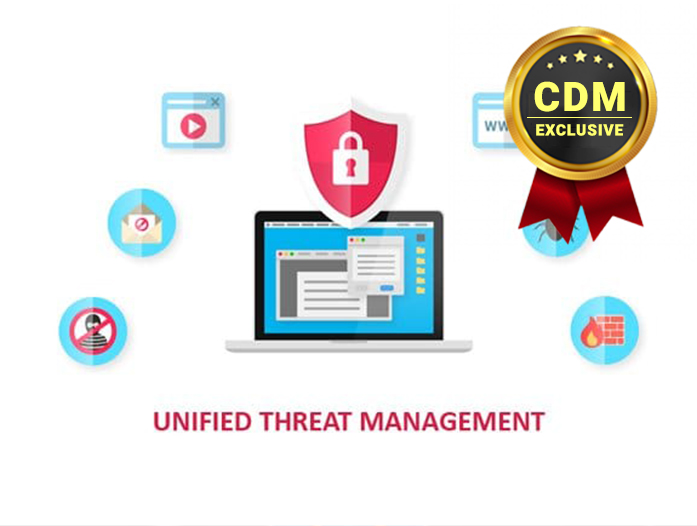
Why True AI/ML Capabilities are Essential for Next-Gen Risk Analytics
By Sanjay Raja, VP of Product Marketing at Gurucul
As cloud adoption continues to grow and remote work becomes the new normal, security teams are facing increased challenges with decreased visibility and a larger influx of security event data. As ransomware attacks continues to rise (i.e., recent SonicWall data showed 148% increase through Q3’21), SecOps teams are struggling to identify attacks before damage is done. As a result, they’re chasing solutions that accelerate detection and response, while increasing operational efficiencies.
Unfortunately, in many cases vendor claims only provided minimal improvements that are not keeping pace with the today’s threat actors. Traditional SIEMs and Endpoint-focused XDR are not fulfilling the promise of reducing the burden on understaffed security teams. The volume of alerts and false positives make it an uphill battle. For organizations wanting to reduce cyber risk across the on-prem, cloud, and remote infrastructures commonly supported today, security teams need to leverage unified data collection, a multitude of analytics, non-rule-based Machine Learning (ML) and Artificial Intelligence (AI), consolidated investigation interfaces, and targeted automation for faster response.
A very small set of next-gen SIEM solutions are innovating through more unified security and risk analytics capabilities that are crucial for success today. In this article, I’d like to explore why the future of threat detection and response is stemming from these new advancements.
SIEM was initially designed primarily for log collection and storage for compliance, then evolved to include the correlation of more log data sources for threat detection. Over time that functionality increased to integrate log, network, and endpoint data into a single location and match it up with security events. These helped analysts investigate commonalities or groups of related events. And as rules were developed around these related events, the SIEM could help to detect known threats.
Then came the rise of the terms like Machine Learning and Artificial Intelligence (ML/AI) – offering the promise of a silver bullet to solve threat detection and response. However, these terms were commonly misused and in reality, were just rule-based analytics engines that would conditionally gather more data for greater context. However, as attackers stayed hidden inside the network longer, rule-based analytics often failed to correlate seemingly disparate events across time and continued to focus on known attacks. As a result, new, unknown, and emerging attacks and variants were easily able to avoid detection. Furthermore, SIEM was also traditionally plagued by the lack of cloud-native offerings that were built to handle both cloud and hybrid infrastructures equally.
Today, newer advancements in SIEM are focused in several areas designed to make it the primary platform for the security operations center (SOC). This includes security monitoring, improved threat detection, and playbooks to drive faster response. Many EDR, XDR, and SIEM solutions that claim to use ML/AI continue to use rule-based engines with finite models, patterns, and signatures that are not updated fast enough when new attacks are discovered.
However, there are next-gen SIEM solutions incorporating unified security and risk analytics that are taking the extra step to deliver out-of-the-box advanced data modeling across cloud, user, network, asset, endpoint, and log telemetry. The few that offer true ML/AI can automatically detect new, unknown, and emerging attacks, including subtle variants. Along with an understanding of user access and entitlements, behavioral modeling, and risk metrics, the end goal of next-generation SIEM is to streamline every facet of the SOC. This includes reducing noise and false positives, prioritizing which IoCs need to be investigated, consolidating data for easier investigations, and providing high confidence, low-risk automated response to prevent a successful attack.
What does that mean? Let’s look at the key elements of unified security and risk analytics in a next-generation SIEM.
- Unified Correlation, Continuous Risk Profiling, and Behavioral Anomaly Detection – A Next-generation SIEM must unify data collection across the entire infrastructure, on-prem, cloud, and remote, by gathering endpoint, log, user, access, entity/asset, network, and other data to provide greater context. With risk profiling applied to abnormal behaviors, a behavior-based risk can be calculated to elevate which events are truly relevant for investigation, or can even be used to determine an immediate threat with conviction. This shrinks the noise created by false positives and provides more context to enable a much more targeted response, ideally before an attack campaign starts to establish itself.
- Identity and Access Analytics – Next-gen SIEM uses Identity Analytics (IDA) leveraging data science that monitors for and identifies risky access controls, entitlements, user behaviors, and associated abnormal or deviant activity. These types of advanced analytics data can also serve as key indicators for provisioning, de-provisioning, authentication, and privileged access management by IAM teams. IdA surpasses human capabilities by leveraging machine learning models to define, review and confirm accounts and entitlements for access, and works with risk analytics to prioritize suspicious activity as more malicious.
- Cross-Channel Fraud Prevention – Next-gen SIEM offers modern fraud detection capabilities with the ability to link data from a multitude of sources to provide a contextual view of what’s happening in the environment. Such platforms highlight anomalous transactions based on historic user and community profiles so analysts can initiate investigations or execute automated remediation actions. It analyzes online and offline activity, including public records, contact center interactions, point of sale transactions, ATM transactions, and more. It mines and normalizes data and then creates a risk score for fraud and abuse which can be used for real-time decision making.
The ability to combine these elements to best suit the needs of an organization offer SecOps power and flexibility when protecting users and the business from data exfiltration, cyber fraud, privilege access abuse, account compromise and more – using behavior and context. As a result, teams can prioritize risks and alerts, quickly investigate problems, automate risk response, have a comprehensive view of case management, conduct contextual natural language search, and more, all consolidated into a single management console.
As the consolidation of security capabilities continues, providers are working to layer on more capabilities to further unify security, including UEBA, SOAR, and XDR. They’re also working to provide better security and to lower capital and operational requirements, including scaling, training, management, and maintenance. In addition, security operations teams have long invested and been focused on external threats. This has led to a lack of monitoring for insider threats. As part of the foundation of a successful security program, teams must monitor for both external and internal threats. And a mature UEBA set of capabilities should be incorporated to fully protect the organization.
What questions should you be asking today about your SIEM or to your SIEM provider?
- How is the SIEM platform delivered? The ability to run as a collection of services entirely within the cloud makes it ideal for risk analysis of security data. Organizations have the advantage of aggregating and analyzing data from worldwide sources in a single application instance. These platforms must also scale (both up and down) to accommodate varying workloads. Furthermore, a cloud-native solution is often easier to maintain over time since the vendor can perform upgrades quickly, and in real-time.
- Do they offer open analytics and allow teams to easily modify and build customer ML models? Open analytics are critical for security teams to be able to customize their ML models to suit their specific needs or build their own models. It’s important to understand exactly what goes into a model to be confident in its output. With black box analytics, results must be taken on faith since nobody knows how the answers are obtained, or if the results are valid.
- What are my options for data lake? Where and how data is stored is a critical factor in the flexibility, speed, quality, and cost of security data processing, ingestion, and storage. Open choice of big data offers major economic advantages over traditional data warehouses for scaling to terabytes or petabytes. It’s imperative that a SIEM platform works with what you already have or plan to purchase versus being locked into a proprietary vendor data lake.
- What does the risk modeling approach look like? Look for a platform that offers self-learning, self-training, and contextually aware algorithms that score every transaction as they’re evaluated in near real-time. This requires a comprehensive risk engine that performs continuous risk scoring and can provide real-time risk prioritized alerts for incident analysis. The risk scoring framework needs to roll up risk scores from multiple contributing elements (with the ability to deliver normalized user and entity risk scores). As a result, a finite number of targeted response actions can be defined that are both targeted and driven by high-fidelity automation and thereby accelerating threat response.
SIEM is not just about ingesting data sources. To empower security teams these solutions must deliver a variety of capabilities. This includes providing actionable context of the ingested data, reducing noise, and identifying and prioritizing the right events associated with an attack. It also means delivering highly accurate and targeted investigation capabilities with confirmation of the attack and high-confidence automated responses. Finally, these solutions need to thwart the successful detonation of ransomware or the execution of the main attack purpose (corruption, disruption, or theft).
A next-generation SIEM with unified security and risk analytics should be the core of a successful security operations program. Security teams must evaluate innovative technologies that continue to improve and consolidate analytical capabilities to provide a more usable platform that also improves the ROI of the SOC program.
About the Author
Sanjay Raja brings over 20 years of experience in building, marketing and selling cyber security and networking solutions to enterprises, medium-to-small business, and managed service providers. Previously, Sanjay was VP of Marketing at Prevailion, a cyber intelligence startup. Sanjay has also several successful leadership roles in Marketing, Product Strategy, Alliances and Engineering at Digital Defense (acquired by Help Systems), Lumeta (acquired by Firemon), RSA (Netwitness), Cisco Systems, HP Enterprise Security, Crossbeam Systems, Arbor Networks, Top Layer Networks, Caw Networks (acquired by Spirent Communications), Nexsi Systems, 3Com, and Cabletron Systems. Sanjay holds a B.S.EE and an MBA from Worcester Polytechnic Institute.
Sanjay can be reached online at our company website https://gurucul.com
FAIR USE NOTICE: Under the “fair use” act, another author may make limited use of the original author’s work without asking permission. Pursuant to 17 U.S. Code § 107, certain uses of copyrighted material “for purposes such as criticism, comment, news reporting, teaching (including multiple copies for classroom use), scholarship, or research, is not an infringement of copyright.” As a matter of policy, fair use is based on the belief that the public is entitled to freely use portions of copyrighted materials for purposes of commentary and criticism. The fair use privilege is perhaps the most significant limitation on a copyright owner’s exclusive rights. Cyber Defense Media Group is a news reporting company, reporting cyber news, events, information and much more at no charge at our website Cyber Defense Magazine. All images and reporting are done exclusively under the Fair Use of the US copyright act.