- Essential commands for Linux server management
- AI-Ready Infrastructure: A New Era of Data Center Design
- UK ICO Fines 23andMe £2.3m for Data Protection Failings
- Amazon Prime Day returns July 8-11: Here's what's new (and what to know)
- I tested Hisense's flagship QLED TV and didn't miss my OLED set (especially when it's $700 off)
How SASE uses AI
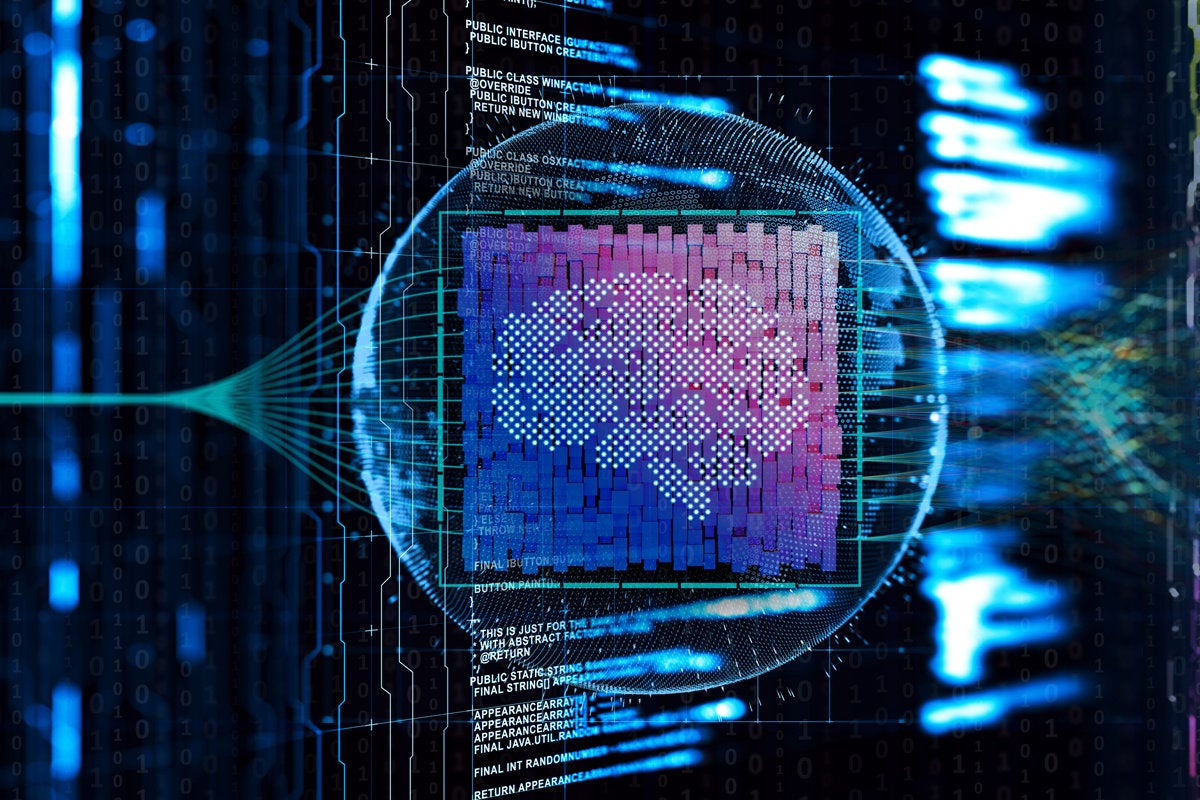
Secure access service edge, or SASE, combines networking and security into a cloud-based service, and it’s growing fast. According to Gartner projections, enterprise spending on SASE will hit almost $7 billion this year, up from under $5 billion in 2021. Gartner also predicts that more than 50% of organizations will have strategies to adopt SASE by 2025, up from less than 5% in 2020.
The five core components of the SASE stack are SD-WAN, firewall-as-a-service (FWaaS), secure web gateway (SWG), cloud access security broker (CASB), and zero trust network access (ZTNA).
There’s also a sixth key technology that’s increasingly playing a big role in every aspect of SASE, and that’s artificial intelligence. “It’s something that most, if not all, SASE vendors are working on,” says Gartner analyst Joe Skorupa.
It helps that SASE vendors are already sitting on some of the biggest collections of network and cybersecurity-related data on the planet. Some forward-thinking vendors started collecting this data even before the AI and machine-learning algorithms were fully developed, Skorupa says.
“Some of the vendors that I talked to on the SD-WAN side were building their data lakes five years ago, knowing that it would be valuable even though they couldn’t build the ML capabilities they wanted then,” he says. “It’s the same thing on the security side — I think the vendors have seen the promise, and it has simply taken time for the enabling technologies to mature.”
Because they collect global data about networking and cybersecurity threats, SASE vendors can learn faster than individual companies working with their own limited data sets. As a result, the AI and machine learning used by SASE vendors has the potential to become smarter and faster than that of individual companies, particularly those that are smaller to mid-sized.
Gartner predicts that artificial intelligence and automation capabilities will become key features enterprises look for when choosing a SASE vendor. While many of these are still in development, here are some capabilities to expect in future releases.
Reducing false positive alerts
The College of Southern Nevada had to support more than 40,000 remote students, faculty, and staff overnight due to the pandemic, and, as many enterprises did at the time, moved to SASE for ease of deployment and scalability.
Better security was a welcome bonus, in particular the way the system reduced false-positive alerts using AI, says Mugunth Vaithylingam, the college’s chief experience officer.
“Our SASE provider, Open Systems, uses AI to eliminate false positive alerts, which we were previously flooded with,” Vaithylingam says. “Now, instead of being overwhelmed — and sometimes paralyzed — by all these alerts, my internal network and security teams can focus on their tasks with vastly greater efficiency.”
He’s not the only one facing this issue.
Alert fatigue is real — security analysts are suffering due to the large volume of alerts they have to manage on a daily basis. In a global survey of 800 IT professionals released this March by cloud security company Orca Security, 60% said they’re receiving more than 500 cloud security alerts every day, and the volume of work caused 55% of respondents to miss critical alerts on a daily or weekly basis.
Orange Cybersecurity, a cybersecurity firm which processes more than 60 billion security events daily, analyzed nearly 100,000 cybersecurity incidents last year and found that 40% were false positives.
Open Systems uses AI for security and incident anomaly detection and to classify incidents, says Stefan Keller, vice president for SASE at the vendor. “The chief benefits are an increased detection rate along with a dramatic reduction of the false positive rate,” he says.
Network analysis and repair
In the big picture, enterprises are moving toward autonomous networks that leverage AI and machine learning to make decisions with little or no human intervention. In a SASE environment, that might take the form of automated network traffic analysis, for example.
SD-WAN that utilizes AI can track traffic peaks to avoid performance problems. It might suggest that a company should think about ordering more bandwidth for a particular link or branch office, or that it should update its traffic steering policies, says Gartner’s Skorupa. “They could move some traffic off of that particular link and free up what they need without having to buy more bandwidth.”
An AI-driven network could move workloads or shift user access when a service level isn’t being met, adds Abe Ankumah, vice president of product management for VMware SASE. “That could be making global routing decisions, or that could be steering traffic to a different application resource or a different cloud,” Ankumah says.
Skorupa warns that earning customer trust will be a longer-term challenge. Ops isn’t going to hand everything over to the algorithms, he says. “You have to demonstrate as a vendor that you bring valuable insights and that the suggested changes would be valuable on the network side.”
Gartner tells clients to think about day-two operation and management – the day-to-day use of networking products and services in their environments. “Think about those long-term operational issues,” Skorupa says.
Less than 5% of enterprises with SD-WAN deployments used artificial intelligence functions to automate day-two operations in 2021, according to Gartner, but the number’s expected to reach 40% by 2025.
Predictive maintenance
Another clear use case for AI is predictive maintenance, Skorupa says.
“You get predictive analytics running in a branch office looking at SD-WAN devices, and it shows you that the optical transceiver is demonstrating behaviors that show it’s going to fail in the next few days,” he says. “Will you be comfortable having the algorithm reach out to the folks who do hardware support and send a technician out to do the fix? Absolutely.”
Predictive maintenance has already become popular in other applications for AI. For example, in manufacturing, it’s one of the top use cases, according to McKinsey’s 2021 state of AI report, released in December.
And in network performance monitoring, it’s AI, predictive analytics and machine learning that are propelling growth in the market, according to a report released by Persistence Market Research.
User and entity behavior analytics
SASE vendors have access to a lot of data, which they can use to establish a baseline for how humans and devices should act within a network, which can help both in authentication and in spotting suspicious activity.
“From a network perspective, there is a need to ensure the identity of the entities connecting to the network,” says Trent Fierro, senior marketing manager for cloud and AIOps solutions marketing at Aruba, a Hewlett Packard Enterprise company.
AI models can quickly identify the type of endpoints connecting to a network, profile each client that’s accessing a network, and give security experts an awareness of what’s on their networks, Fierro says.
At Aruba, the company has telemetry from more than 120,000 customer sites, 120 million endpoint clients, and nearly 2 million infrastructure devices from which it can train its models, he says.
Anomaly detection
Anomaly detection is a type of machine-learning algorithm that detects activity that doesn’t fit normal patterns. It’s one of the biggest use cases for AI in cybersecurity, and it can be dramatically effective when used against SASE vendors’ large cybersecurity and networking data sets.
“AI is immensely valuable when used to detect behaviors that aren’t inherently good or bad but are hard to detect with traditional techniques,” says Aaron Sant-Miller, data scientist at information technology consulting firm Booz Allen Hamilton. When the results are provided to analysts, they can review the information and decide if a malicious threat is moving down the cyber kill chain, he says.
However, not all anomalous behavior is easy to classify.
“Anomaly detection systems struggle because many anomalous behaviors are benign and not inherently malicious,” says Sant-Miller. “This can drive up false-positive rates for analysts, fueling distrust in AI.”
Also, behaviors on one network are determined by how it’s configured, so taking an AI capability that’s built for a specific network’s data and running it on another can result in false alerts, he added.
Data loss prevention
Data loss prevention isn’t a core SASE feature, but it’s one that many SASE vendors have recently added or are in the process of rolling out. It prevents sensitive data from being exfiltrated from within a company’s systems, either by external attackers or malicious insiders.
When augmented with AI, data loss prevention tools can identify data that was deliberately obfuscated in order to get past simple keyword-based filters.
Insider threat is one of the biggest issues enterprises face today, says Krishna Naraynaswamy, chief technology officer at Netskope.
“Departing employees tend to take sensitive information like design documents and code that they contributed to while working in the company,” he says. “Malicious insiders also steal company data and share it externally.”
AI can track sensitive information that a person already has in their possession — even if a file is taken outside of a company’s network, he says.
But AI can do more from keeping data from leaving a company. It can also deny access to that data in the first place.
AI algorithms can maintain a risk score for every user — similar to a credit score — and feed the score into zero-trust access policies, Naraynaswamy says. “A user with a poor user risk score can be denied access to sensitive data.”
Some SASE vendors include data loss prevention technology in the agents that end users have running on their machines, says Gartner’s Skorupa.
So, for example, a malicious user might try to take a screenshot of a spreadsheet in order to steal the data and then send it out, he says. “And it gets blocked.”
“I could disconnect from the company VPN so the company isn’t seeing my network traffic and drag it into my Gmail, and it still gets blocked,” Skorupa adds. That’s because the SASE vendor’s agent has been tracking the sensitive information while it was transformed
Not all SASE vendors offer this technology yet, he says, but about a handful already do.
Identifying and preventing zero-day attacks
Traditional intrusion-detection systems are good at detecting known vulnerabilities and can prevent the same attack from happening again, but they can be slow to respond to new threats. “It’s always easier to prevent an attack that has already happened,” says Anand Oswal, senior vice president at Palo Alto Networks.
By training AI models with all the known vulnerabilities and exploits, attacks that haven’t happened yet can be discovered and stopped immediately — and many new attacks are different versions of previously known threats.
Some 90% of malware is actually variations of existing malware, Oswal says. “So we can use our AI engine to stop this malware by pushing the machine-learning models on the platforms and stopping them in real-time.”
While some threats benefit from monitoring and automatic mitigation, more complicated attacks should still involve security experts directly, says Gartner’s Skorupa. “You can certainly get false positives on the security side, so you may very well have some senior engineering staff looking at some of these things.”
DDoS mitigation
The continuing growth in unsecured connected devices, the move to high-speed 5G networks, and the expansion of the DDoS-as-a-service industry are combining for a perfect storm when it comes to distributed denial-of-service (DDoS) attacks.
Research firm Spamhaus reported more than 3,200 botnet command-and-control servers in the fourth quarter of 2021, up from under 1,400 in the fourth quarter of 2020.
In January, Microsoft reported the largest DDoS attack it has ever recorded, at 3.47 terabits per second and a rate of 340 million packets per second. Meanwhile, Cloudflare reported a record-breaking volumetric attack, with 17 million requests per second last summer. That’s almost three times larger than any attack the company had seen before.
With a blunt attack like a DDoS, companies need to have algorithms that very rapidly mitigate the threat, says Skorupa. DDoS mitigation is a common feature offered by SASE vendors. It’s also one of the easiest things for companies to trust AI to handle, says Skorupa.
Easing the burden on security analysts
When repetitive and routine tasks can be handled by AI, security analysts can spend their time on more complicated issues.
AI serves as a force multiplier that augments a security professional’s job by learning their tendencies and preferences and helping them to complete their daily work more efficiently, says Booz Allen Hamilton data scientist Colin Friedman.
“The goal is to support AI adoption so that the people with the expertise can dedicate their focus to the things that require their skill sets and less of the arduous tasking that consumes valuable time,” he says.
But AI isn’t ready to work without humans in the loop, he warns. “I don’t think we’re in a place or want to be in a place where AI is removing human intervention,” says Friedman.
Real AI benefits yet to come
Looking ahead, the true value of AI for SASE applications will come later, when vendors are able to offer full-stack observability of their systems, says Ron Howell, managing architect and engineer for SD-WAN and SASE at consulting firm Capgemini Americas.
“AI within SASE depends on the SASE solution chosen and used,” he says. “Proactive visibility is the primary key.”
Companies need to have observability in the full stack of network, security, and applications, he says. “A few of the SASE vendors are beginning to include AI capabilities in AIOps and measurement. However, many of the SASE solutions are not ready for AI or full stack observability.”
AI is still in its early stages in almost every SASE solution, he says. “The long-term potential is a proactive end-to-end secure network as a service,” he says.
At the same time, enterprises themselves are still reluctant to trust AI to make key decisions. “They cannot afford downtime if something goes wrong,” he says. “Even though AI is valuable, we still need good engineers making solid decisions.”
Copyright © 2022 IDG Communications, Inc.