- I recommend the Pixel 9 to most people looking to upgrade - especially while it's $250 off
- Google's viral research assistant just got its own app - here's how it can help you
- Sony will give you a free 55-inch 4K TV right now - but this is the last day to qualify
- I've used virtually every Linux distro, but this one has a fresh perspective
- I replaced my JBL speaker with this surprise alternative. Here's why it's my new top pick
JetBlue optimizes data operations with shift to the cloud

The air travel industry has dealt with significant change and uncertainty in the wake of the COVID-19 pandemic. In 2020, JetBlue Airways decided its competitive advantage depended on IT — in particular, on transforming its data stack to consolidate data operations, operationalize customer feedback, reduce downstream effects of weather and delays, and ensure aircraft safety.
“Back in 2020, the data team at JetBlue began a multi-year transformation of the company’s data stack,” says Ashley Van Name, general manager of data engineering at JetBlue. “The goal was to enable access to more data in near real-time, ensure that data from all critical systems was integrated in one place, and to remove any compute and storage limitations that prevented crewmembers from building advanced analytical products in the past.”
Prior to this effort, JetBlue’s data operations were centered on an on-premises data warehouse that stored information for a handful of key systems. The data was updated on a daily or hourly basis depending on the data set, but that still caused data latency issues.
“This was severely limiting,” Van Name says. “It meant that crewmembers could not build self-service reporting products using real-time data. All operational reporting needed to be built on top of the operational data storage layer, which was highly protected and limited in the amount of compute that could be allocated for reporting purposes.”
Data availability and query performance were also issues. The on-premises data warehouse was a physical system with a pre-provisioned amount of storage and compute, meaning that queries were constantly competing with data storage for resources.
“Given that we couldn’t stop analysts from querying the data they needed, we weren’t able to integrate as many additional data sets as we may have wanted in the warehouse — effectively, in our case, the ‘compute’ requirement won out over storage,” Van Name says.
The system was also limited to running 32 concurrent queries at any one time, which created a queue of queries on a daily basis, contributing to longer query run-times.
The answer? The Long Island City, N.Y.-based airlines decided to look to the cloud.
Near real-time data engine
JetBlue partnered with data cloud specialist Snowflake to transform its data stack, first by moving the company’s data from its legacy on-premises system to the Snowflake data cloud, which Van Name says greatly alleviated many of the company’s most immediate issues.
Ashley Van Name, general manager of data engineering, JetBlue
JetBlue
Jet Blue’s data team then focused on integrating critical data sets that analysts had not previously been able to access in the on-premises system. The team made more than 50 feeds of near real-time data available to analysts, spanning the airline’s flight movement system, crew tracking system, reservations systems, notification managers, check-in-systems, and more. Data from those feeds is available in Snowflake within a minute of being received from source systems.
“We effectively grew our data offerings in Snowflake to greater than 500% of what was available in the on-premise warehouse,” Van Name says.
JetBlue’s data transformation journey is just beginning. Van Name says moving the data into the cloud is just one piece of the puzzle: The next challenge is ensuring that analysts have an easy way to interact with the data available in the platform.
“So far, we have done a lot of work to clean, organize, and standardize our data offerings, but there is still progress to be made,” she says. “We firmly believe that once data is integrated and cleaned, the data team’s focus needs to shift to data curation.”
Data curation is critical to ensuring analysts of all levels can interact with the company’s data, Van Name says, adding that building single, easy-to-use “fact” tables that can answer common questions about a data set will remove the barrier to entry that JetBlue has traditionally seen when new analysts start interacting with data.
In addition to near real-time reporting, the data is also serving as input for machine learning models.
“In addition to data curation, we have begun to accelerate our internal data science initiatives,” says Sai Pradhan Ravuru, general manager of data science and analytics at JetBlue. “Over the past year and a half, a new data science team has been stood up and has been working with the data in Snowflake to build machine learning algorithms that provide predictions about the state of our operations, and also enable us to learn more about our customers and their preferences.”
Ravuru says the data science team is currently working on a large-scale AI product to orchestrate efficiencies at JetBlue.
“The product is powered by second-degree curated data models built in close collaboration between the data engineering and data science teams to refresh the feature stores used in ML products,” Ravuru says. “Several offshoot ecosystems of ML products form the basis of a long-term strategy to fuel each team at JetBlue with predictive insights.”
Navigating change
JetBlue shifted to Snowflake nearly two years ago. Van Name says that over the past year, internal adoption of the platform has increased by almost 75%, as measured by monthly active users. There has also been a greater than 20% increase in the number of self-service reports developed by users.
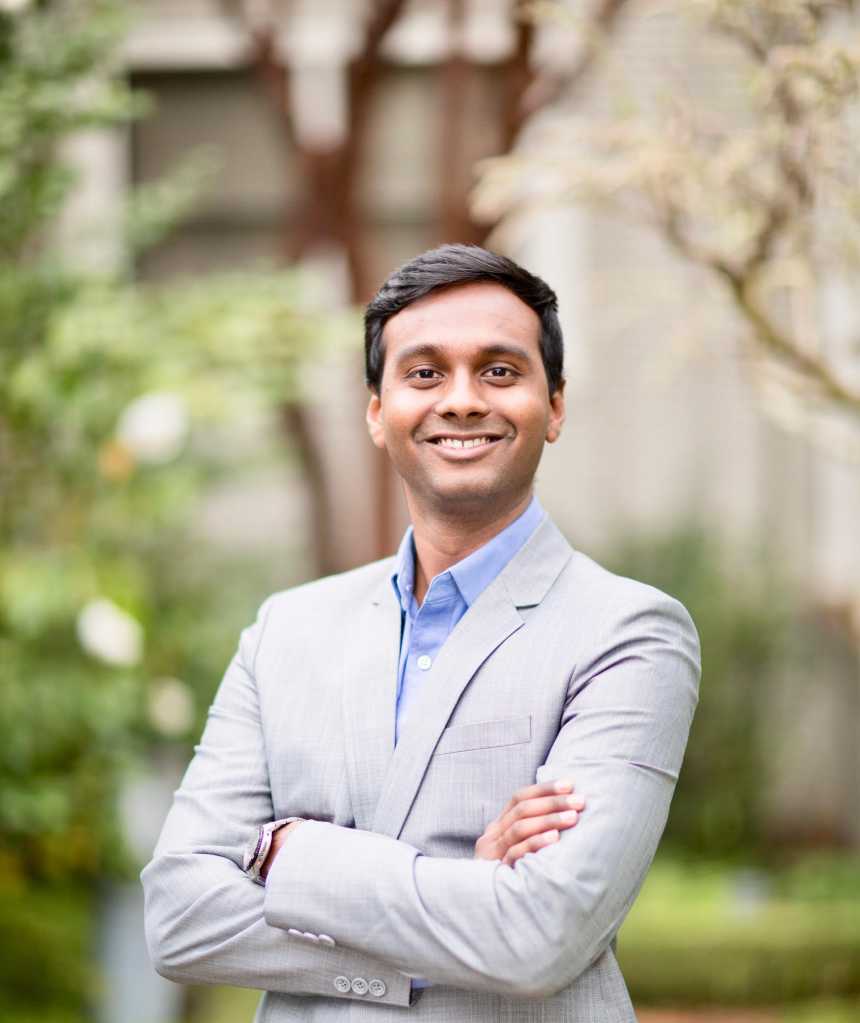
Sai Pradhan Ravuru, general manager of data science and analytics, JetBlue
JetBlue
Ravuru says his team has deployed two machine learning models to production, relating to dynamic pricing and customer personalization. Rapid prototyping and iteration are giving the team the ability to operationalize data models and ML products faster with each deployment.
“In addition, curated data models built agnostic of query latencies (i.e., queries per second) offer a flexible online feature store solution for the ML APIs developed by data scientists and AI and ML engineers,” Ravuru says. “Depending on the needs, the data is therefore served up in milliseconds or batches to strategically utilize the real-time streaming pipelines.”
While every company has its own unique challenges, Van Name believes adopting a data-focused mindset is a primary building block for supporting larger-scale change. It is especially important to ensure that leadership understands the current challenges and the technology options in the marketplace that can help alleviate those challenges, she says.
“Sometimes, it is challenging to have insight to all of the data problems that exist within a large organization,” Van Name says. “At JetBlue, we survey our data users on a yearly basis to get their feedback on an official forum. We use those responses to shape our strategy, and to get a better understanding of where we’re doing well and where we have opportunities for improvement. Receiving feedback is easy; putting it to action is where real change can be made.”
Van Name also notes that direct partnership with data-focused leaders throughout the organization is essential.
“Your data stack is only as good as the value that it brings to users,” she says. “As a technical data leader, you can take time to curate the best, most complete, and accurate set of information for your organization, but if no one is using it to make decisions or stay informed, it’s practically worthless. Building relationships with leaders of teams who can make use of the data will help to realize its full value.”