- GITEX GLOBAL 2024: Huawei to Accelerate Industrial Digitalization and Intelligence
- 5 ways to balance self-promotion with the demands of the business
- Learn a new language with Babbel for 69% off
- How to upgrade your 'incompatible' Windows 10 PC to Windows 11: Two options
- Wiping a Windows laptop? Here's the safest way to erase your personal data - for free
Building a vision for real-time artificial intelligence

By George Trujillo, Principal Data Strategist, DataStax
I recently had a conversation with a senior executive who had just landed at a new organization. He had been trying to gather new data insights but was frustrated at how long it was taking. (Sound familiar?) After walking his executive team through the data hops, flows, integrations, and processing across different ingestion software, databases, and analytical platforms, they were shocked by the complexity of their current data architecture and technology stack. It was obvious that things had to change for the organization to be able to execute at speed in real time.
Data is a key component when it comes to making accurate and timely recommendations and decisions in real time, particularly when organizations try to implement real-time artificial intelligence. Real-time AI involves processing data for making decisions within a given time frame. The time frame window can be in minutes, seconds, or milliseconds, based on the use case. Real-time AI brings together streaming data and machine learning algorithms to make fast and automated decisions; examples include recommendations, fraud detection, security monitoring, and chatbots.
A whole lot has to happen behind the scenes to succeed and get tangible business results. The underpinning architecture needs to include event-streaming technology, high-performing databases, and machine learning feature stores. All of this needs to work cohesively in a real-time ecosystem and support the speed and scale necessary to realize the business benefits of real-time AI.
It isn’t easy. Most current data architectures were designed for batch processing with analytics and machine learning models running on data warehouses and data lakes. Real-time AI requires a different mindset, different processes, and faster execution speed. In this article, I’ll share insights on aligning vision and leadership, as well as reducing complexity to make data actionable for delivering real-time AI solutions.
A real-time AI north star
More than once, I’ve seen senior executives completely aligned on mission while their teams fight subtle yet intense wars of attrition across different technologies, siloes, and beliefs on how to execute the vision.
A clear vision for executing a real-time AI strategy is a critical step to align executives and line-of-business leaders on how real-time AI will increase business value for the organization.
The execution plan must come from a shared vision that offers transparency and includes defining a laundry list of methodologies, technology stacks, scope, processes, cross-functional impacts, resources, and measurements with sufficient detail so that cross-functional teams have enough direction to collaborate and work together to achieve operational goals.
Machine learning models (algorithms that comb through data to recognize patterns or make decisions) rely on the quality and reliability of data created and maintained by application developers, data engineers, SREs, and data stewards. How well these teams work together will determine the speed they deliver real-time AI solutions. As real-time becomes pervasive across the organization, several questions begin to arise:
- How are cross-functional teams enabled to support the speed of change, agility, and quality of data for real-time AI, as ML models evolve?
- What level of alerting, observability, and profiling can be counted on to ensure trust in the data by the business?
- How do analysts and data scientists find, access, and understand the context around real-time data?
- How is data, process, and model drift managed for reliability?
- Downstream teams can create strategy drift without a clearly defined and managed execution strategy; is the strategy staying consistent, evolving, or beginning to drift?
- Real-time AI is a science project until benefits to the business are realized. What metrics are used to understand the business impact of real-time AI?
As scope increases, so does the need for broad alignment
The growth of real-time AI in the organization impacts execution strategy. New projects or initiatives, like adding intelligent devices for operational efficiency, improving real-time product recommendations, or opening new business models for real-time, tend to be executed at an organization’s edge—by specialized experts, evangelists, and other individuals who innovate.
The edge is away from the business center of gravity—away from entrenched interests, vested political capital, and the traditional way of thinking.
The edge has less inertia, so it’s easier to facilitate innovation, new ways of thinking, and approaches that are novel compared to an organization’s traditional lines of business, institutional thinking, and existing infrastructure. Business transformation occurs when innovation at the edge can move into the center lines of business such as operations, e-commerce, customer service, marketing, human resources, inventory, and shipping/receiving.
A real-time AI initiative is a science project until it demonstrates business value. Tangible business benefits such as increased revenue, reduced costs in operational efficiency and better decisioning must be shared with the business.
Expanding AI from the edge into the core business units requires continuous effort in risk and change management, demonstrating value and strategy, and strengthening the culture around data and real-time AI. One should not move AI deeper into the core of an organization without metrics and results that demonstrate business value that has been achieved through AI at the current level. Business outcomes are the currency for AI to grow in an organization.
A real-time data platform
Here we see the current state of most data ecosystems compared to the real-time data stack necessary to drive real-time AI success:
DataStax
Leaders face challenges in executing a unified and shared vision across these environments. Real-time data doesn’t exist in silos; it flows in two directions across a data ecosystem. The data used to train ML models may exist in memory caches, the operational data store, or in the analytic databases. Data must get back to the source to provide instructions to devices or to provide recommendations to a mobile app. A unified data ecosystem enables this in real time.
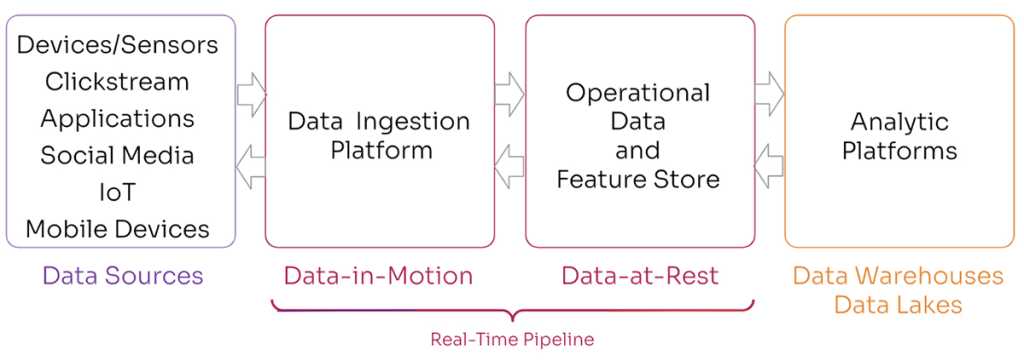
DataStax
Within the real-time data ecosystem, the heart of real-time decisioning is made up of the real-time streaming data, the ML feature store, and the ML feature engine. Reducing complexity here is critical.
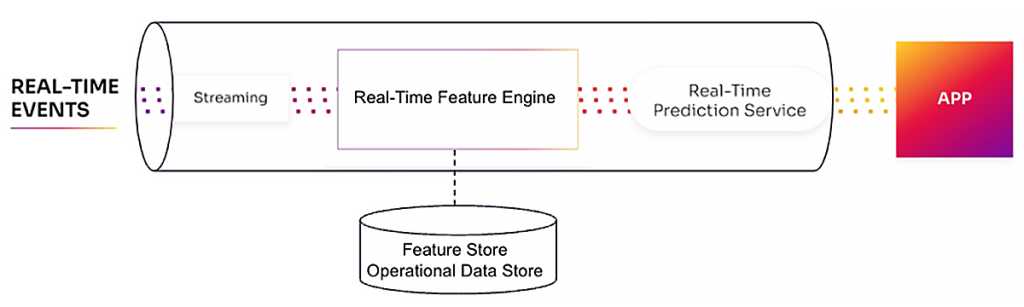
DataStax
I’ve highlighted how data for real-time decisioning flows in both directions across data sources, streaming data, databases, analytic data platforms, and the cloud. Machine-learning features contain data used to train machine-learning models and to be used as inference data when the models run in production. Real-time models that make decisions in real-time require an ecosystem that supports speed and agility for updating existing models and putting new models into production across the data dimensions shown below.
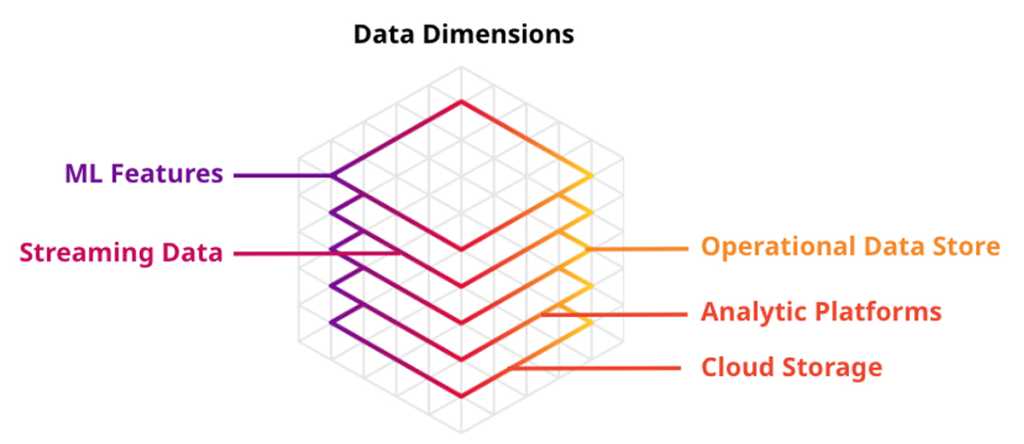
DataStax
A real-time data ecosystem includes two core components: the data ingestion platform that receives real-time messages and event streams, and the operational data store that integrates and persists the real-time events, operational data, and the machine learning feature data. These two foundational cores need to be aligned for agility across the edge, on-premises, hybrid cloud, and multi-vendor clouds.
Complexity from disparate data platforms will not support the speed and agility that data needs to work at to support real-time AI. Changing criteria, new data, and evolving customer conditions can cause machine learning models to get out of date quickly. The data pipeline flows across memory caches, dashboards, event streams, databases, and analytical platforms that have to be updated, changed, or infused with new data criteria. Complexity across the data ecosystem impacts the speed to perform these updates accurately.
A unified, multi-purpose data ingestion platform and operational data store greatly reduce the number of technology languages teams must speak and the complexity of working with real-time data flows across the ecosystem. A unified stack also improves the ability to scale real-time AI across an organization. As mentioned earlier, reducing complexity also improves the cohesiveness of the different teams supporting the real-time data ecosystem.
New real-time AI initiatives need to look at the right data technology stack through the lens of what it takes to support evolving machine learning models running in real-time. This doesn’t necessarily require ripping and replacing existing systems. Minimize disruption by running new data through an updated, agile, real-time data ecosystem and slowly migrate out of data platforms to the real-time AI stack as needed.
Wrapping up
Moving real-time AI from the edge of innovation to the center of the business will be one of the biggest challenges for organizations in 2023. A shared vision, driven by leadership and a unified real-time data stack, are key factors for enabling innovation with real-time AI. Growing a community around innovation with real-time AI makes the whole stronger than the parts–and is the only way that AI can bring tangible business results.