- Schneider Electric ousts CEO over strategic differences
- Pakistani Hackers Targeted High-Profile Indian Entities
- The 2-in-1 laptop I recommend most is not a Dell or Lenovo (and it's $200 off)
- Los CIO buscan perfeccionar la gobernanza de la IA a pesar de las dudas
- Chinese Air Fryers May Be Spying on Consumers, Which? Warns
Understanding AI’s Role in Cybersecurity Beyond the Hype
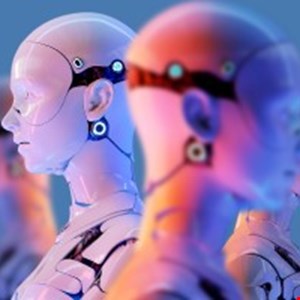
AI has enormous potential benefits in cybersecurity, including identifying threats in a network or system early, phishing attack prevention and offensive cybersecurity applications. It is also hoped these technologies will help reduce the cyber-skills gap by reducing workloads on security teams.
However, the term ‘AI’ has often become something of a buzzword in recent years, and many product vendors and organizations misunderstand or misrepresent their use of the technology.
Speaking on day one of the RSA 2023 Conference, Diana Kelley, CSO at Cyberize, said that it is important to evaluate the role of these technologies accurately, as it can lead to unrealistic expectations that have potentially “serious consequences,” including in cybersecurity.
“The reason we have to separate hype from reality is because we trust these systems,” she noted.
Kelley observed that the capabilities of AI generally have been overhyped. For example, the development of fully self-driving cars has proven a much harder challenge than previously anticipated. Fears about AI’s potentially dystopian uses are “technically possible” but certainly not for the foreseeable future Kelley noted.
She added that the abilities of AI are commonly over-estimated. Kelley highlighted a question she asked ChatGPT about which cybersecurity books she had authored – it responded with five books, none of which she had contributed to.
Nevertheless, AI technologies are playing an increasingly crucial role cybersecurity – largely in “reasoning over activity data and logs looking for anomalies” so far.
Understanding AI
For organizations to utilize AI effectively, they need to understand the different forms of AI and how they should be used. Then, they can ask the right questions of vendors, to understand if they need the ‘AI’ technology being offered.
AI covers a broad range of technologies, and their differences must be understood. For example, machine learning is a subset of AI and has very different roles and capabilities compared to generative AI systems such as ChatGPT.
Kelley said it is important to recognize that generative AI systems like ChatGPT responses are probabilities based on the data it is trained on. This is why Chat GPT got the question about her books so wrong. “There was a high probability I wrote those books,” she commented.
ChatGPT, which has been trained on information throughout the entire internet, will make a lot of mistakes “as there is a lot wrong on the internet.”
There are also significant variations in how different generative AI models operate, and their uses.
There are unsupervised learning models, in which algorithms discover patterns and anomalies without human interventions. These models have a role in discovering patterns “that humans can’t see.” In cybersecurity, this includes finding an association with a form of malware and a particular threat actor, and the users who are most likely to click on a phishing link – e.g. those who reuse passwords.
However, unsupervised AI models have drawbacks as its output is based on probability. There are issues “when being wrong has a very high impact.” This could include overreacting when malware is detected and shutting an entire system down.
Supervised learning aims to train AI models with labelled datasets to predict outcomes accurately. This makes it useful in making predictions and classifications based on known information – such as whether an email is legitimate or phishing. However, supervised learning requires lots of resources and continuous updating to ensure the AI has a high level of accuracy.
Kelley also highlighted a number of intentional and unintentional cyber risks with AI. Intentional include the creation of malware and unintentional data biases from the data it is trained on.
Therefore, it is important organizations understand these issues and ask appropriate questions of cybersecurity vendors who are offering AI-based solutions.
These include how the AI is trained e.g., “what data sets are used” and “why are they supervised or unsupervised.”
Organizations should also ensure vendors have built in resiliency into their systems to prevent intentional and unintentional problems occurring. For example, do they have a secure software development life cycle (SSDLC) in place.
Finally, it is vital to scrutinize whether the benefits of the AI provide true return on investment. “You are best placed to assess this,” said Kelley.
She added that using data scientists and platforms such as MLCommons can help make this assessment.