NIST Fortifies Chatbots and Self-Driving Cars Against Digital Threats
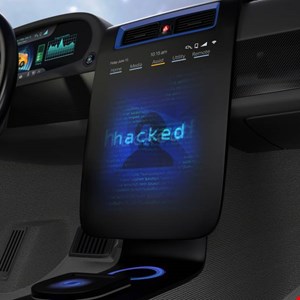
In a landmark move, the US National Institute of Standards and Technology (NIST) has taken a new step in developing strategies to fight against cyber-threats that target AI-powered chatbots and self-driving cars.
The Institute released a new paper on January 4, 2024, in which it established a standardized approach to characterizing and defending against cyber-attacks on AI.
The paper, called Adversarial Machine Learning: A Taxonomy and Terminology of Attacks and Mitigations, was written in collaboration with academia and industry. It documents the different types of adversarial machine learning (AML) attacks and some mitigation techniques.
In its taxonomy, NIST broke down AML attacks into two categories:
- Attacks targeting ‘predictive AI’ systems
- Attacks targeting ‘generative AI’ systems
What NIST calls ‘predictive AI’ refers to a broad understanding of AI and machine learning systems that predict behaviors and phenomena. An example of such systems can be found in computer vision devices or self-driving cars.
‘Generative AI,’ in NIST taxonomy, is a sub-category within ‘predictive AI,’ which includes generative adversarial networks, generative pre-trained transformers and diffusion models.
“While many attack types in the PredAI taxonomy apply to GenAI […], a substantial body of recent work on the security of GenAI merits particular focus on novel security violations,” reads the paper.
Evasion, Poisoning and Privacy Attacks
For ‘predictive AI’ systems, the report considers three types of attacks:
- Evasion attacks, in which the adversary’s goal is to generate adversarial examples, which are defined as testing samples whose classification can be changed at a deployment time to an arbitrary class of the attacker’s choice with only minimal perturbation
- Poisoning attacks, referring to adversarial attacks conducted during the training stage of the AI algorithm
- Privacy attacks, attempts to learn sensitive information about the AI or the data it was trained on in order to misuse it
Alina Oprea, a professor at Northeastern University and one of the paper’s co-authors, commented in a public statement: “Most of these attacks are fairly easy to mount and require minimum knowledge of the AI system and limited adversarial capabilities. Poisoning attacks, for example, can be mounted by controlling a few dozen training samples, which would be a very small percentage of the entire training set.”
Abusing Generative AI Systems
AML attacks targeting ‘generative AI’ systems fall under a fourth category, which NIST calls abuse attacks. They involve the insertion of incorrect information into a source, such as a webpage or online document, that an AI then absorbs.
Unlike poisoning attacks, abuse attacks attempt to give the AI incorrect pieces of information from a legitimate but compromised source to repurpose the AI system’s intended use.
Some of the mentioned abuse attacks include:
- AI supply chain attacks
- Direct prompt injection attacks
- Indirect prompt injection attacks
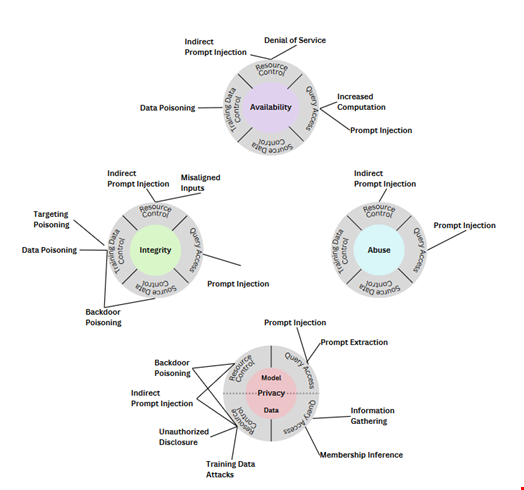
Need for More Comprehensive Mitigation Strategies
The authors provided some mitigation techniques and approaches for each of these categories and sub-categories of attacks.
However, Apostol Vassilev, a computer scientist at NIST and one of the co-authors, admitted that they are still largely insufficient.
“Despite the significant progress AI and machine learning have made, these technologies are vulnerable to attacks that can cause spectacular failures with dire consequences. There are theoretical problems with securing AI algorithms that simply haven’t been solved yet. If anyone says differently, they are selling snake oil,” he said.
“We […] describe current mitigation strategies reported in the literature, but these available defenses currently lack robust assurances that they fully mitigate the risks. We are encouraging the community to come up with better defenses.”
NIST’s Effort to Support the Development of Trustworthy AI
The publication of this paper comes three months after the release of Joe Biden’s Executive Order on Safe, Secure, and Trustworthy Development and Use of Artificial Intelligence (EO 14110). The EO tasked NIST to support the development of trustworthy AI.
The taxonomy introduced in the NIST paper will also serve as a basis to put into practice NIST’s AI Risk Management Framework, which was first released in January 2023.
In November 2023 at the UK’s AI Safety Summit, US Vice-President Kamala Harris announced the creation of a new entity within NIST, the US AI Safety Institute.
The Institute’s mission is to facilitate the development of standards for the safety, security, and testing of AI models, develop standards for authenticating AI-generated content, and provide testing environments for researchers to evaluate emerging AI risks and address known impacts.
The UK also inaugurated its own AI Safety Institute during the summit.
Read more: AI Safety Summit: OWASP Urges Governments to Agree on AI Security Standards
