- Arm backs big data center ambitions with migration tools
- Get 10% off TurboTax to save on filing your taxes
- Help Us Keep You in the AI Driver’s Seat
- This simple laptop accessory is my secret weapon against desk clutter (and it's on sale)
- Polar adds personalized fitness subscription to its smartwatch app, like Garmin's
Rhadamanthys Malware Deployed By TA547 Against German Targets
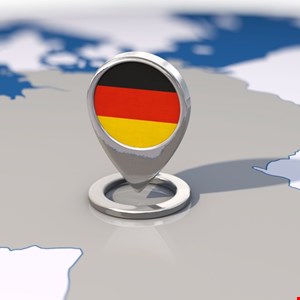
The threat actor TA547 has been observed targeting German organizations with the known stealer Rhadamanthys.
According to a recent report from Proofpoint, this is the first time this threat actor has been associated with such activity.
What’s particularly intriguing according to the researchers is the actor’s apparent employment of a PowerShell script likely generated by large language models (LLMs) such as ChatGPT, Gemini or CoPilot.
Impersonating the well-known German retail company Metro, TA547 dispatched emails relating to invoices. These emails, sent to numerous organizations across different industries in Germany, contained a password-protected ZIP file harboring an LNK file.
Upon execution, this LNK file triggered PowerShell to initiate a remote script, ultimately loading and executing the Rhadamanthys malware directly into system memory, bypassing the need for writing to disk.
Notably, the PowerShell script exhibited characteristics uncommon in typical threat actor or legitimate programmer code, indicating possible LLM involvement. Such factors included grammatically correct and hyper-specific comments above each script component, a hallmark of LLM-generated content.
This campaign showcases TA547’s strategic shift, including the adoption of compressed LNKs and the introduction of Rhadamanthys. It also underscores how threat actors leverage suspected LLM-generated content in their malicious endeavors.
According to Proofpoint, however, while threat actors can use LLMs to help understand complex attack chains and potentially enhance their campaigns, this doesn’t alter malware’s functionality or efficacy. In fact, the company believes that most behavior-based detection mechanisms remain effective regardless of the origin of malicious software.
“In the same way LLM-generated phishing emails to conduct business email compromise (BEC) use the same characteristics of human-generated content and are caught by automated detections, malware or scripts that incorporate machine-generated code will still run the same way in a sandbox (or on a host), triggering the same automated defenses,” the company explained.