- Trade in your phone to Verizon and get almost any new phone for free - here's how
- Beyond Recognition: Celebrating Cisco's Women Channel Leaders in 2025
- AMD, Nvidia partner with Saudi startup to build multi-billion dollar AI service centers
- This $95 Lenovo tablet is fantastic for mobile entertainment - and I'm tempted to buy two
- 6 trends that will shape the future of the cloud: Gartner
A Look Behind the Glass: How AI Infrastructure Can Empower Our National Labs
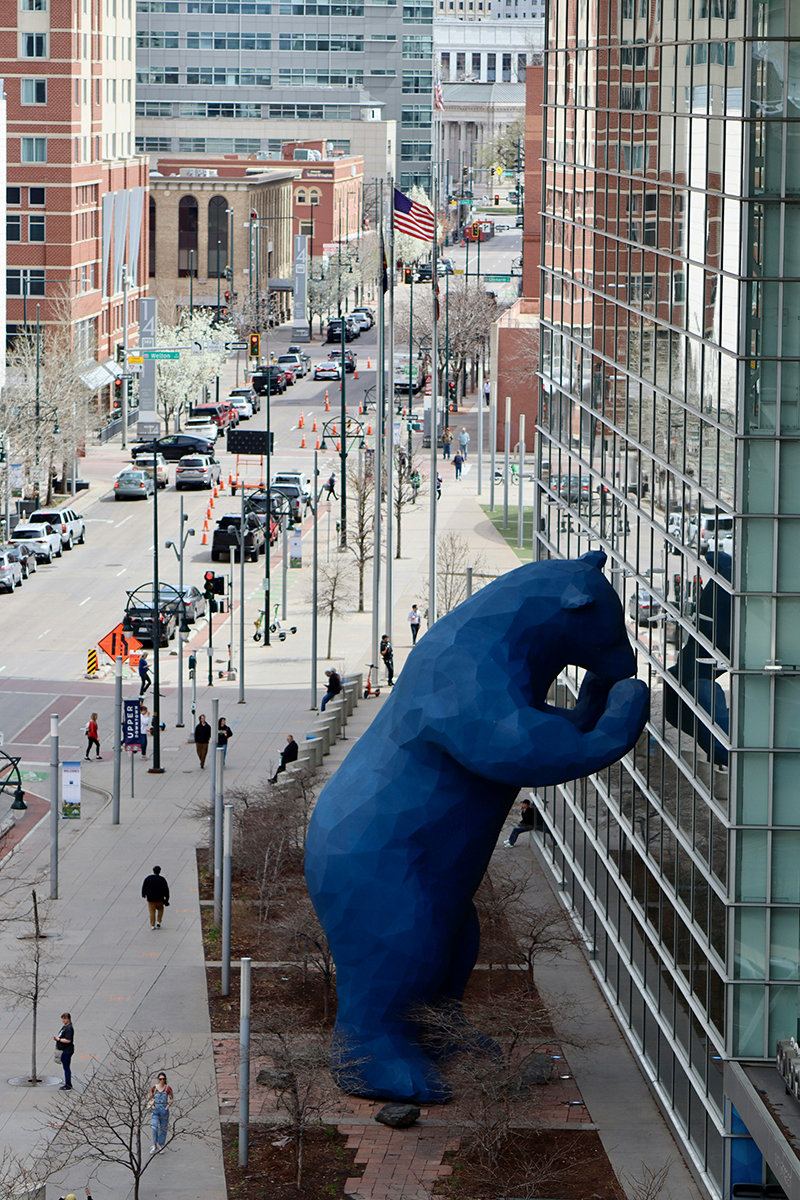
When you walk up to the Denver Convention Center, it’s impossible to miss the giant, blue 40-foot bear peering through the glass. Officially titled “I See What You Mean” by artist Lawrence Argent, the sculpture is a symbol of curiosity and wonderment. It was inspired by a photo of a bear looking into someone’s window during a Colorado drought, and Argent’s creation captures the interest the public has around “the exchange of information, ideas, and ideologies” during events like this year’s National Laboratory Information Technology (NLIT) Summit, held May 5-8, 2025 (source).
Inside the convention center, that same spirit of curiosity was alive and well as hundreds of attendees from across the DOE National Laboratories gathered to exchange new learnings and innovations. This year, one of the most heavily discussed topics was AI infrastructure—a subject as vast and complex as the research it powers. In this post, I’ll take you behind the glass for a closer look at the conversations, challenges, and opportunities surrounding AI in our national labs.
Setting the Scene: What Is NLIT and Why Does It Matter?
The NLIT Summit is a cornerstone event for the Department of Energy’s (DOE) National Laboratories, where experts come together to discuss the IT and cybersecurity operations that underpin some of the most important research in the world. The DOE’s 17 labs—one example being the Lawrence Livermore National Laboratory (LLNL)—tackle challenges ranging from clean energy innovation to climate modeling, national security, and healthcare advancements. They even use massive laser arrays to create tiny stars right here on earth; see the excellent – dare I say illuminating? – works of the National Ignition Facility (NIF) at LLNL.
At the heart of their work, like so many scientific labs, lies data—massive amounts of it. Managing, securing, and extracting insights from this data is no small task, and that’s where AI infrastructure comes into play. Simply put, AI infrastructure refers to the hardware, software, and tools required to develop and run artificial intelligence models. These models can be built in-house, such as custom large language models (LLMs), or pulled from existing platforms like GPT-4 or Llama. And while the potential is enormous, so are the logistical and operational challenges.
AI in Action: A Vision of What’s Possible
AI’s applications span a wide range, one example being complex data analysis that drives scientific discovery. The ability to run AI models locally or natively on high-performance computing systems gives labs the power to process data faster, make predictions, and uncover patterns that were previously invisible.
AI can also be used in institutional tooling that automates day-to-day operations. Imagine this: A national lab uses AI to optimize HVAC systems, reducing energy consumption while keeping labs running smoothly. Contractors are managed more efficiently, with AI optimizing schedules and spotting potential issues early. Decision-making becomes more informed, as AI analyzes data and predicts outcomes to guide big decisions.
In this future, AI isn’t just a tool—it’s a partner that helps labs tackle all kinds of research challenges. But getting there isn’t as simple as flipping a switch.
The Reality Check: Implementation Challenges
While the vision of AI-empowered laboratories is exciting, there’s a rubber meets the road moment when it comes to implementation. The reality is that building and maintaining AI infrastructure is complex and comes with significant hurdles.
Here are some of the biggest challenges raised during NLIT 2025, along with how they can be addressed:
1. Data Governance
- The Challenge: National laboratories in the Department of Energy rely on precise, reliable, and often sensitive data to drive AI models that support critical research. Strong data governance is crucial for protecting against unauthorized access, breaches, and misuse in areas like nuclear research and energy infrastructure.
- Solution: Implement data governance for workloads from ground to cloud. Some example steps: Use a CNI (Container Network Interface) like eBPF-powered Cilium to monitor and enforce data flows to ensure compliance, and establish anomaly detection with real-time automated response (see tools like AI Defense).
2. Observability and Policy Enforcement
- The Challenge: AI systems are attractive targets for cyberattacks. Protecting sensitive research data and ensuring compliance with security policies is a top priority.
- Solution: Adopting observability tools (like those provided by Cisco and Splunk) ensures that systems are monitored for vulnerabilities, while advanced encryption protects data in transit and at rest. Apply granular segmentation and least-privilege access controls across workloads.
3. Data Egress from Private Sources
- The Challenge: Moving data out of private, secure environments to train AI models increases the risk of breaches or unauthorized access.
- Solution: Minimize data movement by processing it locally or using secure transfer protocols. Identify unauthorized egress of sensitive or controlled information. AI infrastructure must include robust monitoring tools to detect and prevent unauthorized data egress.
Bridging the Gap: Turning Vision into Reality
The good news is that these challenges are solvable. At NLIT, there was a strong focus on pragmatic conversations—the kind that bridge the gap between executive visions for AI and the technical realities faced by the teams implementing it. This collaborative spirit is essential because the stakes are high: AI has the potential to revolutionize not only how labs operate but also the impact their research has on the world. Cisco’s focus on AI-powered digital resilience is well-suited to the unique challenges faced by national labs. By pushing security closer to the workload and leveraging hardware acceleration capabilities from SmartNICs to NVIDIA DPU’s, combined with Splunk observability, labs can address key priorities such as protecting sensitive research, ensuring compliance with strict data regulations, and driving operational efficiency. This partnership enables labs to build AI infrastructure that is secure, reliable, and optimized to support their critical scientific missions and groundbreaking discoveries.
Peering Into the Future
Just like the giant blue bear at the Denver Convention Center, we’re peering into a future shaped by AI infrastructure. The curiosity driving these conversations at NLIT 2025 pushes us to ask: how do we practically and responsibly implement these tools to empower groundbreaking research? The answers may not be simple, but with collaboration and innovation, we’re moving closer to making that future a reality.
Share: