Achieving Reliable AI Models for Network Performance Assurance
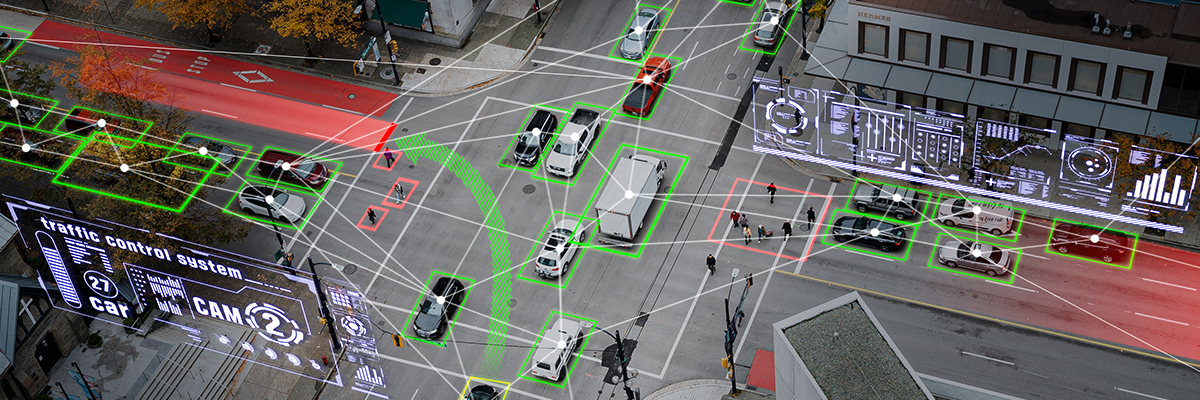
AI models are only as good as the data they are trained on. For AI models to be truly useful, they require accuracy, granularity, and diverse sources of data—like how the quality of a photograph depends on sharpness and clarity, level of detail, and the information it conveys.
- Accuracy is how true to life an image is—if it’s blurry or distorted, the details are misleading.
- Granularity is the level of detail captured—zoomed out, you get a broad overview; but zoomed in, you see the fine textures and nuances.
- Diversity relates to the composition of an image—is there enough distinctiveness in the contents of the image for the viewer to understand the story the image is trying to tell?
For network operators to rely on AI models to help them determine the root cause of network issues and predict failures before they occur, those models depend on accurate, granular, and diverse sources of network performance data. When done right, AI models can enable the necessary insight to move towards truly autonomous networks.
Strengthen AI-ready network assurance
Let’s break down how data granularity, accuracy, and diversity apply to network performance and how they translate into assuring network quality by being predictive, rather than reactive.
1. Data granularity: Seeing the finer details of network behavior
Imagine monitoring a network with an AI model that is trained on data samples taken at a rate of just one second per minute. During the remaining 59 seconds of that minute, spikes in latency, brief outages, or bursts of congestion could be missed entirely.
Without access to granular data, AI models can fail to detect critical events and lack useful context. This leads to an inability to do root cause analysis and produces incorrect predictions from AI models.
On the other hand, a network model trained on highly detailed data down to millisecond levels can identify network behaviors that impact customer experience. This granular level of detail allows AI to interpret nuanced changes, like the early signs of network congestion or packet loss. Fine-grained data in network performance AI model training enables the model to detect small but significant patterns that help anticipate, identify, and solve issues before they escalate.
2. Data accuracy: Ensuring trustworthy and reliable monitoring
While data granularity and volume are critical for high-performance models, so is accuracy of that data. When looking at a high-performance network—whether it’s a 5G fronthaul link, a high-frequency financial trading network, or a manufacturing IoT network—microseconds count. Low-accuracy data can introduce bias or errors in AI models, leading to incorrect predictions and risky conclusions about the root cause of network issues.
A wrong prediction or faulty conclusion can result in unplanned downtime. One European automotive manufacturer reports that unplanned downtown cost the company approximately US $2.3 million per hour.
Highly accurate data helps ensure the AI model understands the temporal relationship between issues happening in the network. High-accuracy data enhances the reliability of the AI model’s performance monitoring and strengthens network assurance.
3. Data diversity: Learning from a wide range of network scenarios
When it comes to training AI, diverse data sets generally result in more accurate outputs. Network complexity means packets are treated differently—packet sizes, VLAN tags, DSCP markings, hashing across ECMP, and so on, can all impact performance in various ways.
Diverse sources of data ensure that measurements are being conducted across a wide range of possible factors that can impact network performance, ensuring that AI models take every relevant factor into account.
A diversity of data sources also enables AI models to get a fuller picture of all types of traffic on the network, leading to outputs that have increased confidence in the root cause of issues in the network.
Move toward fully autonomous networks
Data granularity, accuracy, and diversity help ensure AI models have the foundation to interpret complex, disparate, and unpredictable situations that can arise in network performance. Reliable network performance data and accurate predictive insight from AI models enable the root cause analysis and predictive insights that allow organizations to move from reactive to predictive network assurance to fully autonomous networks.
Autonomous networks play a critical role in expanding networks without also significantly increasing operational costs. With reliable AI models driving autonomous networks, organizations can avoid hidden performance issues that cause application delays and impact productivity, while eliminating unplanned downtime that impacts the bottom line.
Share: