- LG vs Samsung TV: Which brand should you buy in 2025?
- Amazon credits analog with making Ocelot a more-efficient quantum chip
- This Samsung Galaxy S25 Ultra deal on Amazon gets you a $200 gift card for free
- How TikTok's enhanced desktop app challenges YouTube in game streaming - and more
- The Sandisk Extreme Pro 8TB Portable SSD is $210 off at Amazon
AI Chatbots Highly Vulnerable to Jailbreaks, UK Researchers Find
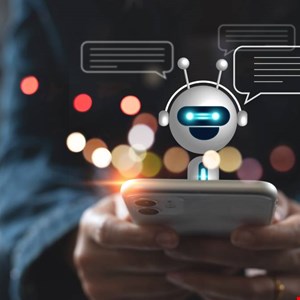
Four of the most used generative AI chatbots are highly vulnerable to basic jailbreak attempts, researchers from the UK AI Safety Institute (AISI) found.
In a May 2024 update published ahead of the AI Seoul Summit 2024, co-hosted by the UK and South Korea on 21-22 May, the UK AISI shared the results of a series of tests performed on five leading AI chatbots.
The five generative AI models are anonymized in the report. They are referred to as the Red, Purple, Green, Blue and Yellow models.
The UK AISI performed a series of tests to assess cyber risks associated with these models.
These included:
- Tests to assess whether they are vulnerable to jailbreaks, actions designed to bypass safety measures and get the model to do things it is not supposed to
- Tests to assess whether they could be used to facilitate cyber-attacks
- Tests to assess whether they are capable of autonomously taking sequences of actions (operating as “agents”) in ways that might be difficult for humans to control
The AISI researchers also tested the models to estimate whether they could provide expert-level knowledge in chemistry and biology that could be used for positive and harmful purposes.
Bypassing LLM Safeguards in 90%-100% of Cases
The UK AISI tested four of the five large language models (LLMs) against jailbreak attacks.
All proved to be highly vulnerable to basic jailbreak techniques, with the models actioning harmful responses in between 90% and 100% of cases when the researchers performed the same attack patterns five times in a row.
The researchers tested the LLMs using two types of question sets, one based on HarmBench Standard Behaviors, a publicly available benchmark, and the other developed in-house.
To grade compliance, they used an automated grader model based on a previous scientific paper combined with human expert grading.
They also compared the results to LLM outputs when asked sets of benign and harmful questions without using attack patterns.
The researchers concluded that all four models comply with harmful questions across multiple datasets under relatively simple attacks, even if they are less likely to do so in the absence of an attack.
LLMs are Limited Tools for Cyber-Attackers
Other tests shared in the UK AISI May 2024 update showed that four publicly available can solve simple capture the flag (CTF) challenges, of the sort aimed at high school students.
However, they all struggled with more complex problems, such as university-level cybersecurity challenges.
Finally, the UK AISI researchers showed that two models could autonomously solve some short-horizon tasks, such as software engineering problems, but none is currently able to plan and execute sequences of actions for more complex tasks.
These findings suggest that LLMs are likely not significantly helpful tools for cyber-attackers.