AI/ML Digital Everest: Dodging System Failure Summit Fever
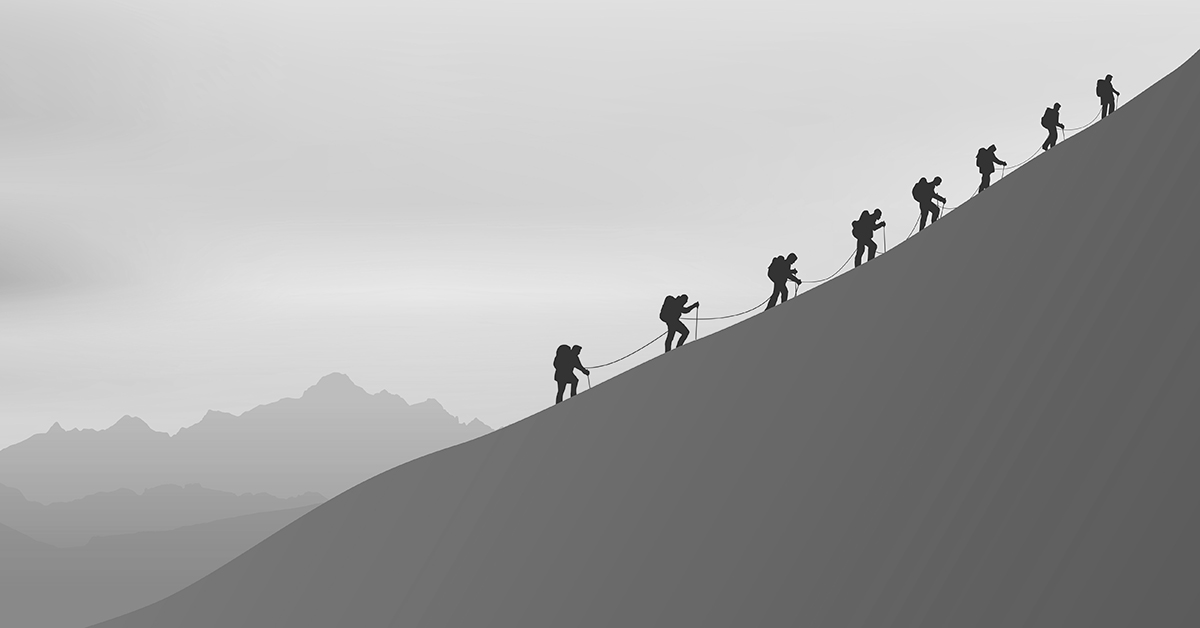
Summit Fever Syndrome, a cause of many extreme altitude climbers’ deaths, is due to a lack of oxygen and mission blindness, which leads to impaired judgment where climbers take needless risks, disregard safety precautions, and make deadly errors.
Deploying AI/ML models is like climbing Mount Everest. Both climbers and AI projects chase their peaks with (sometimes too much ) determination and succumb to “Summit Fever.” This obsession, much like climbers ignoring the perilous signs on Everest, can doom AI initiatives through mission blindness, poor planning, and rushing forward, ignoring risks.
All climbers or organizations can suffer fatal errors, but understanding why overly ambitious efforts fail and using a guide can help a climber or project team minimize the mistakes.
- Mission Blindness.
- Not Adjusting Reserves to Planning.
- Misplaced Haste.
- Preventing AI/ML Summit Fever.
- The Checklist Guide for Your AI/ML LLM Journey.
Mission Blindness
The drive to complete a mission can induce people to ignore warning signs. In mountain climbing, the weather can be one of the most hazardous forces. Climbers who ignore forecasts or push ahead despite bad weather often find themselves in dangerous situations. Similarly, in AI/ML, data is the weather system. Deploying models without scrutinizing the quality, relevance, and bias in your data is like climbing Everest in a storm. Poor data quality can lead your AI/ML projects into a whiteout, where visibility is zero, and the path forward is obscured.
Not Adjusting Reserves to Planning
On Everest, every extra kilogram in a climber’s pack can be a liability, draining energy and making the ascent more dangerous. In the AI/ML realm, this is similar to overfitting your model. Just as climbers must balance their load to carry only what is essential, AI/ML practitioners must trim their models to generalize well so they can provide useful responses. Overfitting is like carrying too much gear; it may make you feel prepared, but it ultimately hampers your ability to reach new heights and adapt to the terrain ahead.
Misplaced Haste
Acclimatization is crucial in high-altitude climbing; it allows the body to adjust to lower oxygen levels, reducing the risk of altitude sickness. In AI/ML, validation plays a similar role. Skipping the step of validating your models on a separate dataset is like heading to the summit without acclimatizing. It may lead to models that perform well in a controlled environment but fail miserably in the real world, where conditions are far more variable and less forgiving.
Navigating the ascent of AI/ML deployment demands respect for the terrain and an understanding that the journey is as fraught with risk as it is ripe with reward. Like Everest climbers who meticulously plan their route, assess conditions, and adjust their strategy, successful AI/ML practitioners approach their projects with a clear head, thorough preparation, and the agility to pivot when faced with unforeseen challenges.
Summit Fever Syndrome in AI/ML Deployment
Just as climbers can become so goal-oriented that they neglect safety, AI/ML teams can suffer from a similar tunnel vision. This “Summit Fever” in AI/ML projects manifests when teams become overly focused on deploying a system or achieving a result at all costs, neglecting critical signs that suggest a need to reassess the project’s viability, ethical implications, or potential biases in the model. The desire to achieve a breakthrough or launch a product can overshadow the importance of due diligence, such as thorough testing, data validation, ethical considerations, and the long-term impacts of the deployment.
Preventing AI/ML Summit Fever
- Institute Checkpoints: Just as climbers have camps to assess their readiness for the next phase, AI/ML projects should have predefined review stages. These checkpoints serve as opportunities to evaluate the project’s current state, reassess goals, and ensure that the pursuit of innovation does not compromise ethical standards or quality.
- Encourage Dissent: Climbing teams benefit from members who voice concerns about safety or conditions. Similarly, AI/ML teams should foster an environment where dissent is welcomed and considered. Encouraging team members to express doubts or concerns about the project can provide valuable insights that prevent oversight and promote a more cautious and thoughtful approach to deployment.
- Focus on Sustainable Goals: The fixation on reaching the summit at any cost can be counterproductive. In AI/ML, this means prioritizing sustainable and ethical AI practices over the rush to market. This includes acknowledging when a project may need more development time when models require further refinement, or when ethical concerns necessitate a pivot in approach.
The Checklist Guide for Your AI/ML LLM Journey
The release of ChatGPT exposed both the benefits and threats of AI/ML Large Language Models and the need for business and technology leaders to be able to quickly assess the pros, cons, and threats to their organizations from this rapidly evolving technology. The OWASP LLM AI Cybersecurity & Governance Checklist is a reliable guide and map for this journey. The checklist follows the OODA Loop decision-making framework, which helps organization leaders prioritize threats and make decisions quickly.
AI threats are organized into five categories for easier analysis and mitigation.
Each topic area includes a checklist of questions and action items for leaders to consider and address:
Summit Fever in AI/ML deployment underscores the critical balance between ambition and responsibility. Leaders and project teams can protect their AI/ML initiatives against failure-inducing blind ambition by establishing checkpoints, promoting dissent, emphasizing sustainable goals, and using resources such as the OWASP LLM AI Cybersecurity & Governance checklist to ensure their AI/ML summit journey is a successful one.
Editor’s Note: The opinions expressed in this guest author article are solely those of the contributor and do not necessarily reflect those of Tripwire.