- T-Mobile's data breach settlement payments finally rolling out this month - after April delay
- Cisco unveils prototype quantum networking chip
- What’s Next for MCP Security? | Docker
- Zero Trust: Begin your journey with these first steps
- Ready to ditch Windows for good? This is the Linux distro I recommend for beginners
AI Workloads Accelerated with Cisco UCS X-Series, Nvidia GPUs, and Cloudera CDP
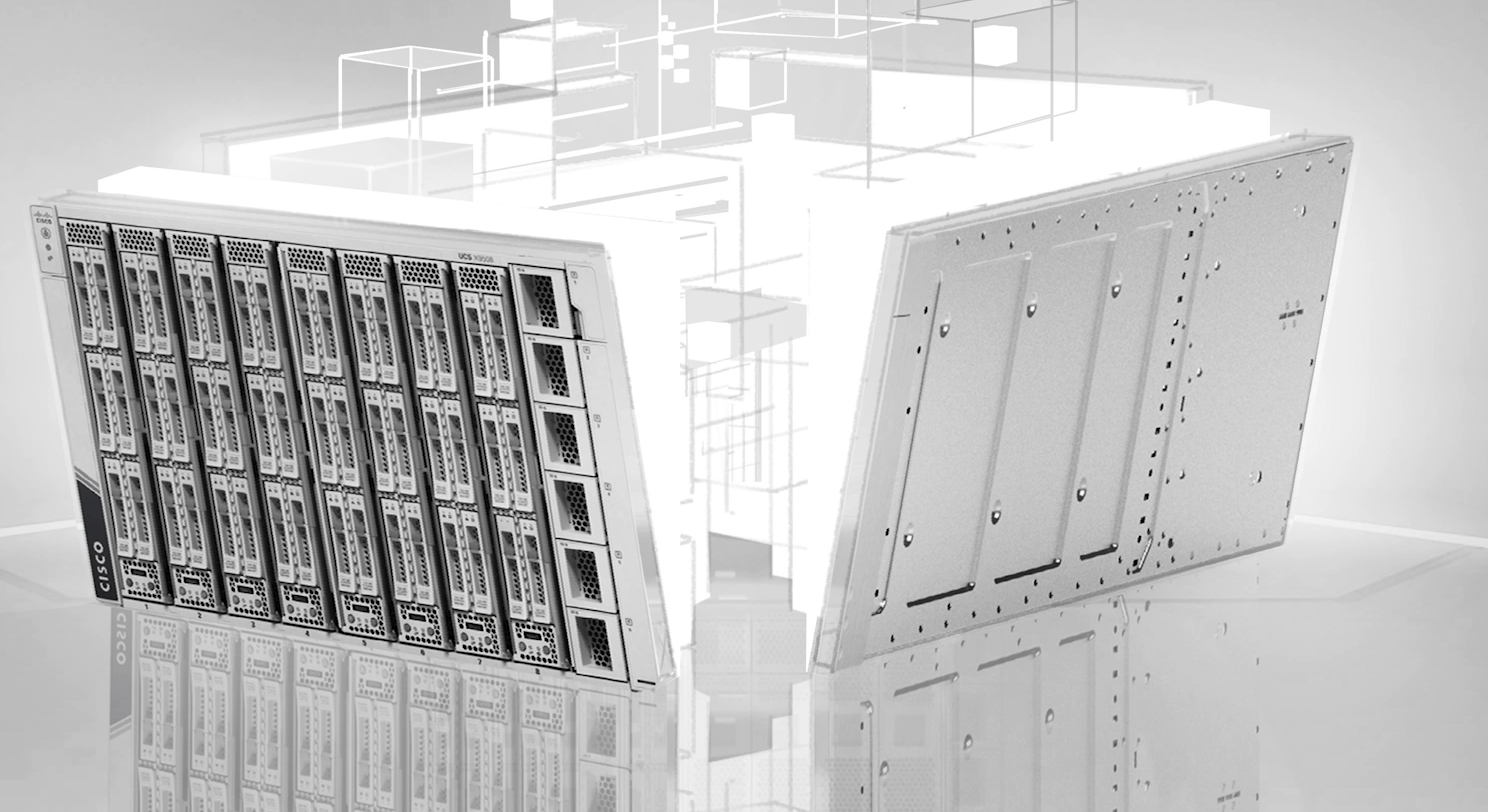
I’m turning over Authoring of this blog to Samuel Nagalingam, Product Manager & Hardik Patel, Technical Marketing Engineer, to talk about GPU accelerated big data on UCS X-Series.
Enterprises across all industries are recognizing the true potential of AI/ML. Data scientists are utilizing large data sets, implementing use cases such as transforming supply chain models, responding to increased levels of fraud, predicting customer churn, and developing new product lines, to mention a few key use cases. The global artificial intelligence software market size valued at USD $53B in 2021, is projected to grow exponentially in the coming years to reach USD $850B by 2030, growing at a CAGR of 41% from 2022 to 2030.
According to the research report by Tractica, over 330 AI use cases across 28 industries will contribute to the market growth with significant opportunities in automotive, consumer, healthcare, banking and financial, telecommunications, education, and retail and eCommerce sectors.
To address these multi-variate use cases, you need a platform with high performance, that can scale, is secure and easy to manage. In response, Cisco, Cloudera, and Nvidia have partnered to deliver hybrid and/or private cloud solution that meets all the above requirements. The architecture has the Cloudera Data Platform (CDP) software running on Cisco Data Intelligence Platform (CDIP) with Nvidia GPUs.
The Big Data architecture has evolved from a monolithic cluster of storage and compute to desegregated components of storage and compute.
- Data Lake (storage): The CDP Private Cloud Base software running on UCS X-Series Servers or Cisco UCS M6 Rack Servers provide storage and supports traditional data lake environments with Apache Ozone, the next gen file system for data lake.
- Compute Farm (analytics / AI): The CDP Private Cloud Data Services software running on Cisco UCS X-Series servers supports analytics and AI/ML workloads.
The compute farm for Analytics/AI has CDP Private Cloud Data Services deployed on Red Hat OpenShift Container platform or Cloudera Embedded Container Service, running on UCS X-Series, a future ready, modular system that meets the needs of modern cloud native applications and is managed by Cisco Intersight.
Compute, GPUs & 100G End-to End Connectivity
The modularity of X-Series makes it easy to add or upgrade individual elements like CPUs or GPUs.
The UCS X-Series X9508 Chassis with 8 slots, accommodates the UCS X210c Compute Node with third generation Intel Xeon Scalable processors and the UCS X440p PCIe Node that supports Nvidia GPUs – A16, A40, A100 & T4. The UCS X210c Compute Node together with UCS X440p PCIe Node can be paired to form a dual-wide node server with GPUs. You can further increase the GPU density per server by adding two GPUs to the X210c Compute Node.
- GPU with parallelization and acceleration can provide more than ~ 10X faster data analytics and machine learning at a lower cost.
Today, only Cisco UCS X-Series supports 100Gbps end-to-end in a modular server form factor.
- Higher bandwidth further increases the AI/ML workloads performance.
CDIP in action
In the video, we have an example of one of Cloudera CDP Data Services, Cloudera Machine Learning, which with its distributed GPU scheduling and training for model deployment, allows faster processing and extraction of insightful business analytics.
Cisco had developed Cisco Validated Designs for this architecture that will cover a broad range of workloads. They provide guidance on deploying solutions at customer site with minimal risk with 24/7 support options.
Summary
The Cisco Data Intelligence Platform with Cloudera Data Platform (CDP) running on UCS X-Series with Nvidia GPUs and 100G end-to-end connectivity has tremendous computing power, faster acceleration, and wide bandwidth needed to address the multitude of cloud native AI/ML workloads that customers are running.
Resources
Share: