- How to disable ACR on your TV (and stop companies from spying on you)
- I expected this cheap multitool to be a waste of money, but it's my new a toolbox essential
- Have The Last Word Against Ransomware with Immutable Backup
- Multi-channel Secure Communication
- Apple's bold idea for no-code apps built with Siri - hype or hope?
Arista floats its answer to the strain AI puts on networks
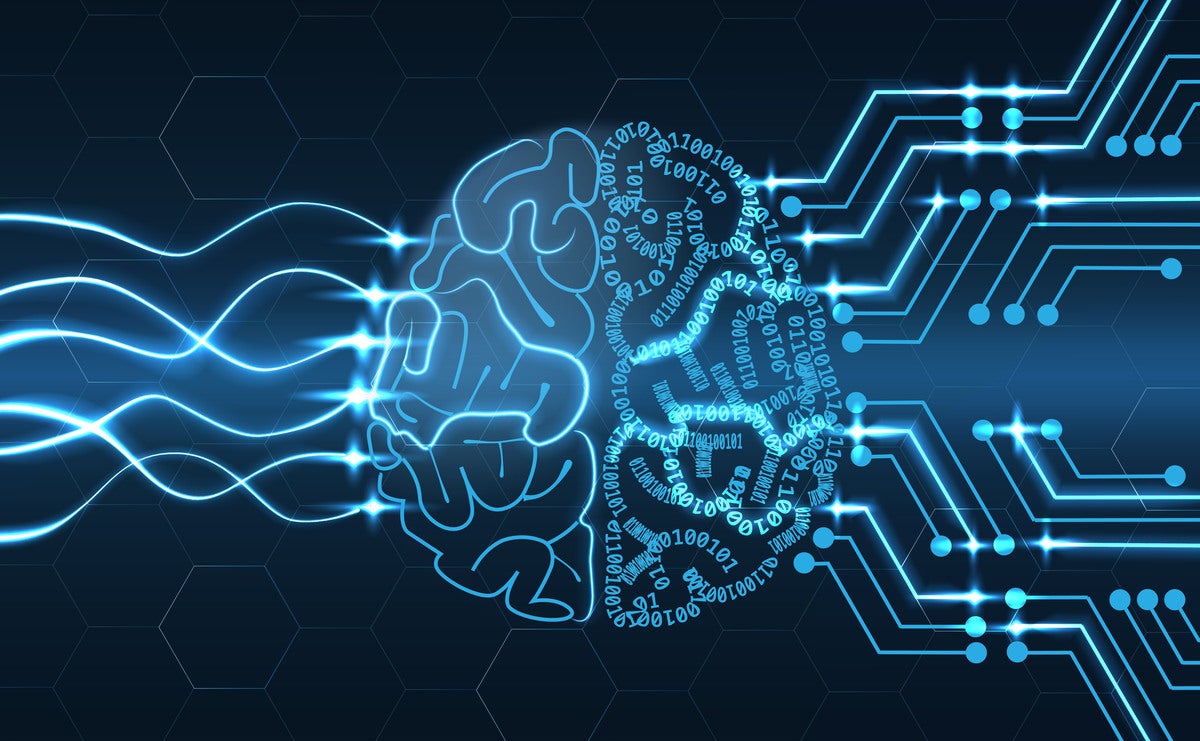
If networks are to deliver the full power of AI they will need a combination of high-performance connectivity and no packet loss
The concern is that today’s traditional network interconnects cannot provide the required scale and bandwidth to keep up with AI requests, said Martin Hull, vice president of Cloud Titans and Platform Product Management with Arista Networks. Historically, the only option to connect processor cores and memory have been proprietary interconnects such as InfiniBand, PCI Express and other protocols that connect compute clusters with offloads but for the most part that won’t work with AI and its workload requirements.
Arista AI Spine
To address these concerns, Arista is developing a technology it calls AI Spine, which calls for switches with deep packet buffers and networking software that provides real-time monitoring to manage the buffers and efficiently control traffic.
“What we are starting to see is a wave of applications based on AI, natural language, machine learning, that involve a huge ingestion of data distributed across hundreds or thousands of processors—CPUs, GPUs—all taking on that compute task, slicing it up into pieces, each processing their piece of it, and sending it back again,” Hull said.
“And if your network is guilty of dropping traffic, that means that the start of the AI workload is delayed because you’ve got to retransmit it. And if during the processing of those AI workloads, traffic is going backwards and forwards again, that slows down the AI jobs, and they may actually fail.”
AI Spine architecture
Arista’s AI Spine is based on its 7800R3 Series switches, which, at the high end supports 460Tbps of switching capacity and hundreds of 40Gbps, 50Gbps, 100Gbps, or 400Gbps interfaces along with 384GB of deep buffering.
“Deep buffers are the key to keeping the traffic moving and not dropping anything,” Hull said. “Some worry about latency with large buffers, but our analytics don’t show that happening here.”
AI Spine systems would be controlled by Arista’s core networking software, the Extensible Operating System (EOS), which enables high-bandwidth, lossless, low-latency, Ethernet-based networks that can interconnect thousands of GPUs at speeds of 100Gbps, 400Gbps, and 800Gbps along with buffer-allocation schemes, according to a white paper on AI Spine.
To help support that, the switches and EOS package creates a fabric that breaks apart packets and reformats them into uniform-sized cells, “spraying” them evenly across the fabric, according to Arista. The idea is to ensure equal access to all available paths within the fabric and zero packet loss.
“A cell-based fabric is not concerned with the front-panel connection speeds, making mixing and matching 100G, 200G, and 400G of little concern,” Arista wrote. “Moreover, the cell fabric makes it immune to the ‘flow collision’ problems of an Ethernet fabric. A distributed scheduling mechanism is used within the switch to ensure fairness for traffic flows contending for access to a congested output port.”
Because each flow uses any available path to reach its destination, the fabric is well suited to handling the “elephant flow” of heavy traffic common to AI/ML applications, and as a result, “there are no internal hot spots in the network,” Arista wrote.
AI Spine models
To explain how AI Spine would work, Arista’s white paper provides two examples.
In the first, a dedicated leaf-and-spine design with Arista 7800s tied to perhaps hundreds of server racks, EOS’s intelligent load-balancing capabilities would control the traffic among the servers to avoid collisions.
QoS classification, Explicit Congestion Notification (ECN), and Priority Flow Control (PFC) thresholds are configured on all the switches to avoid packet drops. Arista EOS’ Latency Analyzer (LANZ) determines the appropriate thresholds to avoid packet drops while keeping the throughput high and allows the network to scale while keeping latency predictive and low.
The second use case, which could scale to hundreds of endpoints, connects all the GPU modes directly into the 7800R3 switches within AI Spine. The result is a fabric providing a single hop between all endpoints, driving down latency and enables a single, large, lossless network requiring no configuration or tuning,” Arista wrote.
Challenges of networking AI
The need for the AI Spine architecture was primarily driven by technologies and applications such as server virtualization, application containerization, multi-cloud computing, Web 2.0, big data, and HPC. “To optimize and increase the performance of these new technologies, a distributed scale-out, deep-buffered IP fabric has been proven to provide consistent performance that scales to support extreme ‘East-West’ traffic patterns,” Arista wrote.
While it may be early for most enterprises to worry about handling large-scale AI cluster workloads, some larger environments as well as hyperscaler, financial, virtual reality, gaming, and automotive development networks are already gearing up for the traffic disruption they might cause on traditional networks.
As AI workloads grow they put increasing pressure on the network for scale and bandwidth, but also for the right storage and buffer depth, having predictable latency, and handling both small packets an elephant flows, Jayshree Ullal, CEO of Arista recently told a Goldman Sachs technology gathering. “This requires a tremendous amount of engineering to make traditional Ethernet run as a back-end network to support this technology for the future and the growing use of 400G is going to add additional fuel to this development,” Ullal said.
Copyright © 2023 IDG Communications, Inc.