- The 5 gadgets I can't travel without (and why they make such a big difference)
- These are the Memorial Day sales fitness enthusiasts should shop
- Samsung is giving away free 27-inch Odyssey G55C monitors - here's how to qualify
- Get a 230-piece Craftsman toolset for just $99 at Lowe's right now
- Cisco Customer Achievement Awards: Americas 2025 Finalists
Artificial Intelligence Needs People Intelligence

By Sean Foley
Enterprises yearn for the competitive advantage that ML and AI can offer their business, but often prioritize technology strategically over people to unlock the value of their data. The hype about AI and ML, and the ease of access to it through cloud tooling, belies the complexity of effectively leveraging these capabilities. Why are AI and ML critical capabilities to your business and how will pushing their introduction or expanding their use impact your data strategy?
Unfortunately, many leaders also misinterpret the desire for AI/ML capabilities as a proxy for “we need a better data strategy” and underestimate the effort required to take on this change. It’s imperative that leaders define their data ambitions clearly and align them with the business outcomes sought. This is because the key to effectively unlocking the value of your data starts with aligning your people to this business outcome-driven data strategy. Don’t get me wrong, technology is essential for a modern data strategy, but too often organizations over-rotate on technology and forget about the critical strategic and human elements.
Establishing a data strategy that successfully supports AI/ML adoption requires 3 foundational elements:
1) Data strategy aligned to business goals
Define the “Why” and the “What” first. Start by defining what concrete business goals can be achieved through their use, in what timeframe, at what cost, and at the expense of what other organizational priorities. Is your organization looking to drive down product defects, increase client satisfaction, or innovate new products? Understanding the product or service drivers of your new data strategy will highlight how your existing data strategy will need to change.
HPE
What are your business goals? Perhaps new streams of revenue or increased operational leverage? How does AI/ML adoption accelerate those goals? Or do you simply need clean data, delivered faster to your team or to a production process? Thankfully, optimized distribution of high quality data is a step on the journey to enabling AI/ML.
2) A modern data operating model
Many enterprises sit atop siloed islands of data that have grown organically over years. The processes and operating model around maintaining and consuming this data have often calcified over time if not being regularly re-assessed against business goals.
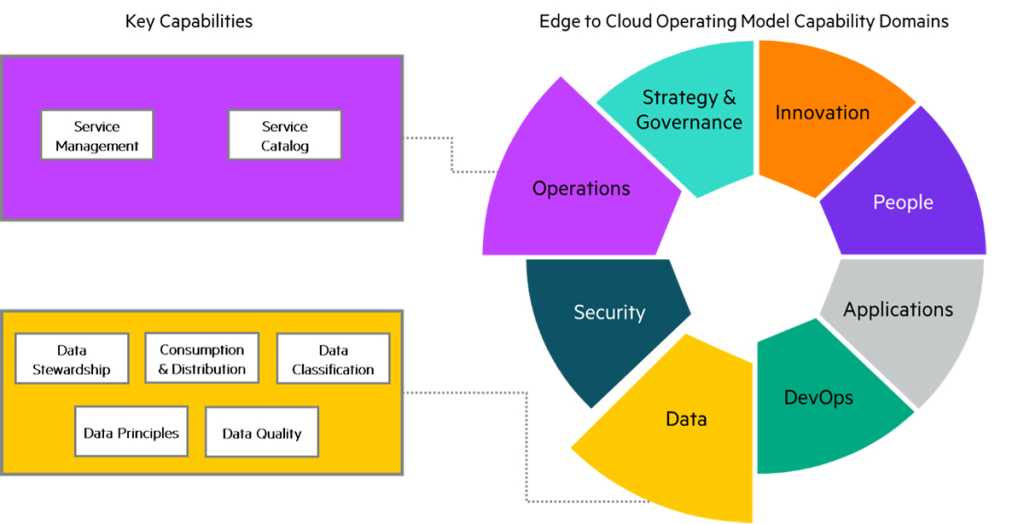
HPE
How are the fundamentals of your data operations and governance handled currently? Are service levels meeting business needs? Are they inhibiting them? Are the right data sets available where they need to be in your organization, when they need to be? How clean is your data? How fresh is it? How heavily has your organization deployed self-service for data and analytics? How well structured are the data stewardship processes? Has an AI and ML pipeline yet been created? How are they operating?
Furthermore, identify which parts of your data operating model provide a competitive advantage and which are simply undifferentiated heavy lifting. This can help uncover opportunities to leverage new platforms or 3rd party support.
3) A skill and function capacity strategy
The competition for talent is fierce so it’s essential to understand the skills you need to support your new strategy and to develop a plan for those skills to be present in your organization. It’s important to evaluate the skills you need in your organization to be able to adopt a data-first strategy which enables AI/ ML adoption? Do you have them in the organization in the depth that you need.
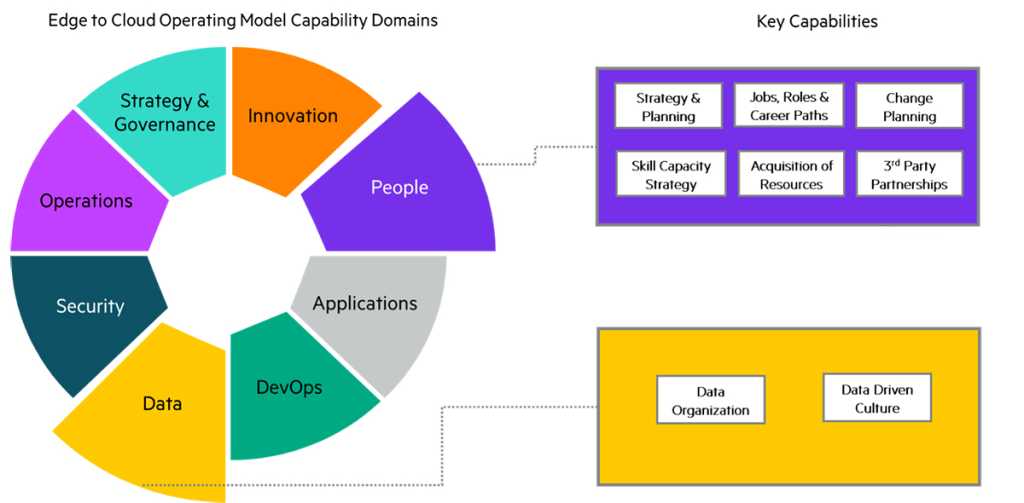
HPE
Of the major functions of an effective data operations environment, how much of this is run internally? How much of this is based on a proprietary process technology, stack, and workflow? Most importantly, are these elements providing a competitive advantage for your enterprise? Are these skills essential to driving value? Once you’ve defined which elements of your data strategy and data operations provide a competitive advantage, define a shared responsibility model for data services. This model should include skills, roles, and functions needed now, during the transition, and once the new strategy is deployed. Some roles may be eliminated, some may evolve, some will only be needed during the transition—identify all of these.
It’s a good idea to up-skill the existing team to fill critical skill gaps first to ensure the “to be” organization understands the enterprise data and how the data strategy aligns to business needs. Augment the team with new hires for the future, but only for roles that provide competitive advantage. Plan to supplement the team with 3rd party SMEs during the transition and as part of the future operating model.
The path to adopting AI/ML capabilities requires a transformation across the IT and Data ecosystems, and that journey alone will amplify value for your business at each step along the way. The approach outlined here will help set the foundations for that journey and will naturally refine the technology choices and ensure that the business strategy frames what technology is needed.
The understanding of the current and future data operating model, and the skills available and needed in your organization, will also help guide both your platform and skill-sourcing models. With these elements in hand, driving this transformation will enable insights for the business faster.
To find out more about Digital Next Advisory or engaging with an HPE Digital Advisor please contact us at digitaladvisors@hpe.com or visit www.hpe.com/digitaltransformation
____________________________________
About Sean Foley
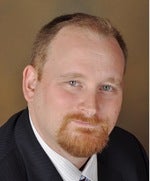