- Have The Last Word Against Ransomware with Immutable Backup
- Multi-channel Secure Communication
- Apple's bold idea for no-code apps built with Siri - hype or hope?
- The camera I recommend to most new photographers is not a Nikon or Sony
- I tested LG's new ultrathin 2-in-1, and it handles creative workloads like a dream
Balancing the Scales: Addressing Privacy, Security, and Biases in AI based on the White House Blueprint for an AI Bill of Rights
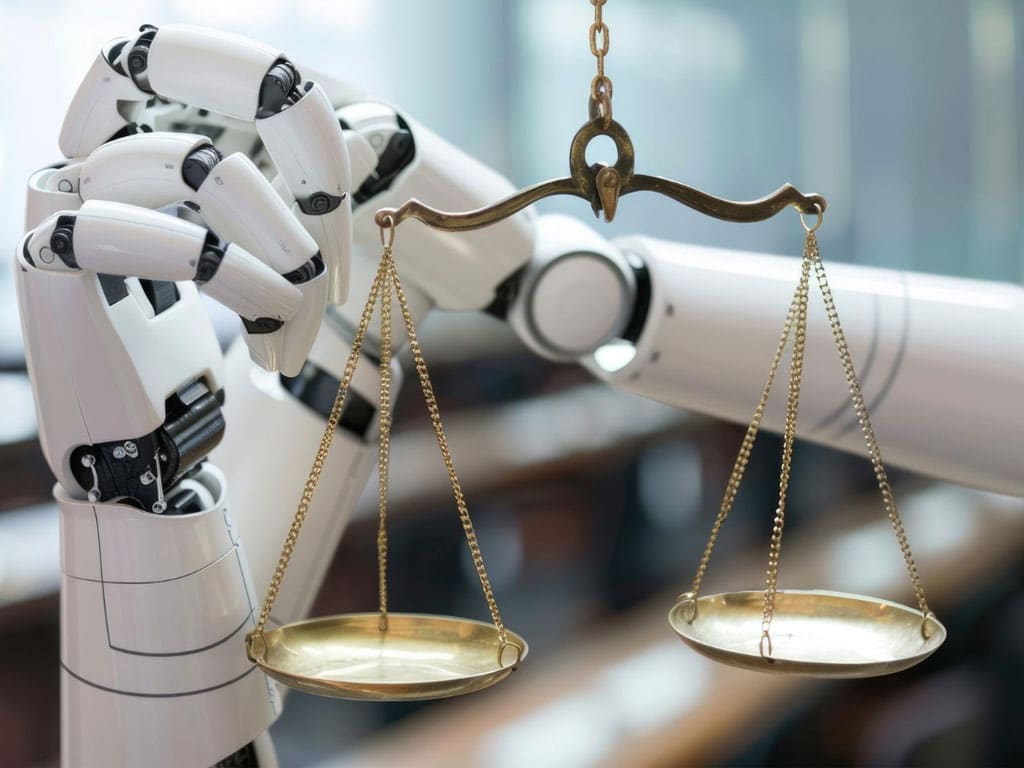
Within the last few weeks, the major AI competitors OpenAI, Google, and Microsoft unveiled several new products and capabilities of their platforms. Perhaps, most notable was OpenAI’s ability to now process speech and visual inputs in real-time. Social media was flooded with a mix of responses and many potential use cases. It’s unavoidable at this point – AI is not only here to stay, but it is already transforming many industries and beginning to be commonplace in the consumer’s everyday life.
Many CISOs are well aware that despite the gained efficiencies of AI, there are several major risks that need to be carefully examined. First, and most often cited, are the issues of privacy and security which enter a new level of importance now that these models are capturing voice and visual input and are even beginning to be worn on your person, as wearable AI seems to be next big thing. Second, and less often discussed, these algorithms can further exacerbate inequalities amongst already marginalized groups and technology illiterate. As the pace of innovation and rate of change increases, many will be left behind.
In this article I’ll attempt to tease out some of the more granular issues that are being overlooked or under-examined. As a point of reference, I will use the current White House Office of Science and Technology Policy (OSTP) Blueprint for an AI Bill of Rights and will discuss other potential measures for regulation and best practices to improve trust, transparency, safety, and accountability, while minimizing harm of AI, particularly as it relates to marginalized communities.
The AI Dilemma
To put it simply, AI relies on massive amounts of data to create statistical correlations in order to accelerate decision-making. In the context of generative AI, models can create new text, image, sound, and more based on the training data sets. These operations have risks around privacy and security, and are already grappling with generating output that may be seen as bias or discriminatory.
Privacy and Security
AI algorithms are dependent on vast amounts of personal data being collected, stored, and analyzed. Like with any technology, the potential for data breaches and unauthorized access poses severe risks. Data leaks, tampering, and downtime of essential services can have significant effects on individuals and businesses depending on the AI systems. Effective cybersecurity controls must be implemented to minimize the likelihood of exposure, misuse, and other compromise. By its nature, the complexity of AI systems often makes it challenging for users to understand how their data is being used, raising concerns regarding transparency and true informed consent. Do you know what data is being collected and how it’s being used and shared? Even if you know, can you do anything about it? Clear communication and implementing robust data privacy and security practices is critical to the effective protection of users.
Bias and Discrimination
AI algorithms depend heavily on the quality of the training data they receive and unfortunately, numerous headline-making stories have demonstrated the inherent risk of these platforms inadvertently amplifying existing biases which can lead to the unfair treatment of different groups, often those already marginalized. Gender biases in training sets can lead to unequal treatment, as shown in the well-documented case of Amazon’s recruiting tool that was trained on previous resumes, which were predominantly men’s, thus leading to the algorithm inadvertently favoring male applicants.
Leveraging biased data sets may also perpetuate systemic racism, leading to discretionary decision-making affecting equal employment opportunities, financial lending, or law enforcement. One example is demonstrated as an AI-based tool used to score the likelihood of criminal re-offense incorrectly labelled Black defendants as twice as likelihood to reoffend as white defendants. Having human intervention and fallback mechanisms are crucial in these situations before the biases are known. But that said – and knowing that nothing is ever easy – there are highly publicized instances of AI being over-corrected by manual intervention, such as with Google’s Perspective API, an AI tool developed to curb hate speech online. It faced criticism that it was overcorrecting and censoring words used in benign contexts and sparking conversations about free speech and policing AI.
Blueprint for an AI Bill of Rights
To address the aforementioned risks, many have looked to government regulations. One example is provided by the White House Office of Science and Technology Policy (OSTP) which introduced a Blueprint for an AI Bill of Rights in 2022, designed to protect civil rights of the American public as the world adapts to AI seeping into nearly every aspect of society. This framework outlines five principles:
Safe and Effective Systems
AI systems must be thoroughly vetted with rigorous testing and ongoing monitoring to ensure they are safely and effectively operating. This is achieved with pre-deployment testing, risk identification and mitigation, and continuous monitoring. Implementing safety measures with regular audits to verify system performance and reliability is key to this success. The foundational goal of protecting users from harm, along with building public trust in AI technologies is dependent on prioritizing both safety and efficacy of these systems. Transparency is paramount in the development process, so that AI systems are both technically sound and in alignment with ethical standards and user expectations.
Algorithmic Discrimination Protections
AI systems must be prevented from perpetuating existing biases and discrimination, while designed in an equitable way. Designers, developers, and deployers of AI systems must include safeguards to actively identify, address, and mitigate biases in algorithms and data sets. Leveraging diverse and representative training data helps proactively minimize the risk of discriminatory outcomes. The use of regular audits and impact assessments can aid in detecting and correcting biases in the AI decision-making processes. By implementing these protections, AI systems are better positioned to treat individuals fairly, regardless of race, gender, religion, or other protected classifications, leading to more inclusivity and effectiveness.
Data Privacy
The rights associated with strong data privacy and security protections underscore the criticality of individual control and agency over personal data. Achieving this principle involves preventing compromises around unauthorized access, misuse, and exploitation requires robust security practices to be in place, along with providing transparency about data collection practices. Individuals must be clearly informed about how their data is being used and given the opportunity to make informed choices about that collection and usage. AI systems should leverage privacy by design model with default protections in place, such as only collecting data necessary for functionality in order to support the goal of trust and transparency with AI.
Notice and Explanation
By now, the ongoing theme of transparency is clear. Understanding the operations of AI is critical for ethical and true consent. AI should not be hidden, but rather, individuals should be clearly informed when and how AI is being used with accessible, understandable, and technically valid language. By ensuring that individuals grasp AI’s involvement, logic, and decision-making processes, more trust can be established and potential issues like biases can be easier identified.
Human Alternatives, Consideration, and Fallback
While AI is advancing at an incredible rate, offering efficiencies and functionalities beyond human ability, it does not eliminate the need for maintaining human oversight. When possible, individuals should have the option for human intervention rather than depending solely on AI’s automated processes. While AI has proven powerful and often effective, it’s not at the point where it should have sole discretion over decisions that significantly impact lives, such as in healthcare, employment, or legal judgements. Fallback or escalation processes to human for consideration should be in place for system failures, errors, and for appeals of decisions made. These mechanisms are necessary for accessible, equitable, effective treatment. By preventing an over-reliance on AI and providing human touchpoints, risks can be more effectively mitigated, promoting accountability, trust, and transparency through these processes.
Regulatory Lag
While the framework above provides a solid foundation, it is simply a blueprint for the AI Bill of Rights – true regulations can take years longer. Regulatory lag is not a new concept and with the pace of AI advancements, the gap between AI, its concerns, and regulation will only grow. The EU has already published the EU Artificial Intelligence Act which, much like GDPR, is likely to set the groundwork for other regulations worldwide. With the power and trajectory of AI, perhaps it’s time for an international governance body made up of diverse stakeholders (including governments, private businesses, academics) to oversee development and deployment of AI with proactive regulation and global standards. However, an attempt to push regulation may be viewed as stifling innovation, so there would be much debate to be had.
For now, the principles in the Blueprint for an AI Bill of Rights provide a valuable framework for AI designers and developers to safeguard the personal rights of users, while promoting fairness, safety, and effectiveness of the tools. While many are focused on bringing the public the latest innovative technologies, there must be a balance with accountability and liability to comply with the basic principles of privacy, security, and fairness.
What should a CISO do?
Virtually every conference, webinar, and local cybersecurity chapter meeting that I’ve personally attended this year has echoed the same themes. CISOs are struggling with enabling the business and end users to benefit from AI tools, while mitigating potential risks. Shadow IT is nothing new. However, preventing end users from accessing AI is particularly challenging due to the ubiquitous nature of the technology. It seems like every vendor is rushing to put AI into every software, platform, and hardware device. Other CISOs are examining the problem from a data classification point-of-view: where if they can only identify their most critical data and ensure it never gets processed into an AI model, then they can reduce most harm. I’m skeptical that this will be achievable.
So, what then should a CISO do? I return to the fundamentals. The back-to-basics approach is to begin with end user security awareness and training, specifically tailored to AI. Many end users do not truly know what AI is, how it actually works, yet they are still using it daily. Educating and empowering your front-line workers to exercise caution around AI will likely yield the best results. Creating a culture where security is enabling innovation, rather than outright blocking tools via policy mandate, is essential. I’ve seen great success with my partners who institute weekly lunch-and-learn sessions specifically for AI use cases. One CISO commented to me that their attendance as dramatically increased once AI became a regular topic. There is a real demand for learning about these tools, because as I mentioned earlier, no one wants to be left behind.
Below, I will revisit the core aspects of the AI dilemma previously discussed with recommendations and best practices to alleviate the potential harm.
Privacy and Security – Revisited
Incorporating privacy and security principles right from the design phase is imperative to minimize potential compromise of data and systems with the goal of increasing trust, transparency, and safety. Consider these practices:
- Security controls: implement strong security principles (such as encryption, incident response, access control, network and endpoint security) to prevent unauthorized access, misuse of data, and tampering of data and systems.
- Audits and transparency: regular audits of AI systems for bias, safety and effectiveness, and clear privacy controls must be publicly shared for true accountability and transparency.
- Data minimization: only collect data that is necessary for functionality.
- Purpose limitation: use data only for the specified purposes.
- Data anonymization: remove identifiable information from data, however note that some Ais are able to re-anonymize de-identified data.
- Informed consent: clearly explain how data is used, if it’s being shared with third-parties, and provide updates when changes in data use and collection occur.
- Individual rights: allow users the ability to select and modify their preferences, opt-out of certain processes, and delete their data.
- Develop policies: as developers of AI, establish and enforce principles for effective security and privacy; as users of AI, establish and enforce requirements around usage of AI. Be sure to educate users on the why.
Bias and Discrimination – Revisited
Regarding bias and discrimination, the following is a non-exhaustive list of considerations to mitigate the risk of exacerbating unfair stereotypes and prejudices.
- Diverse and representative datasets: AI training data should be diverse and representative of the population to mitigate bias and discrimination.
- Inclusive design: design elements must be considered to ensure accessible and equal usability, regardless of background, identity, or physical ability.
- Community engagement and feedback: diverse communities should be consulted for input of the design and implementation to ensure unique needs and perspectives are accounted for.
- Socioeconomical impacts: consider the socioeconomic implications of AI-based automation for various tasks. While this may be an ethical question, take stock of how these systems can potentially lead to job displacement in low-income communities, widening the economic gap between groups.
- Human intervention: ensure protocols are in place for fallback or escalations to people, particularly when AI is used for significant life-changing decisions.
Just like in aspects of cybersecurity where the advancement of technology leads to both more sophisticated tools and controls for defense, but also more savvy advisories and tactics on offense, AI poses a similar paradigm. As the algorithms get more advanced, the risks and potential for harm grows with it. It is crucial to keep the privacy, security, and biases top of mind when leveraging this technology and always calling for the highest standards of transparency, accountability, and protection.
About the Author
Céline Gravelines has 10 years of experience in the cybersecurity industry, specializing in data protection, security policies, incident response, risk & management, vulnerability management, privacy, and more. Named one of the Cyber Defense Global InfoSec Top Women in Security, she currently serves as the Director of Cybersecurity Professional Services at Keyavi where she works with self-protecting data technology to eliminate data loss. Céline holds a BSc in Computer Science and Physics, and a MSc in Computer Science, focusing on applying unsupervised machine learning to brain space.
Céline can be reached by email at [email protected] and at our company website https://www.keyavi.com/.