- You can still buy a 50-inch Hisense 4K TV for $138 at Walmart - here's how the deal works
- New framework aims to keep AI safe in US critical infrastructure
- Temu vs. Amazon: Which shopping site is best for your buying needs?
- ANZ CIO Challenges: AI, Cybersecurity & Data Analytics for 2025
- Want generative AI LLMs integrated with your business data? You need RAG
Building an AI-Native Security Operations Center: Revolutionizing Your Cyber Defense
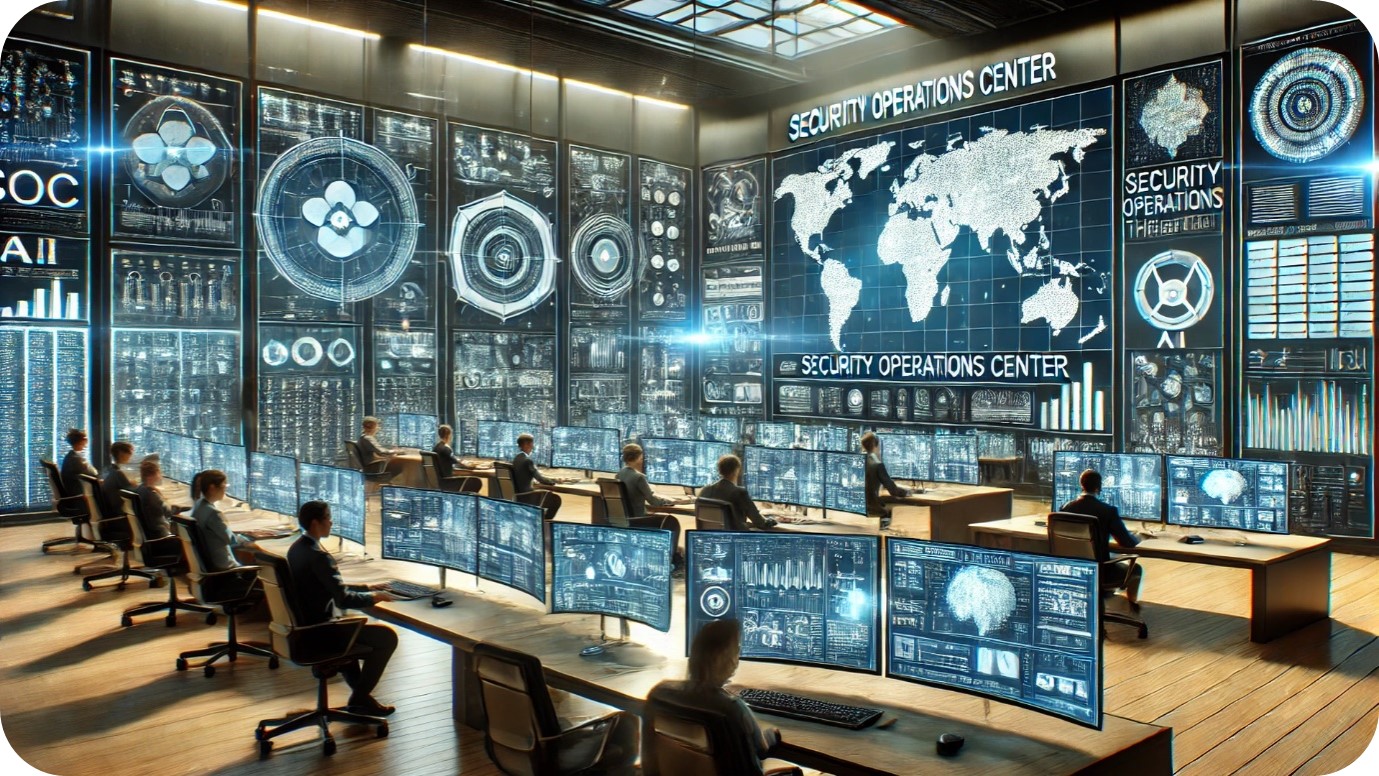
In today’s fast-paced digital world, cyber threats are evolving at an unprecedented rate. For business leaders, safeguarding their organization’s digital assets isn’t just a technical challenge—it’s a strategic imperative. An AI-native Security Operations Center (SOC) represents a transformative leap in cybersecurity, providing the agility, intelligence, and resilience necessary to protect against sophisticated attacks. This blog explores the strategic advantages of an AI-native SOC and outlines a pathway for leaders to embrace this innovation.
Why an AI-Native SOC is a Strategic Game Changer
Traditional SOCs often struggle to keep pace with the volume and complexity of modern cyber threats. An AI-native SOC leverages artificial intelligence to not only detect but also predict and respond to threats in real time. This ensures that your security operations remain ahead of adversaries, providing enhanced protection and futureproofing your security defences.
By handling routine monitoring and initial threat analysis, AI optimizes your security investments, allowing human analysts to focus on more complex, value-driven tasks. This maximizes the impact of your cybersecurity talent and budget while empowering leaders to accelerate decision-making processes, by providing actionable insights faster than traditional methods, which is crucial in mitigating the impact of security incidents.
Expanding the Vision: The Pillars of an AI-Native SOC
The foundation of an AI-native SOC rests on several key components:
- Holistic Data Integration is not merely a technical necessity, within an AI-native SOC, it is the bedrock upon which effective security operations are built. The goal is to create a single source of truth that provides a comprehensive view of the organization’s security landscape. This is achieved by creating a unified data platform that aggregates and consolidates information from network traffic, endpoint logs, user activity, external threat intelligence, and more, into a centralized repository.The challenges of data integration, though, are manifold and must be addressed before any meaningful progress can be made towards an AI-native SOC as AI algorithms depend on accurate data to make reliable predictions. Data from disparate sources can be inconsistent, incomplete, or in different formats. Overcoming these challenges to ensure data quality and consistency requires robust data normalization processes and seamless whole-system integration.
Existing security infrastructure, such as SIEMs (Security Information and Event Management), XDR (eXtended Detection and Response), SOAR (Security Orchestration, Automation, and Response), firewalls, and IDS/IPS (Intrusion Detection Systems/Intrusion Prevention Systems), as well as network infrastructure from the data centre to internal networks, routers, and switches capable of capturing NetFlow, for example, must work in harmony with the new AI tools. This can involve secure engineering (SecDevOps) efforts to develop custom connectors or to leverage middleware solutions that facilitate data exchange between systems.
- Smart Automation and Orchestration are crucial for an AI-native SOC to operate efficiency. Automated response mechanisms can swiftly and accurately handle routine incident responses, such as isolating compromised systems or blocking malicious IP addresses. While orchestration platforms synchronize those responses across various security tools and teams, ensuring a cohesive and effective defence.To confidently reduce the workload on human analysts and minimize the potential for human error, it is critical to develop comprehensive and intelligent playbooks to define automated actions for various types of incidents.
For example, if a malware infection is reported via integrated threat intelligence feeds, the playbook might specify steps to first scan for the IoCs (indicators of compromise), isolate any affected endpoint, scan for other infections, and initiate remediation processes. These actions are executed automatically, without the need for manual intervention. And because you have already seamlessly integrated your security and network solutions when an incident is detected, your orchestration platform coordinates responses across your architecture ensuring that all relevant tools and teams are alerted, and appropriate actions taken at machine speed.
- Human-AI Synergy enhances decision-making. Security analysts benefit from AI-driven insights and recommendations, which augment their ability to make strategic decisions. While AI and automation are powerful, human expertise remains indispensable in the SOC. The goal of an AI-native SOC is not to replace human analysts but to augment their capabilities.For example, when an anomaly is detected, AI can provide context by correlating it with historical data and known threat intelligence. This helps analysts quickly understand the significance of the anomaly and determine the appropriate response.
Continuous learning systems are another vital component. These systems learn from analyst feedback and real-world incidents to improve their performance over time. For instance, if an analyst identifies a false positive, this information is fed back into the AI model, which adjusts its algorithms to reduce similar false positives in the future. This iterative process ensures that the AI system continually evolves and adapts to new threats.
- Advanced AI and Machine Learning Algorithms drive the AI-native SOC’s capabilities. Through proactive anomaly detection, predictive threat intelligence and behavioral analytics these technologies transform raw data into actionable intelligence, enabling the AI-native SOC to detect and respond to threats with unprecedented speed and accuracy.Proactive anomaly detection is one of the primary functions of AI in the SOC. Using unsupervised learning techniques, AI can analyze vast amounts of data to establish baselines of normal behavior. Any deviation from these baselines is flagged as a potential anomaly, prompting further investigation. This capability is particularly valuable for identifying zero-day attacks and advanced persistent threats (APTs), which often evade traditional detection methods.
Predictive threat intelligence is another critical application. Supervised learning models are trained on historical data to recognize patterns associated with known threats. These models can then predict future threats based on similar patterns. For instance, if a specific sequence of events has historically led to a ransomware attack, the AI can alert security teams to take preventive measures when similar patterns are detected.
Behavioral analytics add another layer of sophistication. By analyzing the behavior of users and entities within the network, AI can detect insider threats, compromised accounts, and other malicious activities that might not trigger traditional alarms. Behavioral analytics rely on both supervised and unsupervised learning techniques to identify deviations from normal behavior patterns.
- Ongoing Monitoring and Adaptation ensure that the AI-native SOC remains effective. The dynamic nature of cyber threats necessitates continuous monitoring and adaptation. Real-time threat monitoring involves using AI to analyze data streams as they are generated. This allows the SOC to identify and respond to threats immediately, reducing vital KPIs of MTTA, MTTD, and MTTR. Adaptive AI models play a crucial role in this process. These models continuously learn from new data and incidents, adjusting their algorithms to stay ahead of emerging threats.Feedback mechanisms are essential for maintaining the effectiveness of the SOC. After each incident, a post-incident review is conducted to assess the response and identify areas for improvement. The insights gained from these reviews are used to refine AI models and response playbooks, ensuring that the SOC becomes more robust with each incident.
Implementing Your AI-Native SOC: A Strategic Approach
Successfully implementing an AI-native SOC requires a strategic approach that aligns with your organization’s broader business goals. The following steps outline a comprehensive roadmap for this transformation:
Evaluate Your Current Landscape
Begin by conducting a thorough assessment of your current security operations. Identify existing strengths and weaknesses, and pinpoint areas where AI can provide the most significant benefits. This assessment should consider your existing infrastructure, data sources, and the current capabilities of your security team.
Define Strategic Objectives
Clearly define the strategic objectives for your AI-native SOC initiative. These objectives should align with your organization’s broader business goals and address specific security challenges. For example, your objectives might include reducing response times, improving threat detection accuracy, or optimizing resource allocation.
Select and Integrate Advanced Technologies
Choosing the right technologies is critical for the success of your AI-native SOC. Select AI and automation solutions that complement your existing infrastructure and offer seamless integration. This might involve working with vendors to develop custom solutions or leveraging open-source tools that can be tailored to your needs.
Build a Forward-Thinking Team
Assemble a multidisciplinary team with expertise in AI, cybersecurity, and data science. This team will be responsible for developing, implementing, and managing your AI-native SOC. Invest in ongoing training to ensure that your team remains at the forefront of technological advancements.
Pilot and Scale
Start with pilot projects to test and refine your AI models in controlled environments. These pilots should focus on specific use cases that offer the greatest potential for impact. Use the insights gained from these pilots to scale your AI-native SOC across the organization, addressing any challenges that arise during the scaling process.
Monitor, Learn, and Evolve
Continuously monitor the performance of your AI-native SOC, learning from each incident to adapt and improve. Establish feedback loops that allow your AI models to learn from real-world incidents and analyst feedback. Foster a culture of continuous improvement to ensure that your SOC remains effective in the face of evolving threats.
Overcoming Challenges
Implementing an AI-native SOC is not without challenges. Data privacy and compliance must be ensured, balancing security with privacy concerns. This involves implementing robust data protection measures and ensuring that your AI systems comply with relevant regulations.
Managing false positives is another significant challenge. AI models must be continuously refined to minimize false positives, which can erode trust in the system and waste valuable resources. This requires a careful balance between sensitivity and specificity in threat detection.
The integration process can be complex, particularly when dealing with legacy systems and diverse data sources. Thoughtful planning and expert guidance can help navigate these challenges effectively. This might involve developing custom connectors, leveraging middleware solutions, or working with vendors to ensure seamless integration.
Conclusion
For business leaders, building an AI-native SOC is more than a technological upgrade, it’s a strategic investment in the future security and resilience of your organization. By embracing AI-native security operations, you can transform your approach to Cyber Defense, safeguarding your assets, optimizing resources, and staying ahead of emerging threats. The journey to an AI-native SOC involves challenges, but with the right strategy and commitment, the rewards are substantial and enduring.
Transform your cyber defence strategy today. The future is AI-native, and the future is now.
Share: