- I spent the weekend reading on Amazon's newest Kindle - and it's more capable than it looks
- One of the best cheap Android tablets I've tested isn't made by Samsung or TCL
- Traveling for the holidays? Google Maps uncovers 'hidden gems' to add to your route now
- Gemini Live is available to all iOS and Android users now - for free. How to try it
- The best Kindles of 2024: Expert tested and reviewed
Fortifying the Future: AI Security Is The Cornerstone Of The AI And GenAI Ecosystem
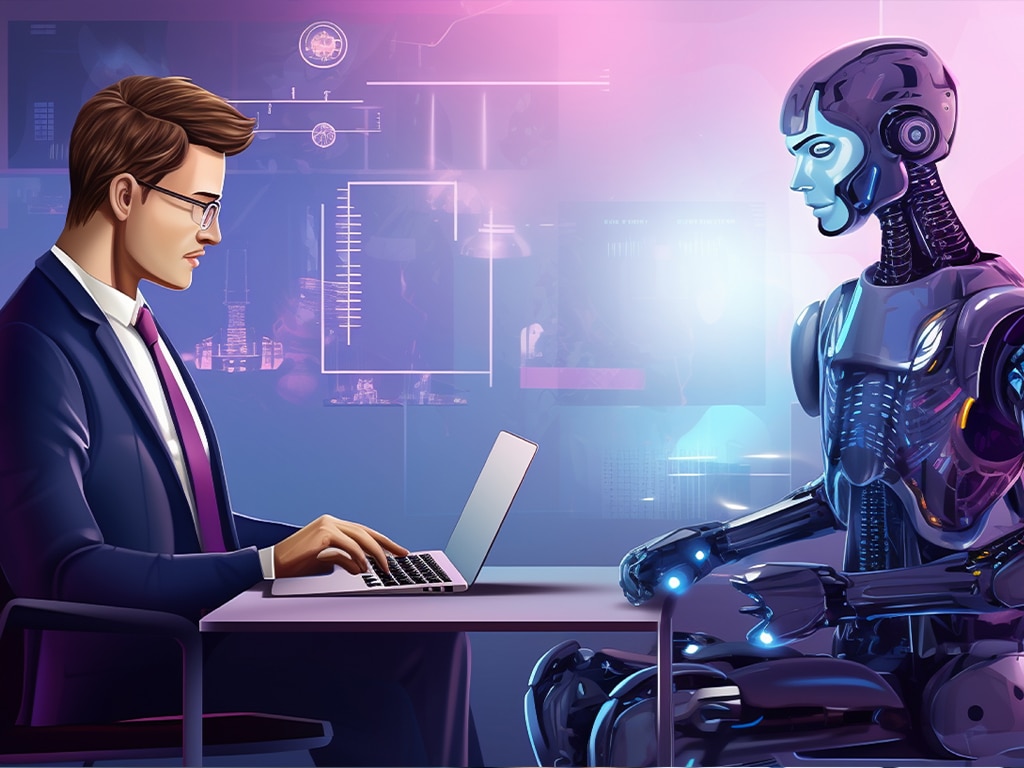
The rapid proliferation of AI technologies is bringing about significant advancements, but it has also introduced a wide range of security challenges. Large language models (LLMs) and computer vision models, key components of generative AI (GenAI), are particularly susceptible to vulnerabilities that compromise security, trustworthiness, and privacy. New solutions are emerging to ensure the safe and ethical deployment of AI systems to address these challenges.
Understanding the Risks
AI models are vulnerable to several types of attacks and mistakes:
- Adversarial attacks, for example when attackers mislead the LLM by adding adversarial content to prompts.
- Hallucination, when AI models generate incorrect or nonsensical information, reducing application accuracy and reliability.
- Data privacy breaches, when AI systems inadvertently leak private data.
- Bias and fairness issues, when AI models perpetuate or even exacerbate existing biases, leading to unfair or discriminatory outcomes and decisions.
- Toxicity, when models produce harmful or offensive content, which is particularly concerning in customer-facing applications.
Evaluation and Risk Assessment
Comprehensive risk assessment solutions are deployed to mitigate AI and GenAI risks. These solutions evaluate AI models on various fronts, identifying vulnerabilities and providing actionable insights to improve security and trustworthiness. Key features of effective risk assessment include:
- Penetration Testing: systematic evaluation of AI models to uncover security weaknesses pre and post deployment.
- Hallucination: detecting and assessing the likelihood AI models will generate false or misleading information.
- Evaluating a model’s overall resilience.
- Privacy: assessing a model’s propensity to leak senJulysitive information.
- Content: detecting and mitigating the generation of toxic, offensive, harmful, unfair, unethical, or discriminatory language.
- Bias and Fairness: identifying and addressing biases within a model to ensure fair and ethical outcomes.
- Weak Spots: pinpointing specific vulnerabilities within AI applications.
Case Studies and Practical Applications: English to French Translation
When DeepKeep evaluated Meta’s LlamaV2 7B LLM, we identified significant weaknesses in its ability to handle translation from English to French. The example below demonstrates the decline in performance DeepKeep found when applying its transformations, resulting in an over 90% drop in accuracy.
The table below showcases 5 test examples:
Original Prompt | LlamaV2 7B’s Translation | Correct Translation |
It is the biggest acquisition in eBay’s history. | C’est l’acquisition la plus importante de l’histoire d’eBay. | C’est la plus grande acquisition de l’histoire d’eBay. |
In Berlin, police estimated 6,500 protestors. | En Berlin, la police a estimé 6 500 manifestants. | À Berlin, la police estime qu’il y avait environ 6 500 manifestants. |
An inquiry was established to investigate. | Une enquête a été créée pour mener une enquête. | Une enquête a été ouverte. |
It has the same molecular structure whether it is a gas, liquid, or solid. | Il a la même structure moléculaire quelle que soit son état (gaz, liquide ou solide). | Il a la même structure moléculaire, qu’il s’agisse d’un gaz, d’un liquide ou d’un solide. |
Since moving to the Catalan-capital, Vidal had played 49 games for the club. | Depuis son arrivée à la capitale catalane, Vidal avait joué 49 matchs pour le club. | Depuis son arrivée dans la capitale catalane, Vidal a joué 49 matchs pour le club. |
Broader Implications
The importance of trust in AI cannot be overstated. The resilience and reliability of GenAI models is becoming critical as enterprises increasingly integrate GenAI into business processes. Evaluating AI models during their inference phase — when they are actively generating outputs — is essential for ensuring they are trustworthy, effective, private and secure.
AI Security’s Role is The Ecosystem’s Foundation
As AI technology evolves, so do the strategies and tools required to secure it. AI security is not just about protecting models from external threats, but also about ensuring they operate ethically and responsibly, providing insights into potential risks and vulnerabilities. This includes adhering to regulatory requirements, maintaining transparency, and safeguarding user privacy. Comprehensive AI security platforms are an essential foundation of the AI and GenAI ecosystem.
About the Author
Dr. Rony Ohayon is the CEO and Founder of DeepKeep, the leading provider of AI-Native Trust, Risk, and Security Management (TRiSM). He has 20 years of experience within the high-tech industry with a rich and diverse career spanning development, technology, academia, business, and management. He has a Ph.D. in Communication Systems Engineering from Ben-Gurion University, a Post-Doctorate from ENST France, an MBA, and more than 30 registered patents in his name. Rony was the CEO and Founder of DriveU, where he oversaw the inception, establishment, and management. Additionally, he founded LiveU, a leading technology solutions company for broadcasting, managing, and distributing IP-based video content, where he also served as CTO until the company was acquired. In the education realm, Rony was a senior faculty member at the Faculty of Engineering at Bar-Ilan University (BIU), where he founded the field of Computer Communication and taught courses about algorithms, distributed computing, and cybersecurity in networks.
Rony can be reached online at https://www.linkedin.com/in/rony-ohayon-40232716a/?originalSubdomain=il and at our company website https://www.deepkeep.ai/