- Get a free iPhone 16 Pro for free from T-Mobile, no trade in required - here's how
- Prime members can save $10 on any $20 or more Grubhub+ order for a limited time - here's how
- Get a Galaxy S25 phone, Watch, and Tab free from Verizon - here's how
- Best early Prime Day laptop deals: My 12 favorite sales live now
- From Innovation to Action: Seizing the $43B Networking Refresh Opportunity with Cisco
Guardians Of the Grid
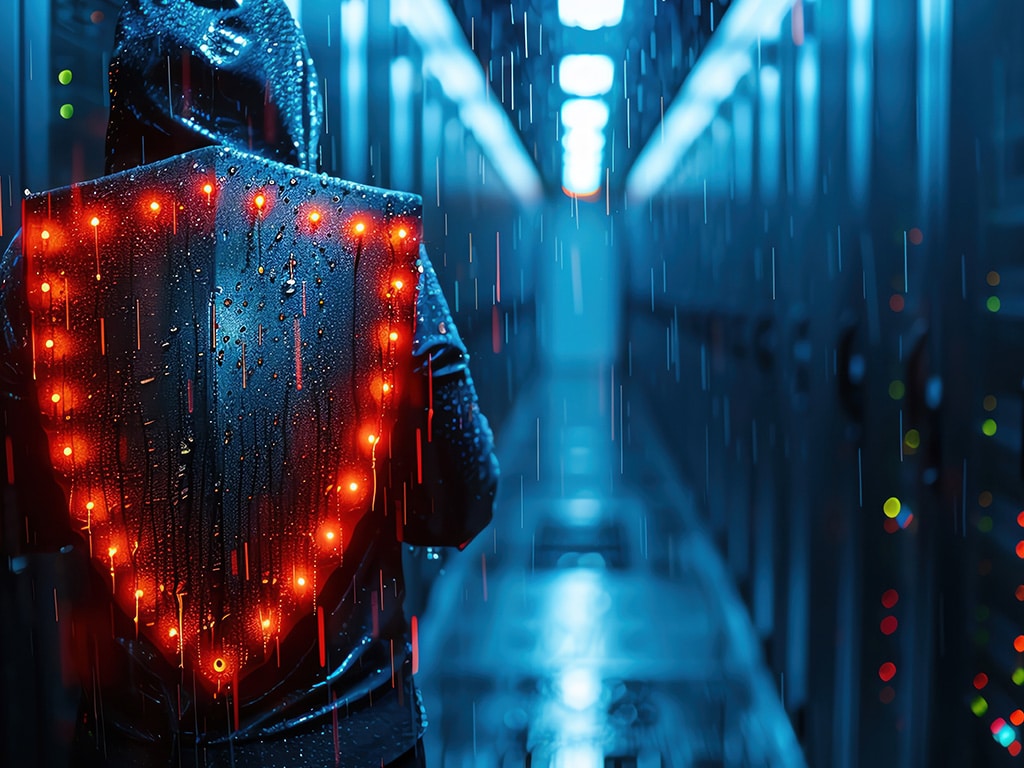
The surge in cyberattacks and the emerging role of Generative AI
The importance of cyber security tools in protecting sensitive information, sustaining organization’s resilience and enabling business continuity during hostile attempts was testified to by the events of cybercrime over the previous year:
- In May 2024, the UK Ministry of Defense had a payroll system breach that led to personal information about almost 270,000 employees being exposed.
- In March 2024, French state services were targeted by a large denial-of-service (DDoS) attack that affected more than 300 web domains and 177,000 IP addresses linked to government.
- In February 2024, Change Healthcare, one of the major US health payment processors experienced a ransomware attack by ALPHV/BlackCat gang with dire consequences. The incident stopped payment processing for some weeks causing as much as USD 100 million daily losses and yet again emphasizing the need for cyber security.
Generative AI has shown potential to disrupt the cybersecurity landscape. Although current and future applications of Gen AI models mainly focus on text, audio video and image-based modalities learning and replication; these models can also identify threats or vulnerabilities themselves, so they predict patterns and trends thus helping mitigate cyber threats. According to a report published by MarketsandMarkets, the market for Generative AI Cybersecurity is anticipated to experience substantial expansion with a compound annual growth rate (CAGR) of 33.4% between 2024 to 2030. This dramatic surge is being fueled by a number of causes. The primary growth driver is the enhancement of existing cybersecurity tools through generative AI algorithms by improving anomaly detection, automating threat hunting and penetration testing, and providing complex simulations for security testing purposes. These techniques enable various cyber-attack scenarios that can be simulated using the Generative Adversarial Networks (GANs), thus enabling the development of better preparedness and response strategies.
Implications of Generative AI within Cybersecurity
Generative AI presents promising applications for improving cybersecurity defense strategies. Generative AI based algorithms can simulate multiple attack scenarios, enabling cybersecurity professionals to anticipate and mitigate risks before they become real-world issues. Moreover, generative AI can automate routine security tasks, enabling security experts to focus on more complex issues.
Like with any rampant technology on the rise, the implementation of Generative AI also poses some stark questions to consider. While the benefits outweigh the negative implications, the technology also has its loopholes that can expose the system to new forms of insecurity. The most concerning issue is the ability of malicious actors to utilize generative AI to build sophisticated phishing attacks, create deep fake messages, and develop malware.
To realize the advantages of generative AI while managing possible misuse, a multifaceted approach must be adopted. This consists of strengthening the organizational cybersecurity framework to empower security analysts and experts at the implementation stage and incorporating robust training and processes to identify potential cybersecurity threats and how to overcome them. However, the principles of ethics cannot be left out of the picture as modern enterprises embark upon the journey to a transformative Gen AI cybersecurity revolution.
Why is Generative AI an imperative for cybersecurity teams?
While the use cases are paramount and positive annotations continue to drive deployment and implementation across the enterprise value chain, potentially, the demands of modern enterprises typically hinge on the ‘detection’ and ‘remediation’ of cyber threats. To broadly categorize, factors that continue to drive the adoption of Generative AI based cybersecurity solutions include:
- Generative AI’s ability to foresee and flag emerging cyber threats drives the future of pre-emptive cybersecurity measures.
- The self-improving nature of generative AI ensures cybersecurity systems evolve alongside new attack vectors and tactics
- Generative AI excels in correlating vast and diverse data sets to uncover hidden threats that traditional methods miss
- The ease of integrating generative AI with current cybersecurity frameworks accelerates adoption and enhances overall defense mechanisms
- Generative AI optimizes resource allocation by prioritizing critical security alerts, ensuring that human and technical resources are used most effectively
Use Cases of Generative AI in Cybersecurity
- Real-Time Threat Detection and Enhanced Threat Intelligence
Generative AI has the capability to assess and understand a large amount of real time data that is essential in detecting early possible threats. The existing traditional systems find it hard to handle the velocity and volume of data that results from modern networks. However, generative models can sift through such data thereby identifying anomalies or patterns indicating cyber threat. These models learn from new data continuously hence they are able to match up with changes in the cyber criminals’ tactics thus acting as proactive defense.
A good example is IBM’s QRadar advisor which uses artificial intelligence for analyzing both structured and unstructured information coming from various sources. This system combines data drawn from different events to detect threats that may not be visible under ordinary circumstances. According to IBM, QRadar Advisor with Watson lowered average response times by 60% which indicated effectiveness of AI in threat detection.
- Improved Incident Response Management
The speed and response efficiency in the event of cyber incident is crucial to curtail damage. The automation of several aspects of the process by generative AI can make incident response better. For instance, AI models can assist in rapidly recognizing the type of attack, identifying its origin and learning about the compromised systems. This automated analysis provides security teams with actionable insights such that their focus is shifted from diagnosis to implementing solutions.
Darktrace is a cybersecurity firm whose technology uses AI to respond to threats autonomously. In UK city council during ransomware assault, Darktrace’s AI identified and responded the real threat which prevented spreading the ransomware and reduced impacts of attacks. There was significant disruption and financial loss associated with this immediate response.
- Secure Software Development Lifecycle (SSDLC)
Generative AI can help address SSDLC security issues by providing automatic identification of code vulnerability and configuration errors during a development process. As well as identifying problematic areas and suggesting possible remedies, AI tools may be used to write secure coding sequences.
A major example of how AI is used in the Security Development Lifecycle (SDL) at Microsoft. Microsoft has developed AI tools that are capable of checking millions of lines of code for vulnerabilities before they are deployed. This has greatly reduced the number of weaknesses that their products suffer from thereby increasing the general security level therein.
- Supplementing Security Analysts
Security analysts often deal with voluminous amounts of threats and alert notifications, which warrant quick redressal. Generative AI proves to be helpful in this regard, taking over such tasks as log analysis, threat hunting, or incident prioritization. For example, generative AI can sieve out false positives, flagging critical issues and provide detailed context to help analysts concentrate on more intricate and strategic assignments.
An illustration that demonstrates this is JPMorgan Chase’s application of gen AI-native cybersecurity across its financial services. The COiN (Contract Intelligence) by JP Morgan Chase uses artificial intelligence systems to extract valuable information from legal documents and thereby reducing the analyst’s workload for accurate compliance and risk management purposes. JPMorgan Chase has optimized their work with artificial intelligence in order to handle security and compliance risks better than they did earlier with traditional cybersecurity tools.
- Ensuring Resiliency and Business Continuity Management
Business continuity is of utmost concern to organizations, especially amid cyber threats. In this regard, Generative AI can help in boosting systems and processes resilience, as generative AI models can simulate various attack scenarios and assess their impact on business operations. A proactive nature enables organization’s identification of potential weak points and implementation of measures aimed at mitigating the risks before materializing.
FireEye for instance uses AI technology to model different kinds of cyber-attacks that may happen; thus assessing how much it will affect clients. The use of such a technology allows organizations to come up with solid plans for business continuity, which means they can handle real-world digital threats more effectively when these occur. Thus, FireEye’s approach based on AI has allowed many companies enhance their cyber defense posture while still running their businesses during an intrusion.
- Guard railing of Large Language Models (LLMs)
LLMs such as OpenAI’s GPT-4 and Google’s Gemini have demonstrated impressive abilities in generating human-like text. However, the same powerful tools can also be misused by unscrupulous individuals to create very convincing phishing emails, fabricate fake news or even design new strains of malware. To prevent this, developers implemented strong guardrails.
Content filtering is one of the main means through which the risks are mitigated whereby LLMs’ outputs are inspected for dangerous or unethical contents like hate speech and misinformation before being shared with users through algorithms. OpenAI uses content filters that detect and block any violations of ethics when using these technologies. In this regard, OpenAI has an API that offers its models under strict usage conditions while being vigilant to activities that may signal some type of dubious activity going on at their end. User access restrictions and constant surveillance keep LLMs protected against misuse. To avoid possible abuses, developers may limit model availability by determining who can use them and how they use them. This implies that they always watch over their technology so as to detect discrepancies in time, which helps maintain credibility.
Amalgamation of generative AI with cybersecurity: the road ahead
The cyber security scene is a battlefield where the stakes have never been higher and the enemies never wilier. In such an environment, generative AI becomes not only a tool but also an agent of transformation that redefines how we approach digital defense. Generative AI enables cyber security teams to outsmart malicious actors with predictive models that come up with threats before they occur and automate monotonous tasks that are however crucial.
Just think about it; imagine a world where cyber threats get neutralized long before they cause destruction, where incident responses are fast and definitive, and where software development is in-built secureness. This is the future that generative AI promises—a future where security becomes proactive rather than reactive, sophisticated instead of primitive. It’s a future in which human genius combines with computer precision to provide a wall against the menace of online attacks.
However, we must take great care in the ethical implications and potential abuse of this technology. By introducing safeguards that are well-designed and encouraging responsible AI culture, generative AI’s power can be fully harnessed while mitigating against its perils.
Generative AI is the grandmaster in the grand chess game of cybersecurity. Organizations should leverage this powerful ally to protect their digital strongholds. The age of generative AI in cybersecurity has come and with it a pledge for a more secure and resilient digital world.
About the Author
Rounak Singh is a Senior Research Analyst with the ICT team at Marketsandmarkets Research Private Ltd. He has over 5 years of experience as a strategic consultant and market research analyst, delivering diverse projects around Artificial Intelligence (AI) and Analytics. His current role sees him spearheading several syndicate and bespoke market studies, with special emphasis around the booming generative AI and Large Language Models ecosystem. He is also responsible for creating synergies with clients operating in the AI and Analytics domain, assisting them in identifying revenue maximization opportunities and hot bets.
Rounak can be reached online at LinkedIn and at our company website https://www.marketsandmarkets.com/