- Run LLMs Locally with Docker Model Runner | Docker
- Microsoft unveils 9 new Copilot features - you can try some now
- Nintendo Switch 2 pre-orders delayed, new price hike likely - here's why
- Why Temu's bargain prices are about to hit a tariff wall
- 5 easy ways to instantly improve your soundbar's audio (without spending a dime)
How AI Is Transforming Cyber Risk Quantification
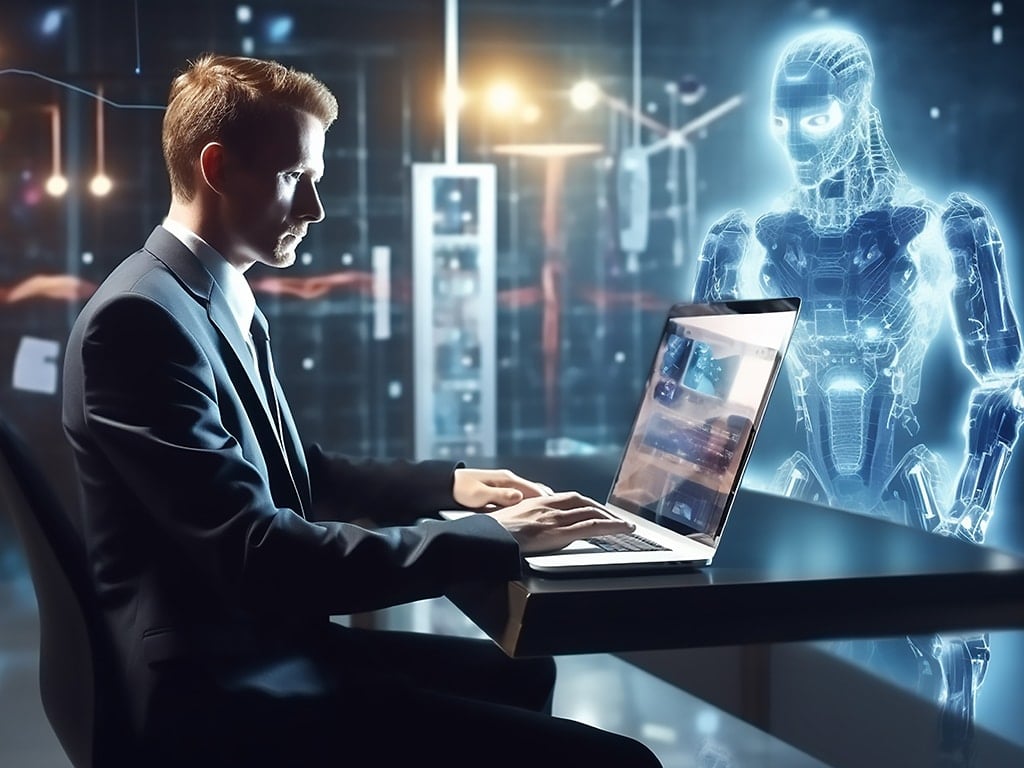
Cyber risks differ from other more familiar risks in life, such as the dangers of a car crash for drivers, or a natural disaster for homeowners. These are well-defined, measurable risks with associated costs that insurers can estimate.
Yet despite the sophistication of modern IT cybersecurity protections, most large organizations still lack substantial empirical data about the risks facing their industrial control systems (ICS) and operational technology (OT) at utility plants, refineries, and factories. This shortcoming is due to the limited availability of data about OT cyber incidents, and an inability to apply traditional actuarial methods to estimate the potential financial consequences.
The lack of data at these facilities makes inherent uncertainty a key challenge for quantifying and prioritizing cyber risk. To get a better handle on this problem, a new field has emerged called Cyber Risk Quantification and Management, or CRQM, which relies on risk transfer practices. New CRQM platforms benefit from the use of artificial intelligence and data-driven tools to better manage, mitigate, and eventually transfer cyber risks to insurers.
To address the glaring gaps in publicly available data and thus account for so much uncertainty, AI-data-driven CRQM platforms use probabilistic models such as Bayesian networks, and probabilistic graphical models. All these approaches can be applied with AI to explicitly represent uncertainty, as they assign probabilities to different outcomes, which helps the AI system make informed decisions based on uncertain data.
You Can’t Quantify What You Don’t Understand
The volume of information required to monitor the interdependencies between cyber physical systems, networks, and the cloud has become too enormous to be processed by mere human intelligence. AI-powered systems can be used to logically identify and automate the processing of data from interconnected systems and analyze the data to deliver continuous outputs that are always up-to-date.
To underwrite a risk, one first needs to understand it. That is why risk data is the lifeblood of the insurance industry. But in many cases, the datasets for operational technology remain incomplete. Or there might be duplicate sources of data from different inputs. Having a precise process to reconcile and normalize all that ingested information requires the creation of a data ontology for cybersecurity.
When AI is fed with enough dependable data about cyber risk, it can bring unprecedented accuracy and speed to help understand risk. The underlying concerns include vulnerability detection, prioritization of security tasks, and the cascading impact of cyber incidents on a network of interconnected critical infrastructure.
By enabling a better assessment and quantification of cyber risk, especially for OT environments and cyber-physical systems, AI also enhances risk transfer practices. On one end, companies get a more thorough understanding of their cyber risk to decide what risk to accept, avoid, transfer, or mitigate. On the other end, underwriters get more evidence-based data to align their cyber insurance parameters, including their policy coverage and limits.
Taking Advantage of AI in the Cloud
AI can help us quantify cyber risk and define the best risk mitigation strategies. Cloud-based CRQM platforms use AI algorithms to normalize and categorize ingested data from dozens of sources, including internal data and raw signals from cybersecurity solutions for intrusion detection and vulnerability management. In addition, natural language processing (NLP) is applied to analyze text and process cyber incident information about victims and threat actors.
To show the scope of computing efforts this represents, CRQM platforms regularly perform millions of Monte Carlo simulations on monitored sites to model the probability of different outcomes for a range of processes that cannot be easily predicted. These simulations run what-if analyses on suggested mitigation projects to identify the ones with the greatest positive impact on risk reduction. Machine learning is also employed to model complex dependencies in the aggregation of risk based on impact and frequency.
This bottom-up approach to risk aggregation enables CRQM platforms to reliably predict where and how incidents are likely to occur in each client’s unique context, and then translate that information into dollars at risk. The critical financial metrics that CRQM delivers to CISOs include value at risk, probability of loss, financial impact of cyber incidents, expected ROI, and risk reduction from evaluated risk mitigation projects. By applying these CRQM principles coupled with AI techniques, CISOs and CFOs can work together to craft the most appropriate risk mitigation strategies for their distinct facilities.
About the Author
Jose M. Seara is the Founder and CEO of DeNexus, a leader in Cyber Risk Quantification and Management for Operational Technology (OT) and Industrial Control Systems (ICS). Jose was previously the President & CEO of NaturEner USA (now BHE Montana) & NaturEner Canada from November 2006 to January 2018. During his time at NaturEner, Seara led the company through a leadership transition, working to ensure a smooth transition for the new team.
Prior to his time at NaturEner, Seara was a founding partner and member of the board of directors at DeWind Co from June 1999 to September 2002. Jose was also a founding partner and principal at PROYDECO Ingenieria y Servicios SL from January 2003 to December 2006, and a partner and director at Proyectos de Cogeneración SL from January 1999 to December 2003.
He holds an Executive Program degree from Singularity University in the field of Exponential Technologies. Jose also holds a Master’s of Science in Naval & Marine Engineering from Universidad Politécnica de Madrid.
Jose can be reached at the DeNexus website: https://www.denexus.io/