Is your network AI as smart as you think?
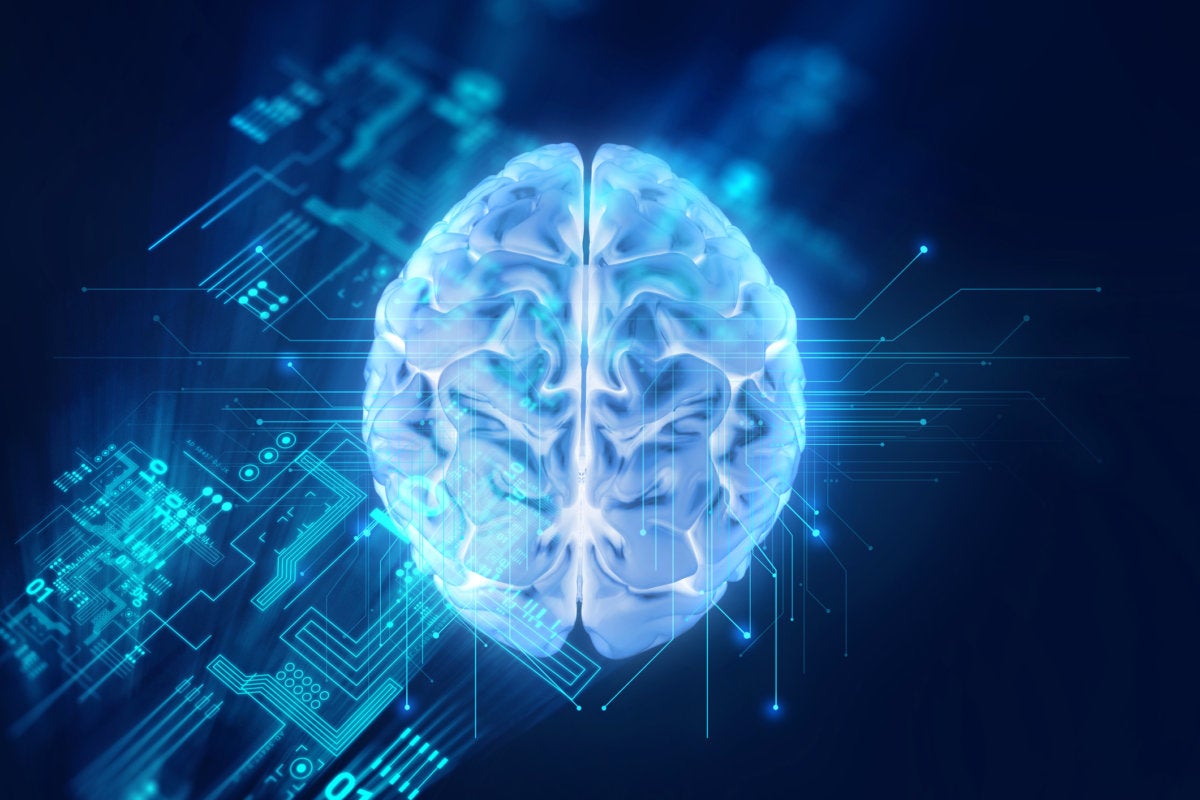
Network-operations types tell me that, in the future, AI is going to manage their networks. They also tell me that their vendors told them that very same thing. The good news is that’s sort-of-true. The bad news is the same; with emphasis on the qualifier “sort-of”. To get the most from AI network management, you have to navigate out of that hazy “sort-of” zone, and you do it by thinking about ants and farmers.
Ants can build wonderfully complex anthills, with all manner of interconnecting tunnels and levels. Do the worker ants have some mighty engineer-ant directing this process? Nope. Each of them is single-mindedly performing its own simple task, and instincts program them. There is in fact an ant-engineer, but it’s their own DNA that’s organized their work to accomplish the goal. That’s a bit like how most network AI works.
Networks are made up of a bunch of technology “collections”, each a bit like an anthill. There are collections based on vendor, on device type, on physical location, and on connection relationships. If you look at network AI today, it operates mostly on collections. Maybe it manages Wi-Fi or maybe edge elements like SD-WAN or SASE. AI applications to manage a collection have the management objectives built into their DNA, their design. We know how Wi-Fi works if we’re a Wi-Fi vendor, and we build that knowledge into our AI management.
The challenge comes when we stop looking at collections as independent elements and start looking at networks as collections of collections. A network isn’t an anthill, it’s the whole ecosystem the anthill is inside of including trees and cows and many other things. Trees know how to be trees, cows understand the essence of cow-ness, but what understands the ecosystem? A farm is a farm, not some arbitrary combination of trees, cows, and anthills. The person who knows what a farm is supposed to be is the farmer, not the elements of the farm or the supplier of those elements, and in your network, dear network-operations type, that farmer is you.
In the early days, the developers of AI explicitly acknowledged the separation between the knowledge engineer who built the AI framework and the subject-matter expert whose knowledge shaped the framework. In software, especially DevOps, the management tools aim to achieve a goal state, which in our farm analogy, describes where cows, trees, and ants fit in. If the current state isn’t the goal state, they do stuff or move stuff around to converge on the goal. It’s a great concept, but for it to work we have to know what the goal is. We need, at the level of an enterprise network, the knowledge that our Wi-Fi expert subliminally introduced into the Wi-Fi AI management tool. If an AI vendor doesn’t know how that knowledge is obtained, their AI can’t help.
Before you decide that your hopes for AI are forever dashed, take heart! Many network-operations types are perfectly happy with AI that manages the collections of technology that make up their network. After all, why worry about coordinating Wi-Fi and SD-WAN management when whatever happens with one can’t be remedied by jiggling the other? If this collection-AI model fits your needs, you’re home free.
A good way to see if it is OK being an ant (network AI-wise, at least) is to ask whether your technology collections are really atomic—totally independent, self-contained. It comes down to the visibility and control scope of your AI. Collection-specific AI keeps to itself, basically. Ideally, you need your AI collection ants to do their own thing, without stepping into one another’s activity. You don’t want AI in one place to be looking over into another collection and reacting to conditions, or two AI-collection processes working on the same problem at the same time, without coordination.
If the remedies for issues in one collection might involve doing something to another collection, then you need your AI to rise up and cover the combination. So, if you see an expensive and overworked network operations center that manages ecosystemic problems and wonder whether AI could let everyone take a coffee break, you need some deeper insight into vendor AI claims. That’s not easy for enterprises, because more than three-quarters of those I’ve chatted with this year say that they don’t have much, if any, AI expertise in-house. Many feel like they’re at the mercy of vendors, who promise great things and don’t seem to quite deliver what’s expected. Is there nothing an enterprise can do?
The easiest way to get a handle on using AI for an entire network ecosystem is to look for a strategy that’s kind of like the old “manager of managers” approach. In modern terms, you could call this intent modeling. If each of your technology collections can be treated as a black box that models its behaviors against its own SLA, and if its AI process works to enforce that SLA, then all you need is for each of those collection AI tools to generate a failure report to a higher-level package. That package can then decide what to do if there’s either a problem that goes beyond a single collection of technologies, or if one collection just throws in the towel and a higher-level fix has to be considered.
The challenge here will be finding that goal state and how to get back to it when something goes wrong. Remember those subject-matter experts and knowledge engineers? It’s difficult to frame an AI solution to a network because all networks are a bit different, and only the users know what they consider “good” or “bad”. Some AI tools may offer a machine-learning (ML) capability that looks over the shoulder of your NOC people and learns what to do, and some may use a baseline that a network vendor knows would usually represent normal options and common remedies.
Both approaches have some issues. Machine learning can take time, and while your AI system is learning its mission, it can drain your NOC’s resources further. Vendor baselines work best when a network is largely made up of equipment from one vendor. Both can be tuned up, but both can run afoul of adaptive network behavior.
IP networks essentially use topology discovery and do their own thing. Influencing the routing is difficult even for the NOC; they’d often have to plan new MPLS routes to do traffic engineering, something AI isn’t likely to do. Some companies (including Google) have gone to software-defined networking (SDN) to provide central control of routing, and AI could then control the network by controlling the SDN controller.
AI in network operations goes back to the combination of events to signal changes, and a way of implementing an effective response. At any level, your prospective AI vendors should be able to talk you through how their offering gathers information, and how it implements its insights. Dig into the detail of those two things, because whatever magic AI claims to work, it won’t work it without those two ingredients. Be a farmer, not an ant.
Copyright © 2021 IDG Communications, Inc.