- Nvidia AI supercluster targets agents, reasoning models on Oracle Cloud
- Palo Alto unpacks security platform to protect AI resources
- This E Ink tablet that runs on Android is irreplaceable when I'm traveling
- Why the LG G4 OLED is one of my favorite TVs for picture quality - even in 2025
- Why the Oura Ring 4 is still the best smart ring on the market - and I've tested dozens of them
MCP for DevOps – Series Opener and MCP Architecture Intro
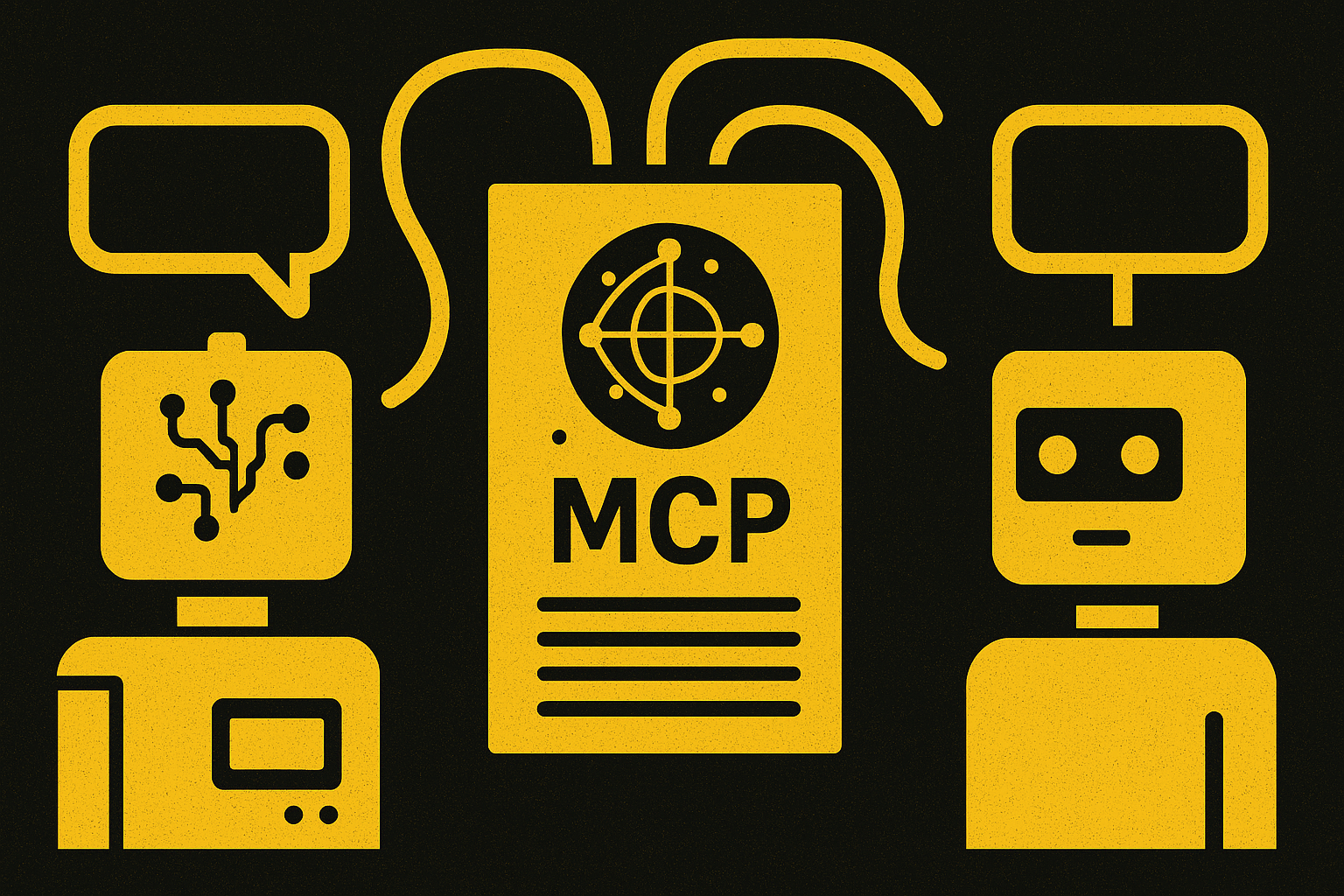
MCP for DevOps – Series Opener and MCP Architecture Intro
You have undoubtedly heard about Anthropic’s MCP (Model Context Protocol) open source project. If you haven’t, I hope your vacation on a remote island without internet access was lovely!
As a die-hard YouTube Premium fan, I am inundated with video recommendations with themes like “What is MCP?” “OMG, This Changes Everything,” and my favorite, “Goodbye Developers, MCP is Here to Stay.” Seriously? While it is a fantastic project, it isn’t here to replace us.
Over the next several weeks, I will delve into these topics:
MCP—Why Should You Care?: This will provide a brief overview of MCP from a communication, discovery, and interaction perspective. We will then explore what it looks like on the wire and how it functions as a client/server architecture, followed by various use cases. I won’t cover the history of MCP or other essential information, as countless excellent resources are available on YouTube, dev.to, Medium, and elsewhere.
MCP for DevOps: I will discuss a selection of use cases that work well for DevOps, NetOps, and SecOps roles.
MCP How-to: This is where things get exciting. I will present multiple demos and walk-throughs for the following use cases:
- Cursor with GitHub: Use Cursor as an MCP client to programmatically interact with an MCP server that integrates with GitHub for a Cisco DevOps workflow
- Cursor with Argo CD: Use Cursor as an MCP client to programmatically interact with an MCP server that employs Argo CD for a Cisco DevOps workflow
- Claude Desktop & DevOps Workflows: We will switch things up by using Claude Desktop instead of Cursor to demonstrate flexibility on the MCP client side
At the end of the series, I will tie all of this together to show how Cursor, with multiple MCP clients, can drive changes to Ansible playbooks in a GitHub repository, triggering actions in the Argo CD workflow. Ultimately, we will use the Ansible playbook to modify configuration settings on Cisco solutions such as Cisco ISE (Identity Services Engine) and other Cisco products.
I hope you join me on this journey.
Let’s get started with discussing the MCP architecture and why you should care about it.
MCP Intro—Why Should You Care?
Welcome to the first post in our three-part technical series on Model Context Protocol (MCP), a new, focused protocol built to help AI applications and agents interact with tools, APIs, files, and databases consistently and programmatically.
If you’re in DevOps and experimenting with AI-driven automation, MCP deserves your attention—not as a silver bullet but as a practical step toward cleaner integration between AI systems and your operational stack. That said, it’s early days. MCP is new and moving fast, and while it already solves a number of real-world problems, there are still corners to polish and edge cases it doesn’t yet cover.
What is MCP, and Why Does It Matter?
As illustrated in Figure 1, Model Context Protocol (MCP) is a protocol that provides a uniform way to plug in an AI model into tools and services.
Figure 1. MCP with LLMs and Tools
It is:
- A lightweight communication protocol designed specifically for AI agents and applications.
- Built to connect those agents to tools, APIs, databases, and file systems.
- Structured as a client/server architecture—simple and predictable.
- Plumbing
It is not:
- A messaging protocol for agent-to-agent communication.
- An LLM, database, AI assistant or agent.
- A general-purpose integration platform.
- A replacement for your existing APIs or data bus.
MCP’s job is tightly scoped: give an AI agent a clean, standardized way to discover, request, and invoke capabilities on existing tool-based infrastructure. If your LLM-powered bot needs to call a REST API, list files, or query a database—MCP provides the glue.
MCP matters because it reduces and, in many cases, removes the toil for AI applications and agents to find, connect to, and leverage external tools and services such as APIs, data sources, and other non-AI native tool sets. For Dev/Net/SecOps staff, it can bring immediate value for you to leverage an AI agent to connect to your existing data sources and APIs so that an operationally-focused agent can more accurately complete tasks.
We will discuss use cases in the next blog, but imagine you need to create a workflow that works with Ansible Playbooks, NetBox, and GitHub and automate configurations against your infrastructure.
An example workflow may look like this:
- You manually create a Jinja2 template for Ansible and host it on GitHub.
- Gather data from your NetBox deployment.
- You use Python + Jinja2 to populate the playbook template with data from NetBox and then invoke Ansible via a Python module, CLI, runner, etc.
- Ideally, you use a CI/CD tool to auto-run this workflow.
Fast forward from the good ’ole days; you or someone in your organization learn about the power of AI Agents and create a series of AI agents that can tap into each tool and data source without writing any code. They can leverage MCP to connect to each resource as MCP servers and interact with them natively—no special script code. No scouring the internet for SDKs or some mysterious script someone recommends that you don’t understand. To me, this is one of many value-add use cases of MCP.
Overview of MCP – Architecture and Core Components
MCP has a streamlined architecture and there aren’t many moving parts.
As illustrated in Figure 2 MCP uses a client/server architecture. Let’s define what the client and server components do.
Figure 2. MCP Components
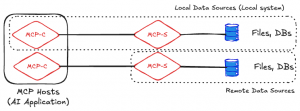
Figure 2 shows an MCP host which is an AI application such as an AI agent, IDE, coding assistant, etc..
The MCP client (MCP-C) is software that runs on MCP hosts and has one-to-one connections to MCP servers (MCP-S).
The MCP server is software that represents specific service or tool capabilities.
The MCP host uses the language-specific MCP SDK for client connections (example: MCP Python SDK) to establish connections to MCP servers. The MCP SDK is used for both client-side and server-side code.
Example Python MCP client code.
Example Python MCP server code.
Many current MCP clients are complete applications or AI agents with the MCP client SDK functionality natively built in. You can see an example list here: https://modelcontextprotocol.io/clients
There are numerous sources of MCP server lists on the Internet. Here is a list from the MCP project: https://modelcontextprotocol.io/examples. Some MCP client providers, such as Cursor, have their own list of servers: https://cursor.directory/.
Figure 2 shows that each MCP-C instance has a one-to-one connection to each MCP-S instance. In the figure, there are two MCP clients running on the MCP host, an AI agent in this example. The first MCP client is connecting to a locally-hosted MCP server that provides local machine file system access. The second MCP client is connecting to a remotely hosted MCP server that is providing access to a remote file system.
MCP clients exchange messages with MCP servers using JSON-RPC 2.0 (as the wire format). For local data sources, MCP uses JSON-RPC over stdio (Standard Input/Output) as the transport. Figure 3., illustrates how an MCP-C connects to a local MCP-S for file or DB access using stdio. The MCP-S sends JSON-RPC messages to its standard output / stdout and reads from the standard input / stdin.
Figure 3. JSON-RPC over stdio

Here is an example of running an MCP filesystem server locally in stdio mode and restricting access to a very specific directory:
npx -y @modelcontextprotocol/server-filesystem /Users/shmcfarl/code/mcp-testing Secure MCP Filesystem Server running on stdio Allowed directories: [ '/Users/shmcfarl/code/mcp-testing' ]
Using a great test tool such as the MCP Inspector you can pair a local client (MCP Inspector) with your locally running stdio or HTTP+SSE server:
npx -y @modelcontextprotocol/inspector npx -y @modelcontextprotocol/server-filesystem /Users/shmcfarl/code/mcp-testing Starting MCP inspector... Proxy server listening on port 3000 MCP Inspector is up and running at http://localhost:5173 Query parameters: { transportType: 'stdio', command: 'npx', args: '-y @modelcontextprotocol/server-filesystem -y /Users/shmcfarl/code/mcp-testing', . . . [Output removed for clarity] Spawned stdio transport Connected MCP client to backing server transport Created web app transport Created web app transport Set up MCP proxy Received message for sessionId 697bd02d-5d67-4dfc-85b9-6a12d6a99f45 Received message for sessionId 697bd02d-5d67-4dfc-85b9-6a12d6a99f45 Received message for sessionId 697bd02d-5d67-4dfc-85b9-6a12d6a99f45 Received message for sessionId 697bd02d-5d67-4dfc-85b9-6a12d6a99f45
MCP supports HTTP+SSE (Server-Sent Events) to send structured requests from service backends using MCP servers to MCP clients for local or remote connections. The 2025-03-26 specification changes states that MCP is moving to a more flexible Streamable HTTP transport. However, HTTP+SSE transport can still be used for backward compatibility. This keeps it transparent, traceable, and tool-agnostic. Note: As of the time of writing this blog, the new Streaming HTTP support is not completed in each SDK.
Figure 4 illustrates the connection flow for HTTP+SSE scenarios. In the figure, HTTP POST is used for MCP-C -to- MCP-S messages. HTTP+SSE is used for MCP-S -to- MCP-C messages.
Figure 4. MCP-C -to- MCP-S communication using HTTP+SSE
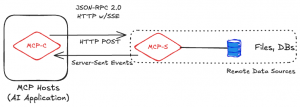
You can go through the MCP quickstart server and client guides to learn how to setup your own weather client/server combo: https://modelcontextprotocol.io/quickstart/server. Using a similar setup, you can see some HTTP messages for stuff like a tools list call:
POST /messages/?session_id=6ccde3779adf43cc9d3f5f661508310b HTTP/1.1 Host: 0.0.0.0:8080 Accept: */* Accept-Encoding: gzip, deflate Connection: keep-alive User-Agent: python-httpx/0.28.1 Content-Length: 46 Content-Type: application/json {"method":"tools/list","jsonrpc":"2.0","id":2} HTTP/1.1 202 Accepted date: Tue, 08 Apr 2025 20:14:51 GMT server: uvicorn content-length: 8 Accepted
And a tool call to get the weather forecast:
POST /messages/?session_id=6ccde3779adf43cc9d3f5f661508310b HTTP/1.1 Host: 0.0.0.0:8080 Accept: */* Accept-Encoding: gzip, deflate Connection: keep-alive User-Agent: python-httpx/0.28.1 Content-Length: 134 Content-Type: application/json {"method":"tools/call","params":{"name":"get_forecast","arguments":{"latitude":39.7392,"longitude":-104.9903}},"jsonrpc":"2.0","id":3} HTTP/1.1 202 Accepted date: Tue, 08 Apr 2025 20:14:54 GMT server: uvicorn content-length: 8 Accepted
And a response for the weather forecast prompt I entered for Denver, CO:
event: message data: {"jsonrpc":"2.0","id":3,"result":{"content":[{"type":"text","text":"nThis Afternoon:nTemperature: 74..FnWind: 12 mph WnForecast: Partly sunny. High near 74, with temperatures falling to around 72 in the afternoon. West wind around 12 mph, with gusts as high as 18 mph.nn---nnTonight:nTemperature: 42..FnWind: 5 to 10 mph WSWnForecast: Partly cloudy, with a low around 42. West southwest wind 5 to 10 mph, with gusts as high as 18 mph.nn---nnWednesday:nTemperature: 71..FnWind: 5 to 15 mph WnForecast: Mostly sunny, with a high near 71. West wind 5 to 15 mph, with gusts as high as 24 mph.nn---nnWednesday Night:nTemperature: 40..FnWind: 2 to 14 mph WNWnForecast: Mostly clear, with a low around 40. West northwest wind 2 to 14 mph, with gusts as high as 29 mph.nn---nnThursday:nTemperature: 68..FnWind: 2 to 8 mph ESEnForecast: Sunny, with a high near 68. East southeast wind 2 to 8 mph, with gusts as high as 16 mph.n"}],"isError":false}}
Since the specification change to Streamable HTTP is very recent and not fully implemented as of the writing of this blog, I will forgo doing a granular explanation of that connection sequence. I recommended that you read about the proposed Streamable HTTP implementation here: https://modelcontextprotocol.io/specification/2025-03-26/basic/transports#streamable-http.
Discovery
When an agent needs to interact with a tool or service, MCP provides a resource discovery mechanism that lets MCP clients discover available resources. The MCP client can use direct resources or resource templates. You can read more about the resource discovery options at https://modelcontextprotocol.io/docs/concepts/resources. But, the important thing to know is that the goal of resource discovery is to find out the following information:
- Supported capabilities and actions
- Protocol versions
- Custom metadata
Figure 5 shows the MCP-C to MCP-S request/response flow for the capabilities discovery.
Figure 5. MCP Discovery Flow
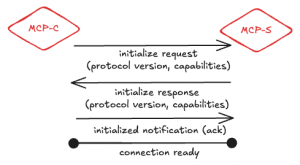
While there is no MCP server registry that MCP clients can search to dynamically discover all available MCP servers and their capabilities, there are MCP server directories as was noted early in the doc. There is an ever-growing number of MCP directories and in many cases, they all have the same or similar list of MCP servers. A few of the many sites include:
MCP Resource Discovery – Example
Let’s look at an example of resource discovery using direct resources.
I have the SQLite MCP Server running on my local machine. I am using Claude Desktop as my AI application with the MCP client functionality configured to use the SQLite MCP server. Here is a snippet from my claude_desktop_config.json file:
"mcpServers": { "sqlite": { "command": "uvx", "args": ["mcp-server-sqlite", "--db-path", "/Users/shmcfarl/code/mcp-testing/sqlite/test.db"] },
When I use Claude Desktop to tool call SQLite and ask for a list of server resources, you can see the message exchange from the MCP client to MCP server.
2025-04-09T18:08:37.964Z [sqlite] [info] Message from client: {"method":"resources/list","params":{},"jsonrpc":"2.0","id":44} 2025-04-09T18:08:37.965Z [sqlite] [info] Message from server: {"jsonrpc":"2.0","id":44,"result":{"resources":[{"uri":"memo://insights","name":"Business Insights Memo","description":"A living document of discovered business insights","mimeType":"text/plain"}]}}
Per the MCP specification you can see the method used by the MCP client is resources/list
and the MCP server responds using the direct resources format:
{ uri: string; // Unique identifier for the resource name: string; // Human-readable name description?: string; // Optional description mimeType?: string; // Optional MIME type }
Conclusion
MCP is off to a strong start, especially for DevOps teams experimenting with AI-driven automation.
At the same time, it’s still a young protocol. MCP gives you a clean foundation if you’re building AI-enabled workflows that need to interact with infrastructure and tools safely—but you’ll still need to assess fit for your specific use case.
There is a lot more introductory content that I could cover, but I think this lays a foundation for the rest of the blog series. For the remainder of the blogs it is important for you to know:
MCP is ideal for:
- Agents need to connect to multiple data sources and services in a standard way
- It abstracts away the per-integration code complexity – just use the MCP SDK
- You need it for a low toil platform or with IDE integrations
What doesn’t MCP do (at least today)?
- MCP is not an agent-to-agent framework
- MCP is not used for the creation, deployment, lifecycle management, and security of agents or tools
- MCP is not an LLM
- MCP is not a data source
- MCP does not dynamically discover tools and services the MCP server will represent
We also learned how MCP clients and servers interact with one another and over which types of protocol and messaging formats.
Let’s stop there and pick back up in the next blog on MCP for DevOps: Use Cases
Prefer to see it in action? Watch the full MCP for DevOps: Architecture & Components video walkthrough here: https://youtu.be/Qdms0EHwhOw
Next in the series
MCP for DevOps: Use Cases
✅ AI Agents Triggering DevOps Tools Use MCP to interact with existing DevOps scripts, APIs, or services in a standard format an AI agent can consume.
✅ Infrastructure-Aware LLMs Let your AI apps ask structured questions like “What kubernetes services are running in namespace default?” or “Create a new database table”—with live answers from systems via MCP servers.
✅ Secure Tool Invocation via AI expose select CLI tools or automation workflows through an MCP server interface, allowing AI agents to interact with them under controlled conditions such as using a Docker scout MCP to scan images.
See you at the next post!
Share: