- How to preorder the Samsung Galaxy S25 series - and the best deals I found
- 4 useful Galaxy S25 Ultra features that creatives and power users will love
- Expanding the Foundation of AI-Native SOCs: Mastering Holistic Data Integration
- This plug-and-play projector made my movie nights cinematic (and it's on sale)
- Samsung likely won't unveil a Galaxy Ring 2 today - but you can look forward to this instead
Strategies to combat GenAI implementation risks

CIOs are under pressure to integrate generative AI into business operations and products, often driven by the demand to meet business and board expectations swiftly. But many IT departments might not thoroughly consider the prerequisites for successful GenAI deployment, and moving quickly can lead to costly errors and implementation failures. We examine the risks of rapid GenAI implementation and explain how to manage it.
As organizations worldwide prepare to spend over $40 billion in core IT (technology budgeted and overseen by central IT) on GenAI in 2024 (per IDC’s Worldwide Core IT Spending for GenAI Forecast, 2023-2027, January 2024), there’s an urgent need to manage the risks associated with these investments. This massive expenditure reflects the rapid adoption of GenAI technologies across various industries, but it also raises significant concerns about the preparedness of IT departments and the greater business to handle the complex requirements for successful implementation.
Notable GenAI blunders
Recent incidents highlight the potential pitfalls of hasty GenAI adoption:
- ChatGPT falsely accused a law professor of harassment.
- Air Canada was ordered to compensate a customer misled by its chatbot.
- Google had to pause its Gemini AI model due to inaccuracies in historical images.
- Samsung employees leaked proprietary data to ChatGPT.
- A ChatGPT bug exposed user conversations to other clients.
These examples underscore the severe risks of data spills, brand damage, and legal issues that arise from the “move fast and break things” mentality. Mark Zuckerberg’s famous quote should serve as a cautionary tale rather than a directive.
Key risks in GenAI implementation
Organizations face a myriad of risks when deploying GenAI, including but not limited to:
- AI and data poisoning
- Bias and limited explainability
- Brand threat
- Copyright infringement
- Cost overruns
- Environmental impact
- Governance and security challenges
- Integration and interoperability issues
- Litigation and regulatory compliance
IDC research shows that two-thirds of companies are overspending on their cloud budgets (IDC CIO Quick Poll, December 2022), and similar budget overruns are expected with GenAI. Additionally, issues like bias, confabulation, and performance inaccuracies are prevalent. The decentralization of GenAI implementation by business staff without IT oversight further complicates governance.
Vendor understanding and preparedness
A significant portion of CEOs (45%) and CIOs (66%) believe that technology vendors don’t fully grasp the risks associated with AI (IDC Worldwide CEO Survey and IDC CIO Quick Poll, January 2024). This skepticism necessitates rigorous questioning of vendors about privacy, data protection, security, and the use of training data. Effective partnering requires transparency and clear documentation from vendors.
GenAI is increasingly being integrated into existing enterprise applications. When considering GenAI implementation risk, organizations can’t forget that “implementation” can be their vendors adding GenAI functionality to products the organization already owns. About 35% of applications currently use some form of AI/ML with that number projected to grow to 50% within two years (Cloud Pulse 2Q23, IDC, August 2023 IDC).
This is a risk that many organizations don’t consider. For instance, Microsoft is embedding its Copilot into Windows 11, and companies like ServiceNow and Box are incorporating GenAI capabilities. It’s crucial for organizations to understand how these integrations work, their implications on data privacy, and the conditions under which they operate.
Organizations should prepare a comprehensive checklist of questions to evaluate these integrations and discuss the implications with their vendors. Organizations need to determine whether to allow or upgrade to the functionality depending on the privacy, security, accuracy, and transparency implications of the embedded implementation.
Five components of GenAI implementation
In an effort to mitigate GenAI implementation risk, consider the following five elements:
- Technology: systems, services, networking, and platforms underpinning GenAI (could be cloud, on-premises, or hybrid)
- Processes: bias mitigation, security, model transparency, data ingestion, and privacy
- Talent: technical, data, and model skills to be obtained through training, hiring, or partnering
- Governance: oversight, accountability, legal, statutory, and ethical expertise.Multi-disciplinary
- Data: good, known, understood data relevant to the use case
IDC, 2024
Assessing AI maturity
Understanding AI maturity is crucial for successful GenAI implementation. Organizations must realistically assess their AI maturity levels relative to the project requirements to avoid overcommitting resources. This maturity can be built, procured, or acquired through partnerships, with knowledge transfer being a critical component of any external collaboration. The key elements to understand when assessing maturity are technology, process, talent governance, and data – not surprisingly, the five elements in the checklist above.
The graphic below describes AI maturity levels as defined by IDC’s MaturityScape model.
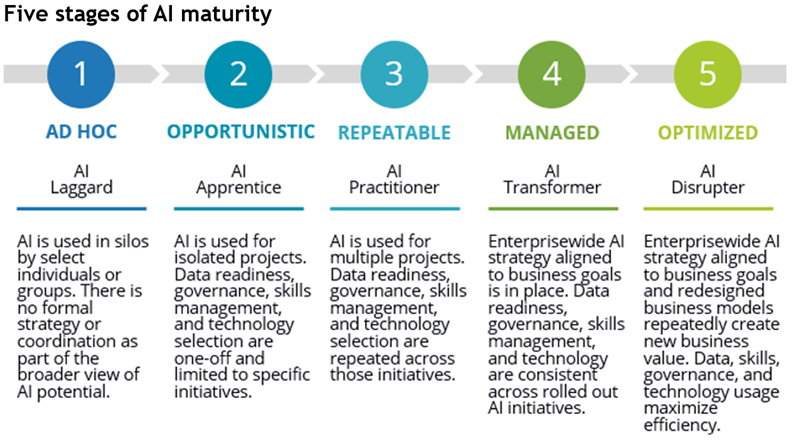
IDC MaturityScape: Artificial Intelligence 2.0, May 2022
When examining use cases, choose ones that match your AI maturity (or the maturity you can procure with third parties). Don’t get over your skis and attempt a use case that your organization is not aligned to support.
Use case categories and their requirements
GenAI use cases fall into three main categories:
- Productivity or efficiency: These are low-risk tasks like summarizing reports or creating RFPs, requiring minimal customization and talent. They correlate to a low AI maturity and typically bring limited benefits.
- Functional: These are medium-risk tasks like hyper-personalized marketing, necessitating good data and available in-house talent. They correlate to a moderate AI maturity and can bring moderate benefits.
- Industry or transformational: These are high-risk, high-impact use cases like generative drug discovery, requiring significant investment in talent and data quality. These can be competitive differentiators or create a competitive moat. They correlate to a high AI maturity and can bring big benefits.
Build versus buy: A balanced approach
Organizations should adopt a mix of build-and-buy strategies tailored to their specific business and technology contexts. This balanced approach ensures that projects align with organizational maturity and value requirements. The figure below illustrates how the use cases map onto maturity and value and then the related implementation options.
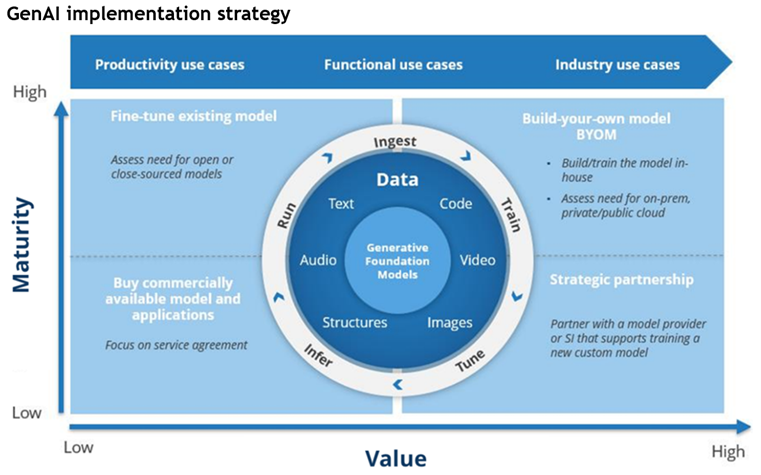
Generative AI: Approaches for Competitive Advantage, IDC, October 2023
Conclusion
Navigating the risks of GenAI requires a comprehensive understanding of an organization’s AI maturity, a balanced build-and-buy approach, and rigorous vendor evaluation. By addressing these challenges and leveraging the right infrastructure, organizations can harness the transformative potential of GenAI while minimizing risks.
International Data Corporation (IDC) is the premier global provider of market intelligence, advisory services, and events for the technology markets. IDC is a wholly owned subsidiary of International Data Group (IDG Inc.), the world’s leading tech media, data, and marketing services company. Recently voted Analyst Firm of the Year for the third consecutive time, IDC’s Technology Leader Solutions provide you with expert guidance backed by our industry-leading research and advisory services, robust leadership and development programs, and best-in-class benchmarking and sourcing intelligence data from the industry’s most experienced advisors. Contact us today to learn more.
Get more advice from IDC on managing the risks of GenAI and build versus buy for GenAI.
Daniel Saroff is group vice president of consulting and research at IDC, where he is a senior practitioner in the end-user consulting practice. This practice provides support to boards, business leaders, and technology executives in their efforts to architect, benchmark, and optimize their organization’s information technology. IDC’s end-user consulting practice utilizes our extensive international IT data library, robust research base, and tailored consulting solutions to deliver unique business value through IT acceleration, performance management, cost optimization, and contextualized benchmarking capabilities.
Daniel also leads the CIO/end-user research practice at IDC. This practice provides guidance to business and technology executives on how to leverage technology to achieve innovative and disruptive business outcomes. IDC’s research enables clients to create grounded business and technology strategies and empowers IT leaders to deliver services and innovation that drive business growth and success.
Daniel has over 20 years of experience in senior-level positions in both consulting organizations and delivering technology. Prior to joining IDC, he served as the CIO for a major Massachusetts state agency, where he led the agency through cloud migrations, digital transformation, technology modernization, enterprise platform reengineering, security in-depth and zero trust, and defense and response to a major cyberattack. He also held consulting positions at both Forrester and Gartner and was an IT director at a major aerospace and defense company. Before joining IDC, he owned a private IT management consultancy.