- These are the Memorial Day headphones deals I recommend most to family and friends
- 4 gadgets I'm bringing to the beach this summer - and why they make such a big difference
- I'm a laptop expert and these are the Memorial Day laptop deals I'd scoop up ASAP
- These are my 13 favorite Memorial Day lawn and outdoor deals right now
- The most comfortable noise-canceling earbuds I've tested are on sale (and they're only $150)
The Challenge of Combatting Threats Against Autonomous Vehicles
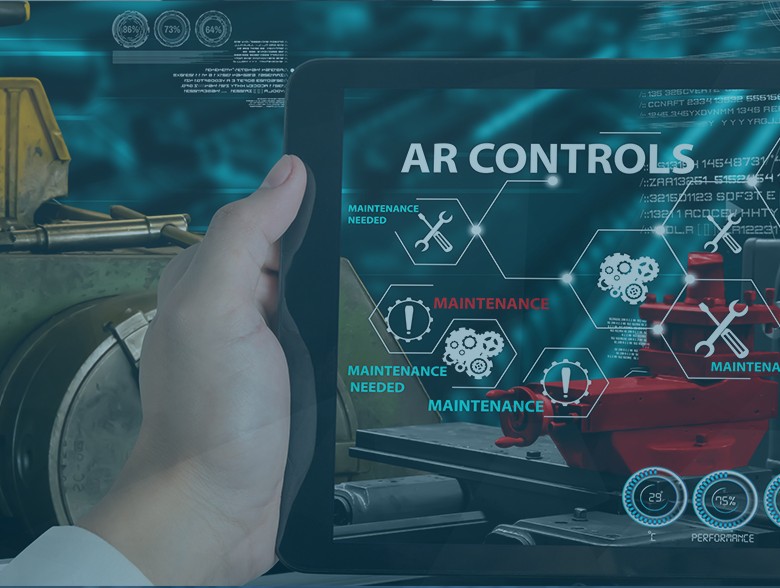
By Joseph Hladik, Cyber Group Lead, Neya Systems
From perception and sensing to mapping and localization, both off-road and on-road autonomous vehicles rely heavily on software and connectivity to operate safely. Unfortunately, as with any connected device, autonomous vehicles can be vulnerable to cyberattacks. As the technology continues to evolve, protecting a vehicle’s software and communication systems from cyber threats is critical to ensuring the safety and integrity of autonomous transportation, whether on a paved road, a dense forest, or a construction site.
A threat actor that compromises a vehicle’s software or communications system and achieves unauthorized access to a vehicle can potentially result in data theft, remote command and control operations, collision, injury, or even loss of life. This is especially true of military applications, where ensuring the cybersecurity of autonomous off-road vehicles is paramount to protect not only the safety of passengers, but also the integrity of the mission.
As it stands, however, cybersecurity for autonomous vehicles is still immature when compared to the protection capabilities of an enterprise environment. The complexity of autonomous systems, combined with a lack of universal standards, makes ensuring the security of these vehicles a challenging task. As such, the potential for adversarial threats requires advanced security measures and constant vigilance.
A Zero Trust Approach
At Neya, we are taking a Zero Trust approach with autonomous vehicles to secure network communications, monitor endpoints, implement identity and access management, and implement cryptographic key management, to name a few examples.
Zero Trust is built upon the philosophy of “trust nothing, verify everything.” Historically, cybersecurity protections have followed Defense-In-Depth, or Layered Security approaches which are based upon physical security concepts of building a bastion. With these security models, you have a trusted internal network and untrusted external network divided by a multi-layered perimeter. Threats are typically identified when these protection layers are penetrated. Instead, Zero Trust assumes that threats may be present both inside and outside the network perimeter.
Another way to look at this is that traditional security implementation models are system-centric, whereas Zero Trust is data-centric. This is a key component of understanding the importance of Zero Trust, since it is concerned with knowing: 1) what data you have; 2) where it is moving within and outside of your network; and 3) who is accessing it.
The threat landscape is always evolving; therefore, the attack surface is much wider than it ever has been. There is a constant escalation between threat actors and those defending against them. As security breaches are detected and investigated, leading to improved defensive measures, offensive actors are forced to develop novel techniques to circumvent enhanced protections. Additionally, IT and OT environments have grown more complex in the last decade. Most environments are now hybrid on-premises with cloud-based components, or multi-cloud. Add in the growing diversity of physical devices such as mobile phones, tablets, wearables, and other IoT devices, which are leading traditional security models to age out of relevancy to effectively protect data and the end-user. A point of vulnerability only recently considered is with autonomous systems, or in this case, autonomous vehicle systems.
With the growing complexity of environments and diversity of devices accessing networks in mind, Zero Trust aims to defend against these many modern use cases. Above all, you need to protect sensitive data. Zero Trust provides the framework to secure access to resources, regardless of the user or device’s location with consistent and enforceable security policies. Implementing least-privilege access policies and encryption mechanisms, followed by continuous verification of both user and device identities, allows organizations to prevent unauthorized access to critical data assets. The goal is to reduce the attack surface available to threat actors. One critical piece of Zero Trust is focused on continuous monitoring and behavioral analysis to detect anomalies and suspicious activities in real-time.
Real-Time Threat Identification
To be effective, cyber autonomy must be able to intelligently identify risks and take action to mitigate potential threats to autonomous vehicle missions. It must be completely self-contained, capable of autonomously detecting, reporting, and defending against threats that can exploit or disrupt a mission. By implementing a suite of behavioral analytics to baseline an expected, normal state-of-vehicle operation, we can leverage anomaly-detection techniques that will function as the “intelligent” subsystem, which then inform the decision-making capability of a cyber autonomous system.
The lifecycle of an autonomous vehicle is in three stages: Pre-Mission, Mission Operation, and Post-Mission. As one might expect, pre-mission routines are focused on defining the assets and mission objective. The vehicle will need to pass a pre-mission checklist for it to be authorized for operation. From a cybersecurity perspective, we need to ensure that each boot-up process is from an expected state to mitigate the potential for unauthorized software or malware usage.
Another example is to ensure that all OS and software is patched and up to date before the mission begins. Post-mission routines are focused primarily on reporting notable events and maintenance. All cybersecurity anomalies, events, and responses will be reported via an analytics dashboard within Neya’s Mission Planning and Management System (MPMS). Additionally, bulk forensic data is captured and made available for authorized personnel. Securely updating the OS and software is also a crucial step in the post-mission routine, as the time to perform this task may cause significant delays when performed during the pre-mission routine.
Mission operation is the most complex stage of the autonomous vehicle lifecycle. Consider how an autonomous vehicle operates using perception and sensing to determine a path of least resistance. The perception and planning systems work to identify anomalous objects that serve as obstacles that cause the vehicle to change direction or operation for optimal traversal. Conceptually, cyber anomaly detection is remarkably similar. Sensors are placed within the vehicle network and its endpoints to detect digital signals of anomalous activity, instead of detecting a physical anomaly using RADAR or LiDAR as sensors.
Relevant data is an absolute necessity for anomaly detection to be successful. It needs to be structured, categorized, and labeled for effective aggregation and consistency to ensure data integrity for the subsystem responsible for processing it. An analytic baseline to determine what is normal or expected is required once the data is understood and organized. A simple example is measuring the network flow volume (i.e., could be bytes, packets, transactions) between two nodes within a vehicle. An anomaly will be reported if the network flow volume increases or decreases an order of magnitude away from what is recorded as normal in the established baseline for that analytic. After extensive testing to determine specific analytics to measure and to improve baseline accuracy, it is ready to begin field operations. The confidence threshold for positively identifying a cyber threat should also improve as more data and telemetry is collected and processed with each mission.
Furthermore, just as the vehicle autonomy system determines a response to physical anomalies, the autonomous system also will need to respond accordingly to a perceived cyber threat. For example, a threat actor attempts to establish unauthorized command and control of an autonomous vehicle using a rogue Operator Control Unit (OCU). There are several methods of protection, including defensive hardening to mitigate this attack vector, but in the case of a breach of defense, a standard operating procedure needs to exist to respond to this detected threat. In enterprise environments, this is handled by a person or group of people. An autonomous vehicle, on the other hand, is not expected to have trained personnel to respond.
Evaluate and Enforce
At Neya, we are introducing the Cyber Autonomous Response and Recovery System (CARRS) to autonomous vehicle platforms to solve this problem. CARRS is a kit that will be attached to the vehicle’s autonomy stack. Its role is to evaluate and enforce Zero Trust policies and actively respond to detected high fidelity threats. CARRS will dynamically issue vehicle profile or configuration changes during a mission in the case of a security policy violation or detected threat. It may determine that turning off the radios is an appropriate response or push a change in network policy to deny traffic from a rogue OCU device, as previously noted in the above example.
The capabilities of autonomous vehicles are ever evolving, and, at the same time, so is the threat landscape. Adversaries are ever vigilant and novel in their approach to cyber-attacks. The complexity of autonomous vehicles, both on-road and off-road, underscores the need for continued vigilance in addressing potential vulnerabilities.
About the Author
Joseph Hladik is the Cyber Group Lead at Neya Systems and has been with Neya since 2023. During this time, Hladik has led various projects, including cyber autonomy, a solution-based software program that will be added to mission planning to mitigate cyber threats during deployment. Prior to Neya, Hladik worked as the Director of Threat Research and Intelligence at Counterflow AI, where he furthered the knowledge and detection of threat actor Tactics, Techniques, and Procedures (TTP) leveraging Machine Learning Algorithms (MLA) and Behavioral Analytics. Before his role at Counterflow AI, Hladik worked at Mandiant as the Regional Manager for the U.S. Northeast and Canada regions.
Hladik can be reached through the Neya company website www.neyarobotics.com