- Want to upgrade your home's tech? First, assess your energy maturity - here's how
- Can you build a billion-dollar business with only AI agents (yet)? This author thinks so
- I replaced my OnePlus with this $700 Motorola flip phone, and it's spoiled me big time
- Your TV's USB port has an underrated benefit that can revitalize an old system - here's how it works
- I finally tried Samsung's XR headset, and it beats my Apple Vision Pro in meaningful ways
The Illusion of Truth: The Risks and Responses to Deepfake Technology
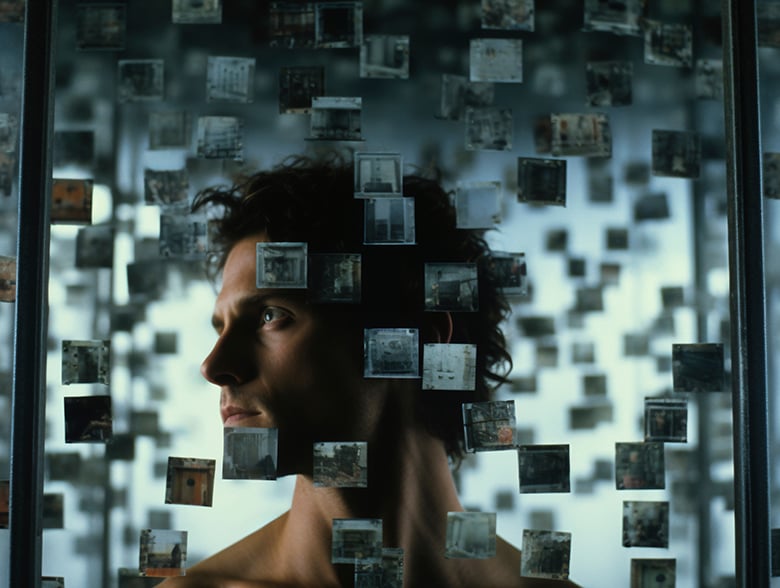
Abstract
In the age of information, where the line between reality and fiction is increasingly blurred, deepfake technology has emerged as a powerful tool with both immense potential and significant risks. Deepfake technology, utilizing sophisticated artificial intelligence (AI) and machine learning techniques to generate hyper-realistic audio and video, poses significant security threats alongside its innovative applications. This article provides an in-depth exploration of deepfake technology, illustrates its potential for misuse in various domains including misinformation and identity fraud, and proposes a comprehensive framework for mitigating these risks through technological, educational, and legislative measures.
How Deepfake Works
Deepfake technology relies on a complex process involving artificial neural networks. These networks are trained on vast amounts of data, such as images and videos, to learn patterns and recognize features. Once trained, the network can generate highly realistic contents, often indistinguishable from the original. To understand the technical foundations of deepfake technology, one must look at the fields of machine learning and artificial intelligence. At the core of this technology are Generative Adversarial Networks (GANs) and Deep Learning.
Generative Adversarial Networks (GANs)
GANs consist of two neural networks—the generator and the discriminator—engaged in a continuous loop of competition. The generator creates images or sounds that mimic the real data, while the discriminator evaluates their authenticity. Over time, the generator learns from the discriminator’s feedback, improving its outputs until they are indistinguishable from authentic data.
Deep Learning:
Deep learning has been pivotal in the advancement of deepfake, with convolutional neural networks (CNNs) being extensively used to analyze and replicate the minute details of human expressions and voices. These models are trained on extensive datasets containing millions of images and audio files, which they use to learn and replicate human features with startling accuracy.
The Dark Side of Deepfake
The increasing accessibility of deepfake technology due to advancements in AI presents both significant opportunities and considerable risks. On one hand, it facilitates creative content generation, enhances artistic expression and improves educational experiences. On the other hand, it poses serious threats, including fraud, the proliferation of misinformation, and social manipulation. Here are some of the most concerning applications of deepfake:
- Disinformation and Propaganda: Deepfake can be used to create false information that can alter public opinion, influence elections, and incite violence. For example, a deepfake video of a politician making controversial statements could damage their reputation and undermine their credibility.
- Personal and Corporate Fraud: Deepfake can bypass facial recognition software or imitate voices in voice-activated systems, which can compromise safe access or personal banking systems. Corporations face threats of espionage with deepfake used in phishing attacks to obtain sensitive information or manipulate stock prices through fabricated announcements from influential figures.
- Harassment and Cyberbullying: When people are portrayed in deepfake videos without their consent, it may lead to psychological distress, social rejection, and legal issues.
- National Security Threats: Deepfake has the potential to destabilize countries, foster international conflict, and produce misleading intelligence.
Mitigating the Risks of Deepfake
Combating the misuse of deepfake technology involves a multi-faceted approach that integrates technological solutions, legal frameworks, public awareness initiatives, and international cooperation. Various methods have been developed, each addressing different aspects of the deepfake detection challenge. Here are some of the key measures that can be applied to mitigate the risks associated with deepfake technology:
- Technological Detection Techniques:
- Digital Forensic Techniques: These involve analyzing the digital fingerprints left behind by deepfake algorithms. By examining pixel-level characteristics, inconsistencies like unnatural blinking or distorted backgrounds can be detected.
- AI-Driven Detection: AI-powered tools can analyze videos frame by frame, identifying inconsistencies in lighting, shadows, and facial expressions that may indicate manipulation.
- Blockchain for Verification: To ensure the authenticity of digital content, blockchain technology can be used to create an immutable ledger of media files. Several companies utilized blockchain to verify the integrity of images and videos at the point of capture, making unauthorized alterations easily detectable.
- Educational Initiatives and Public Awareness
Increasing public awareness and education is pivotal for the early detection and resistance against misinformation spread by deepfake:
- Media Literacy Programs: Programs like MediaWise, a project funded by Google and run by the Poynter Institute, aim to educate young people and the general public on how to identify fake news, including content manipulated by deepfake technologies. They use real examples from recent elections where deepfake videos were employed to create confusion and spread misinformation.
- Workshops and Training: The prominent media outlets have organized workshops that teach journalists and content creators how to spot deepfake. These sessions often use real-life examples, such as manipulated speeches of political figures, to train attendees on the telltale signs of fabricated content.
- Policy and Regulation
Legislative action can also play a significant role in controlling the spread and impact of deepfake:
- Legal Frameworks: The European Union’s GDPR has been adapted to include rights against unauthorized use of biometric data, which can be extended to govern the use of personal images and videos in deepfake. Similarly, in the United States, the DEEPFAKES Accountability Act was introduced in Congress to criminalize the malicious creation and distribution of deepfake content.
- Corporate Policies: Social media platforms like Facebook and Twitter have implemented specific policies to handle deepfake content. For example, Twitter’s approach involves labeling tweets that contain synthetic media, whereas Facebook collaborates with third-party fact-checkers to identify and reduce the circulation of deepfake.
- Industry and Academic Partnerships
Developing and deploying technology solutions in partnership with various stakeholders is essential for a robust defense against deepfake:
- Industry Collaboration: In response to the deepfake threat, major technology firms such as Microsoft have developed tools like Microsoft’s Video Authenticator, which analyzes a video’s content and gives a score indicating the likelihood it’s been artificially manipulated.
- Academic and Industry Research: Universities and tech companies are collaborating on new research initiatives to stay ahead of deepfake technology. For instance, partnerships like the Deepfake Detection Challenge (DFDC) launched by Facebook aim to spur the development of deepfake detection tools through global competitions.
- International Cooperation:
- Global Frameworks: Promote international collaboration to develop unified legal standards and cooperative measures to prevent the global spread of malicious deepfakes. This includes sharing technologies, strategies, and intelligence across borders.
- Cross-Border Enforcement: Work towards agreements for cross-border enforcement of laws against the creation and distribution of harmful deepfake content, recognizing that digital media transcends national boundaries.
Conclusion
Deepfake technology is a double-edged sword. While it presents significant risks, it also offers potential benefits. To harness the positive aspects of this technology while mitigating its negative impacts, a multi-faceted approach is necessary. This includes developing robust detection tools, educating the public about deepfake, and establishing strong legal frameworks to regulate their use. By working together, governments, technology companies, and individuals can ensure that deepfake technology is used responsibly and ethically, ultimately benefiting society as a whole.
As deepfake technology continues to evolve, it is imperative to remain vigilant, adapt to emerging threats, and promote the ethical and responsible use of this powerful tool.
Endnotes:
- Chesney, R., & Citron, D. (2019). Deepfakes and the New Disinformation War: The Coming Age of Post-Truth Geopolitics. Foreign Affairs. https://www.foreignaffairs.com/
- Goodfellow, I., Pouget-Abadie, J., Mirza, M., Xu, B., Warde-Farley, D., Ozair, S., … & Bengio, Y. (2014). Generative Adversarial Nets. Advances in Neural Information Processing Systems.
- Paris, B., & Donovan, J. (2019). Deepfakes and Cheapfakes: The Manipulation of Audio and Visual Evidence. Data & Society Research Institute. https://datasociety.net/
- Verdoliva, L. (2020). Media Forensics and Deepfakes: An Overview. IEEE Journal of Selected Topics in Signal Processing, 14(5), 982–992. DOI: 10.1109/JSTSP.2020.3002101
- Microsoft. (2020). Video Authenticator Tool to Combat Disinformation. Microsoft AI Blog. https://blogs.microsoft.com/
- Deepfake Accountability Act. (2019). U.S. Congress. https://www.congress.gov/
- Sample, I. (2019). What Are Deepfakes – And How Can You Spot Them? The Guardian. https://www.theguardian.com/
- Facebook AI. (2020). Deepfake Detection Challenge. https://ai.facebook.com/
- Vincent, J. (2020). Deepfake Detection Algorithms Will Never Be Enough. The Verge. https://www.theverge.com/
- General Data Protection Regulation (GDPR). European Union. https://gdpr-info.eu/
- Nguyen, T. T., Nguyen, C. M., Nguyen, D. T., Nguyen, D. T., & Nahavandi, S. (2019). Deep Learning for Deepfakes Creation and Detection: A Survey. ArXiv Preprint. https://arxiv.org/abs/1909.11573
- Kietzmann, J., Paschen, J., & Treen, E. R. (2020). Artificial Intelligence in Content Marketing: A Synthesis and Research Agenda. Journal of Business Research, 116, 273–285. DOI: 10.1016/j.jbusres.2020.05.001
- Maras, M.-H., & Alexandrou, A. (2019). Determining Authenticity in the Age of Post-Truth Politics. International Journal of Information Management, 48, 43–50. DOI: 10.1016/j.ijinfomgt.2019.01.017
- Rössler, A., Cozzolino, D., Verdoliva, L., Riess, C., Thies, J., & Nießner, M. (2019). FaceForensics++: Learning to Detect Manipulated Facial Images. Proceedings of the IEEE/CVF International Conference on Computer Vision (ICCV). https://openaccess.thecvf.com/
- Pogue, D. (2019). Deepfakes Are Getting Scary Good. How Do We Tell What’s Real? Scientific American. https://www.scientificamerican.com/
- Schick, N. (2020). Deepfake Videos: How to Protect Yourself and Fight Back. Consumer Reports. https://www.consumerreports.org/
- Citron, D. K. (2019). Sexual Privacy. Yale Law Journal, 128, 1870–1960. https://www.yalelawjournal.org/
- Floridi, L. (2020). AI and Deepfakes: The End of Trust? Philosophy & Technology, 33(3), 385–389. DOI: 10.1007/s13347-020-00417-7
About the Author
Rohit Nirantar | CISM, PMP, Azure Security Engineer Associate, DevOps Engineer Expert
Rohit Nirantar is a Project Manager at Deloitte with over 18 years of experience in IT, specializing in application security, cybersecurity, and cloud security. He has successfully managed and implemented security solutions for global organizations, leveraging his expertise in secure cloud practices, threat management, and compliance frameworks. Rohit holds a diverse range of certifications in Information Security, Project Management, and Cloud Security. He is passionate about promoting cybersecurity awareness and fostering collaboration within professional communities. Rohit can be reached online at [email protected].