- Customer Experience is Ready to Engage at Cisco Live
- Arc reinvented browsing for the better - and that was apparently the problem
- Why I prefer this rugged Samsung phone over flagship models (and it looks just as good)
- Can a home wind turbine replace my solar panels? My results after months of testing
- DragonForce Ransomware Leveraged in MSP Attack Using RMM Tool
Three Key Facts About AI-Driven Network Detection and Response
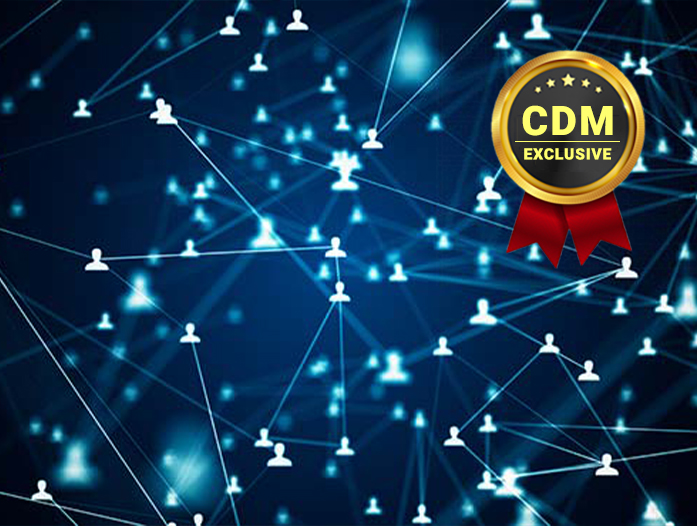
By Eyal Elyashiv, CEO, Cynamics
Most network detection and response solutions and network performance monitoring and diagnostic tools are using the same paradigm that was invented three decades ago. However, networks themselves have changed dramatically; modern networks grow more complex and interconnected every day, and these new connections increase potential for vulnerabilities. Malicious actors are constantly hunting for ways to infiltrate corporate networks, and overly complex, linked systems allow them to slip through the security gaps unnoticed. For years enterprises have been attempting to address this security challenge but have failed to gain the upper hand.
The primary reason for this failure is two-fold: human analysts can’t keep up in this environment and legacy tools can’t either. Enterprises need assistance from AI-based solutions to enable full visibility into their network. Network detection and response (NDR) solutions derive particular benefit from AI. However, to implement NDR well, organizations need clarity on its key elements, both before and after implementation.
AI helps fill in the security skills gaps
As networks become more complex and data volumes continue to grow, the fact is human analysts are incapable of monitoring all of it, alone. To make matters worse, the industry is experiencing an estimated shortage of 2.72 million skilled cybersecurity professionals – there just aren’t enough skilled people to adequately defend organizations’ critical assets. Instead, the industry must learn how to use tools like AI and ML to supplement these skills gaps. The lack of capable and experienced cybersecurity talent can leave networks vulnerable to a myriad of threats.
How AI addresses the network visibility problem
Meanwhile, a seemingly intractable security hurdle arises as “smart networks” increase in scale and complexity. Anomalies, attacks and threats can start with one simple click and begin at one of the hundreds or thousands of devices connected to the network – workstations, routers, switches and more, significantly compromising network security.
It’s both impractical and expensive to add specialized network monitoring and detection solutions to each network device, and it can negatively impact device performance. Monitoring each network component separately is insufficient, detecting a sophisticated attack requires a holistic view of the network and comprehensive analysis of network patterns across devices.
Using AI/ML provides this holistic view. Machine learning techniques extrapolate the most likely behavior of all network traffic based on radically small traffic samples from every network device, including private or public cloud and legacy routers, using standard sampling protocols that are built-in in every network device. Then, the ML automatically learns the most important network fields, using these to summarize the network state in each device at each timestamp. It can also understand changing network trends autonomously.
AI detection models constantly analyze network traffic patterns over time in several layers – including each device by itself, the entire network level and groups of devices, and looks for suspicious behaviors. These models are based on analysis of small samples of network traffic which greatly reduces processing time, compared to current solutions that must collect, process and analyze each and every packet. Such models enable early and faster detection.
Previously unseen traffic patterns can uncover what’s really taking place on networks in real time, without the expense and impracticality of monitoring every device. This makes AI-based NDR solutions time-efficient, cost-effective and holistic in their network coverage.
Predicting threats and anomalies
AI-based NDR can autonomously predict threats and hidden patterns before attacks happen. It automatically monitors the network to detect threats and anomalies for rapid, precise prediction, while you focus on operations. This triggers appropriate policies to block today’s most damaging threats, including ransomware and DDoS attacks, long before they reach your sensitive assets.
A significant benefit of this kind of solution is that it doesn’t require any changes to your network, some are even agnostic to network hardware and architectures. You don’t need to install any appliances or agent, and it’s non-intrusive, which reduces risk.
Integral to network security
Thirty years in the technology realm is like a lifetime due to the rapid pace of change. It’s unrealistic to think that solutions designed three decades ago can protect today’s complex networks against sophisticated attackers. Another massive technology change that has shaken up the industry is the advent of AI- and ML-based security applications, including NDR.
These technologies provide full network visibility across all endpoints, some do so using only a fraction of network traffic. This enables fast and accurate threat detection that immediately identifies network deficiencies and vulnerabilities. These non-intrusive, cost-effective solutions create a comprehensive view of your network and are rapidly becoming an integral part of modern-day network security.
About the Author
Eyal Elyashiv is the CEO and co-founder of Cynamics the only Next Generation (NG) Network Detection and Response (NDR) solution in the market today using standard sampling protocols built-in to every gateway, patented algorithms, and AI and Machine Learning, to provide threat prediction and visibility at speed and scale.
Eyal can be reached online at @cynamics_ai (Twitter) or on LinkedIn at https://www.linkedin.com/company/cynamics/. The company website is: https://www.cynamics.ai/
FAIR USE NOTICE: Under the “fair use” act, another author may make limited use of the original author’s work without asking permission. Pursuant to 17 U.S. Code § 107, certain uses of copyrighted material “for purposes such as criticism, comment, news reporting, teaching (including multiple copies for classroom use), scholarship, or research, is not an infringement of copyright.” As a matter of policy, fair use is based on the belief that the public is entitled to freely use portions of copyrighted materials for purposes of commentary and criticism. The fair use privilege is perhaps the most significant limitation on a copyright owner’s exclusive rights. Cyber Defense Media Group is a news reporting company, reporting cyber news, events, information and much more at no charge at our website Cyber Defense Magazine. All images and reporting are done exclusively under the Fair Use of the US copyright act.